Modified Bald Eagle Search Algorithm With Deep Learning-Driven Sleep Quality Prediction for Healthcare Monitoring Systems
IEEE Access(2023)
摘要
Sleep habits are strongly related to health behaviors, with sleep quality serving as a major health indicator. Current approaches for evaluating sleep quality, namely polysomnography and questionnaires, are often time-consuming, costly, or invasive. Thus, there is a pressing need for a more convenient, nonintrusive, and cost-effective method. The applications of deep learning (DL) in sleep quality prediction represent a groundbreaking technique for addressing sleep-related disorders. In this aspect, the article offers the design of a Modified Bald Eagle Search Algorithm with Deep Learning-Driven Sleep Quality Prediction (MBES-DLSQP) for Healthcare Monitoring Systems. The MBES-DLSQP technique combines the strengths of a DL model with a hyperparameter tuning strategy to provide precise sleep quality predictions. At the primary stage, the MBES-DLSQP technique undergoes data pre-processing. Besides, the MBES-DLSQP technique uses a stacked sparse autoencoder (SSAE)-based prediction model, which can extract and encode high-dimensional sleep data. The MBES-DLSQP incorporates MBESA-based hyperparameter tuning which assures its optimal configurations to further boost the efficiency of the SSAE model. The experimental outcome of the MBES-DLSQP algorithm is tested on the sleep dataset from the Kaggle repository. The experimental value infers that the MBES-DLSQP technique shows promising performance in sleep quality prediction with a maximum accuracy of 98.33%.
更多查看译文
关键词
Sleep,Tuning,Sleep apnea,Monitoring,Predictive models,Prediction algorithms,Medical services,Deep learning,Sleep quality prediction,healthcare monitoring,deep learning,parameter tuning,artificial intelligence
AI 理解论文
溯源树
样例
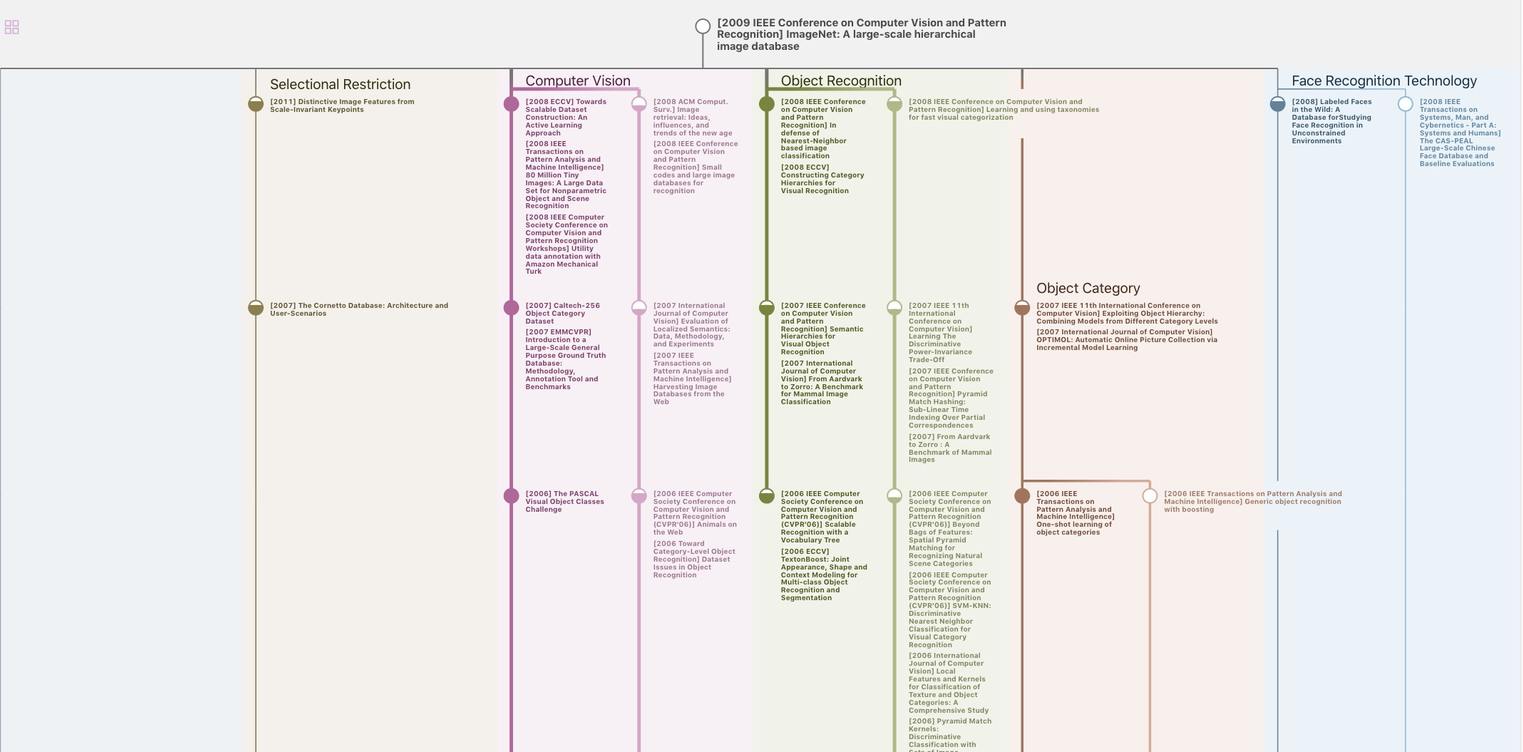
生成溯源树,研究论文发展脉络
Chat Paper
正在生成论文摘要