Predicting the climate impact of healthcare facilities using gradient boosting machines
CLEANER ENVIRONMENTAL SYSTEMS(2024)
摘要
Health care accounts for 9-10% of greenhouse gas (GHG) emissions in the United States. Strategies for monitoring these emissions at the hospital level are needed to decarbonize the sector. However, data collection to estimate emissions is challenging. We explored the potential of gradient boosting machines (GBM) to impute missing data on resource consumption in the 2020 survey of a consortium of 283 hospitals participating in Practice Greenhealth. GBM imputed missing values for selected variables in order to predict electricity use (R2 = 0.82) and beef consumption (R2 = 0.82) and anesthetic gas desflurane use (R2 = 0.51), using administrative and financial data readily available for most hospitals. After imputing missing consumption data, estimated GHG emissions associated with these three examples totaled over 3 million metric tons of CO2 equivalent emissions (MTCO2e). Specifically, electricity consumption had the largest total carbon footprint (2.4 MTCO2e), followed by beef (0.6 million MTCO2e) and desflurane consumption (0.03 million MTCO2e) across the 283 hospitals. The approach should be applicable to other sources of hospital GHGs in order to estimate total emissions of individual hospitals and to refine survey questions to help develop better intervention strategies.
更多查看译文
关键词
Healthcare facility,Climate change,Machine learning,Missing data imputation,Sustainability
AI 理解论文
溯源树
样例
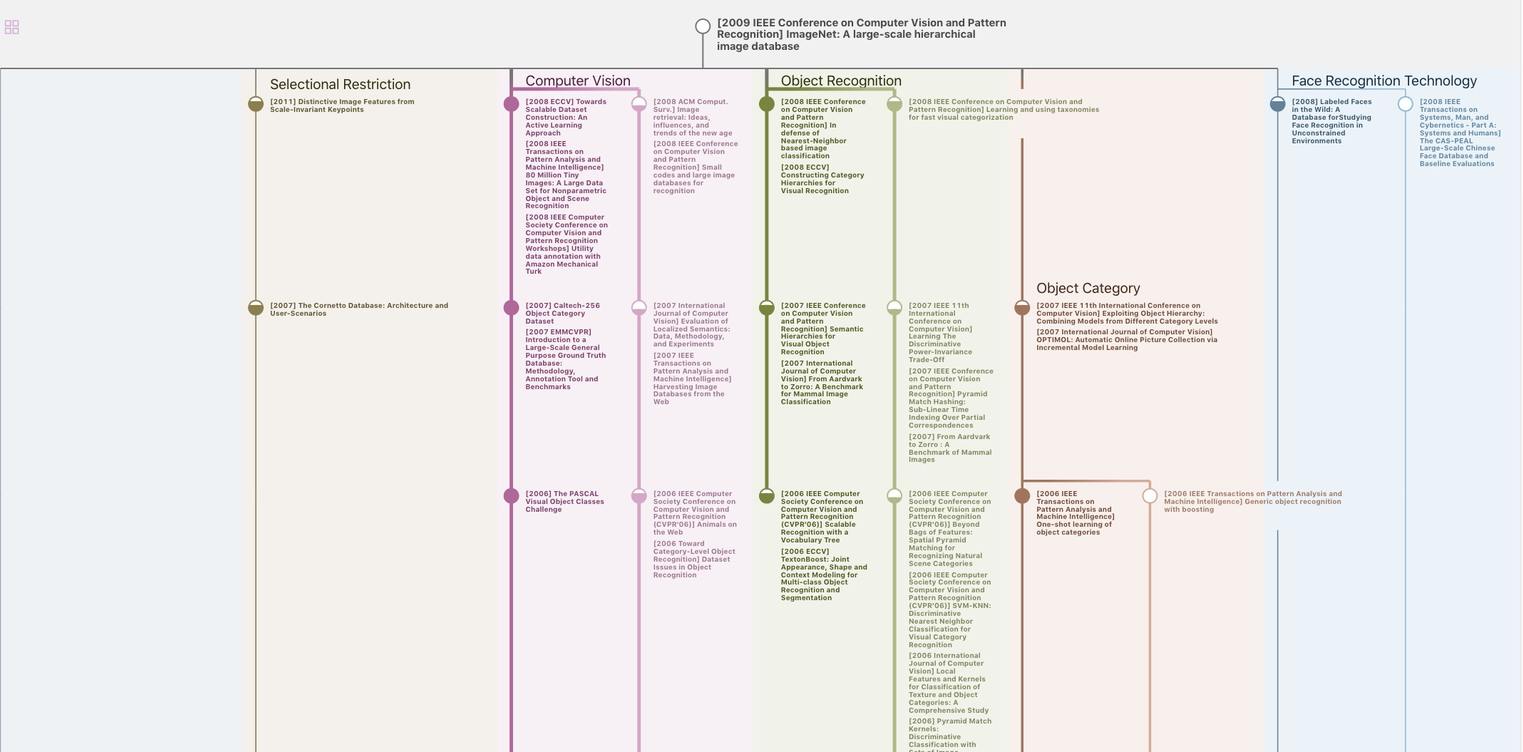
生成溯源树,研究论文发展脉络
Chat Paper
正在生成论文摘要