GAT-FP: Addressing Imperfect Multimodal Learning using Graph Attention Networks and Feature Propagation.
Proceedings of the 12th International Symposium on Information and Communication Technology(2023)
摘要
Multimodal learning tries to increase generalization performance by leveraging information from several data modalities. However, effectively integrating such multi-modality data remains a complex endeavor, particularly when dealing with incomplete data. In real-world scenarios, modalities are often not entirely absent but rather incomplete due to various external or internal factors. For instance, audio data can be corrupted by noise, and text data may suffer from inaccuracies stemming from automatic speech recognition errors. To address these challenges, we propose a novel framework for incomplete multimodal learning in conversational contexts, named GAT-FP. Our GAT-FP model incorporates two key graph neural network-based modules, namely, “Feature Propagation” and “Graph Attention Network”. These modules are designed to estimate missing features and discern the significance of interactions among incomplete feature nodes within the graph structure. To validate the effectiveness of our approach, we conduct extensive experiments on two well-established benchmark multimodal conversational datasets: IEMOCAP and MELD. The experimental results demonstrate that our GAT-FP model surpasses existing state-of-the-art methods in the realm of incomplete multimodal learning.
更多查看译文
AI 理解论文
溯源树
样例
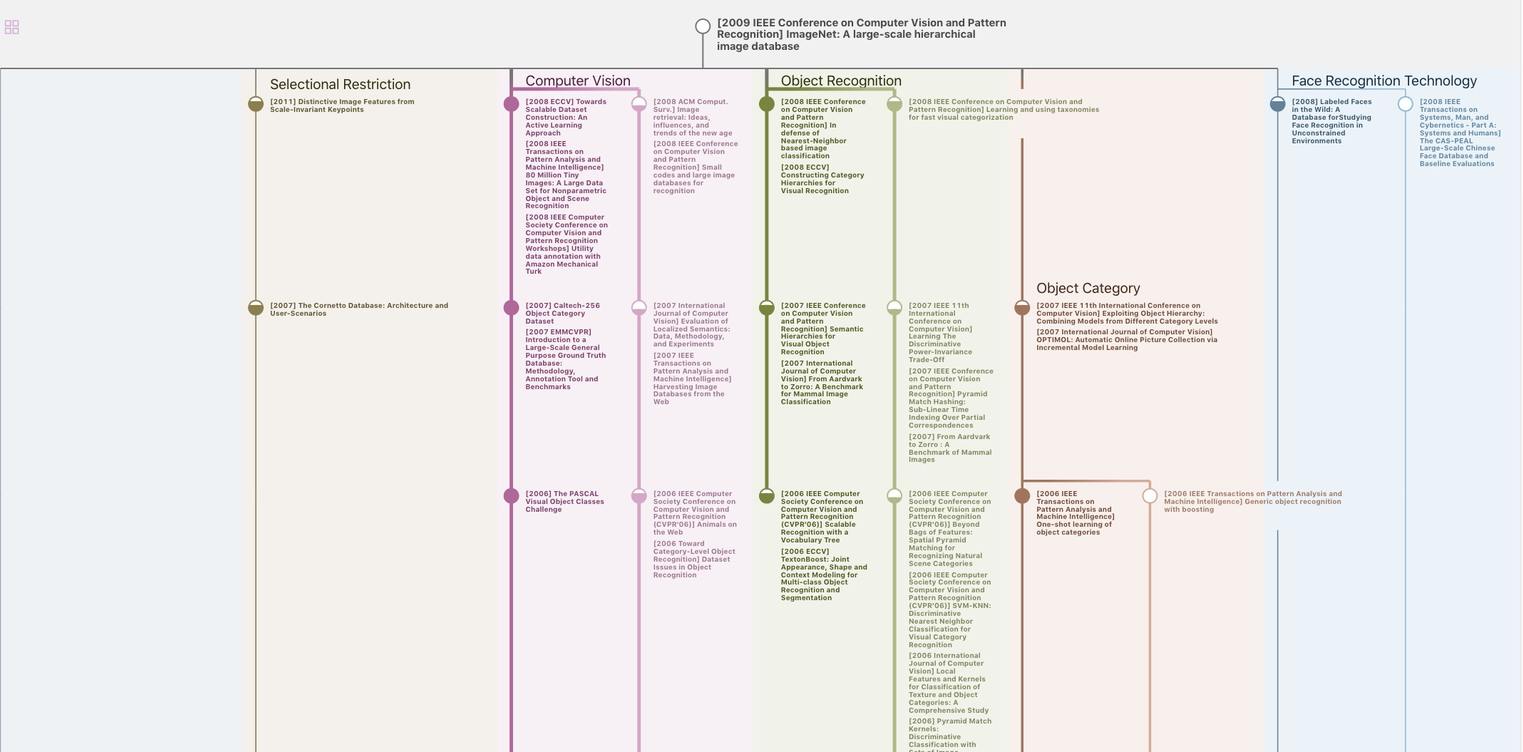
生成溯源树,研究论文发展脉络
Chat Paper
正在生成论文摘要