RMAP-ResNet: Segmentation of brain tumor OCT images using residual multicore attention pooling networks for intelligent minimally invasive theranostics
BIOMEDICAL SIGNAL PROCESSING AND CONTROL(2024)
摘要
Background and objective: The occurrence of brain tumors seriously affects patients both mentally and physically, reducing their quality of life. Automatic recognition and segmentation of tumor boundaries are essential for computer-aided diagnosis and treatment. While optical coherence tomography (OCT) has been widely employed in the detection and analysis fundus lesions and vascular segmentation due to its high resolution and real-time imaging advantages, the segmentation of brain tumor OCT images based on deep neural network has not been studied. However, despite the encoding and decoding structure based on deep learning has achieved good results in medical image segmentation, these models still face limitations in accurately segmenting tumor OCT images due to variations in tumor shapes, sizes, and numbers, and the low contrast of OCT images. Accurate segmentation of tumor tissue contours is crucial as the absence of this mechanism hampers the effective application of OCT imaging methods for brain tumor diagnosis and treatment in clinical practice. Therefore, we propose a novel neural network that can accurately segment brain tumors and their residual tissues in OCT images. Methods: In this study, we propose a novel residual multi-core attention pooling network (RMAP ResNet) for the segmentation of tumorous and its residual tissue. The RMAP module primarily relies on multiple associative effective receptive fields. These fields enable the detection of targets of various sizes. The module also incorporates an attention structure to adjust connection weights, suppressing irrelevant information and emphasizing the characteristics of tumor OCT images. This process restores the contextual dependencies. Results: We validated our proposed RMAP ResNet method on the dataset of mouse tumor OCT imaging obtained using the sweep source OCT imaging system. The experimental results demonstrate that RMAP ResNet achieves superior performance in tumor tissue segmentation. The proposed method improves the quality of tumor segmentation for various shapes and sizes, leading to superior results. RMAP ResNet achieved Dice and IoU values of 94.78% and 90.58%, respectively, confirming the effectiveness and advancement of our proposed technology. Conclusions: Our proposed method addresses the crucial issue of the limited use of the OCT imaging modality in tumor resection surgery. It overcomes the challenge of visually identifying the tumor boundary in OCT modality imaging, where physicians using the naked eye may encounter difficulties. This study has potential applications in the fields of surgical navigation and intelligent minimally invasive treatment of brain tumors.
更多查看译文
关键词
Optical coherence tomography,Image segmentation,Brian tumor,Residual multi-core attention pooling module,Intelligent theranostics
AI 理解论文
溯源树
样例
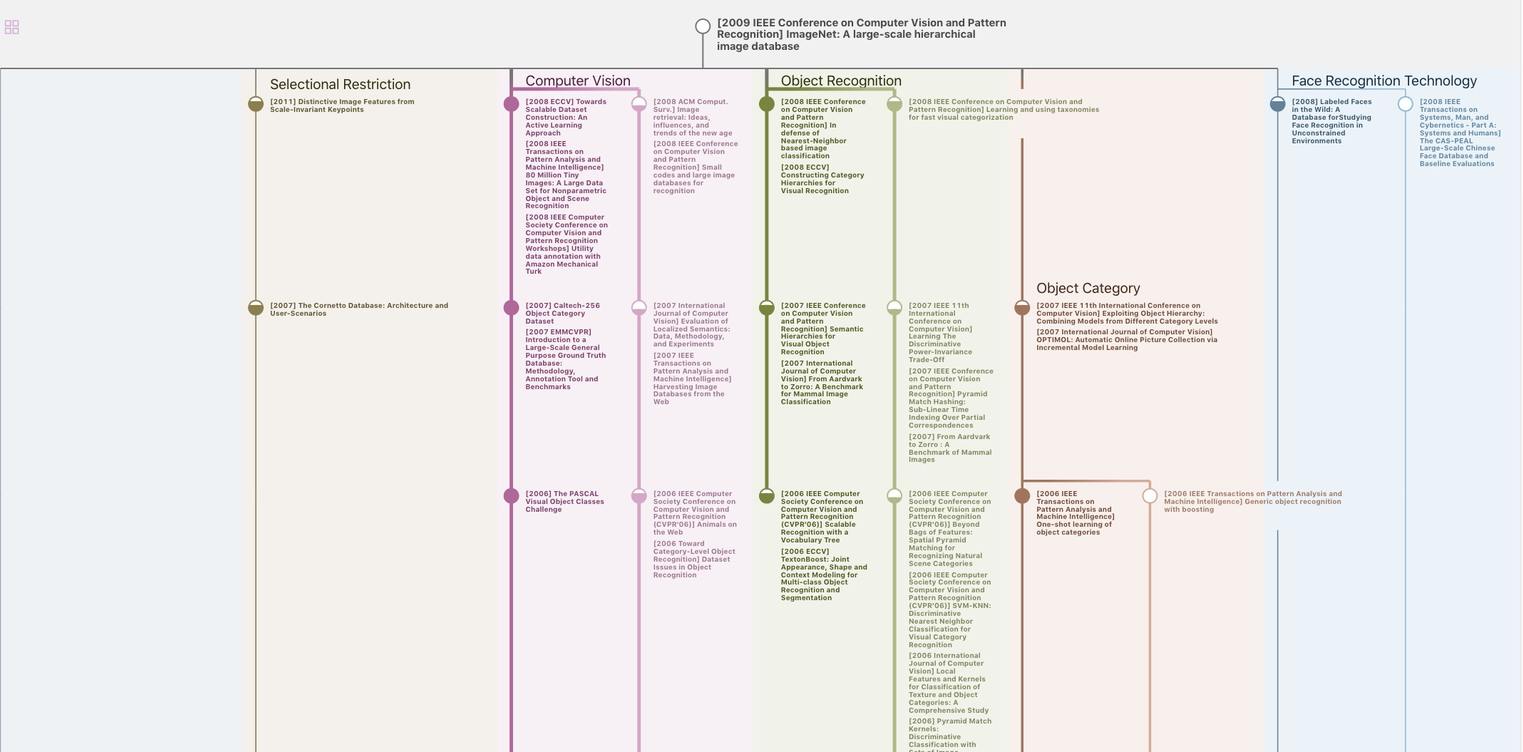
生成溯源树,研究论文发展脉络
Chat Paper
正在生成论文摘要