A Long-Term Actor Network for Human-Like Car-Following Trajectory Planning Guided by Offline Sample-Based Deep Inverse Reinforcement Learning
IEEE TRANSACTIONS ON AUTOMATION SCIENCE AND ENGINEERING(2023)
摘要
Human-like autonomous driving can enhance user acceptance and integration within traffic. In light of this, this paper presents a planning method for the human-like longitudinal trajectory in car-following scenarios with offline sample-based maximum entropy deep inverse reinforcement learning (DIRL). Specifically, the proposed method doesn't mimic human driving behavior directly. Instead, it uses naturalistic driving data to learn the internal reward function that leads to these driving behaviors. To enhance the capacity for fitting the human reward function, DIRL leverages deep neural networks to replace linear functions used by traditional IRL. However, the long-tail effect of naturalistic driving data makes it challenging for DIRL to capture the reward function in edge scenarios. A simulated dataset covering edge scenarios is collected by employing feature-based inverse reinforcement learning to deal with this challenge. Furthermore, this paper trains a long-term actor network guided by DIRL's reward network. The long-term actor network significantly reduces the computation cost by three orders of magnitude compared to the reward network-based method, while also avoiding system oscillation in contrast to the traditional one-step actor network. The simulation experiments confirm that the planning results from the proposed method are closer to human drivers' behavior than the baseline. And, the hardware-in-the-loop experiment results affirm the proposed method's effectiveness and good real-time performance. Note to Practitioners-Human-like autonomous driving can enhance user acceptance and integration within traffic. This paper proposes a planning method for human-like car-following driving behaviors using offline sample-based deep inverse reinforcement learning (DIRL). Instead of mimicking human trajectories directly, the method learns the internal reward function leading to these driving behaviors. To address challenges posed by naturalistic driving data's long-tail effect, a simulated dataset covering edge scenarios is collected by employing feature-based inverse reinforcement learning. Additionally, this paper trains a long-term actor network guided by DIRL's reward network to reduce the computation cost. The simulation experiments confirm that the planning results from the proposed method are closer to human drivers' behavior than the baseline. And, the hardware-in-the-loop experiment results affirm the proposed method's effectiveness and good real-time performance.
更多查看译文
关键词
Human-like,planning,car-following,deep inverse reinforcement learning,autonomous driving
AI 理解论文
溯源树
样例
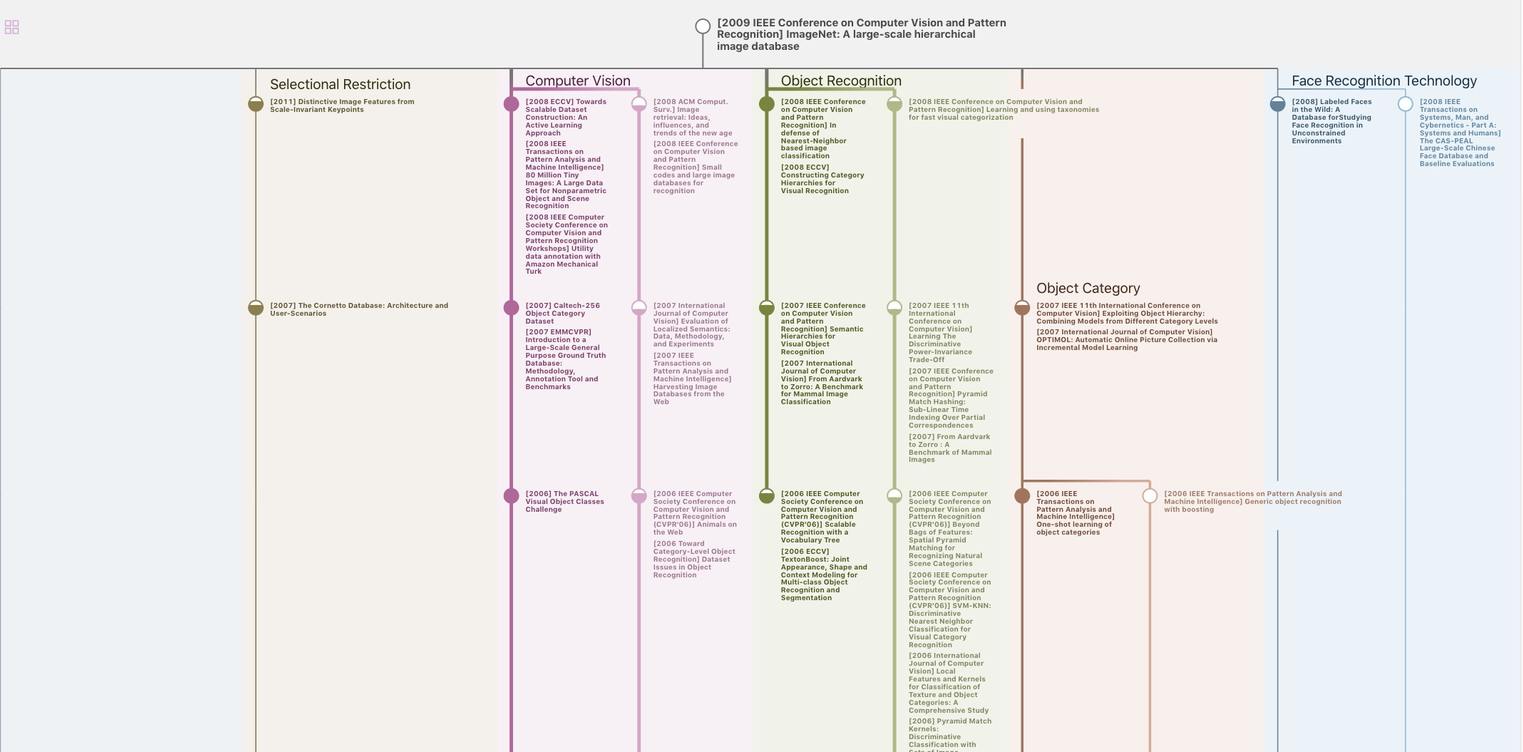
生成溯源树,研究论文发展脉络
Chat Paper
正在生成论文摘要