Attention to Task-Aligned Object Detection for End-Edge-Cloud Video Surveillance.
IEEE Internet Things J.(2024)
摘要
Edge computing extends computing power down to the network edge near the sensors and provides promising solutions to address high bandwidth requirements and latency-sensitive problems in smart video surveillance. Deep learning-based object detection has made significant progress in accuracy and is playing an important role in video surveillance. However, the computation and storage resources are still far below the advances reached by cloud servers, making it difficult to migrate traditional object detection models to the resource-constrained edge. Thus, in this paper, we propose a new lightweight object detection model, called AT-YOLO to detect key targets in the video stream. The efficient single compressed dense block (SCDB) is introduced to build the backbone network of AT-YOLO, which improves the computational efficiency of the network. Besides, the Attention Guide Task-aligned Head (AGT-head), which enhances the classification and the localization features, and encourages the interaction and the alignment of both, is proposed to improve the detection accuracy. Considering the redundancy in video streams and the improvement of detection efficiency, a Convolutional Neural Network (CNN)-based keyframe algorithm is considered as it has only 0.32 M parameters and can accurately and quickly filter out keyframes. We deployed the CNN-based keyframe algorithm and AT-YOLO on the edge devices of the system to implement an intelligent surveillance system based on edge computing. Experiments and evaluations on COCO demonstrate that AT-YOLO achieves 29.9% mAP at 3.47 M parameters. Moreover, in experiments with real devices, our approach can reduce the bandwidth requirement to 467.29 Kbps. And it achieves a processing speed of 13.69 FPS on the Raspberry Pi 4B, which is a single-board computer. With the more powerful device selected, the better real-time performance it can get.
更多查看译文
关键词
Edge Computing,Deep Learning,Lightweight Neural Network,Object Detection,Smart Video Surveillance
AI 理解论文
溯源树
样例
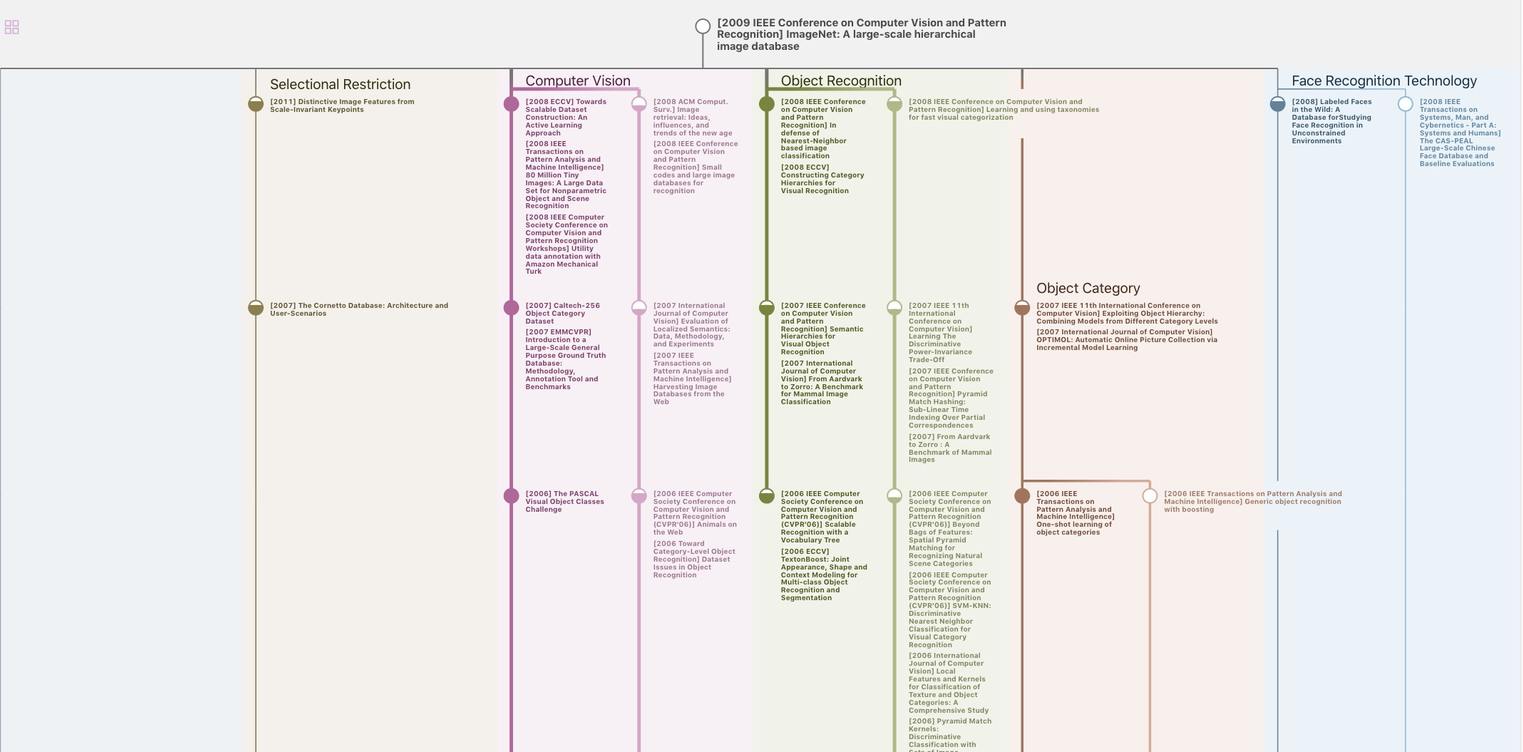
生成溯源树,研究论文发展脉络
Chat Paper
正在生成论文摘要