Large-Scale Spatiotemporal Fracture Data Completion in Sparse CrowdSensing
IEEE transactions on mobile computing(2023)
摘要
Mobile CrowdSensing (MCS) is a widely adopted approach that involves engaging mobile users to collaboratively perform diverse sensing tasks. In Sparse CrowdSensing, the completion of data from partially-sensed sources plays a pivotal role in urban sensing applications. This process is essential as it enables efficient data representation, enhances urban analysis capabilities, and ultimately facilitates informed city planning decisions. By leveraging the power of mobile users, Sparse CrowdSensing contributes to the comprehensive understanding of urban environments, enabling effective utilization of data for optimizing urban infrastructure and fostering sustainable urban development. To achieve accurate completion results, previous methods usually utilize the universal similarity and conventional tendency while incorporating only a single dataset to infer the full map. However, in real-world scenarios, there may exist many kinds of data (inter-data), that could help to complement each other. Moreover, for each kind of data (intra-data), there usually exists a few but important spatiotemporal fracture data which caused by the special events (e.g. data loss, equipment failure, etc.), which may behave in a different way as the statistical patterns. Thus, it is an essential task to consider spatiotemporal fracture data caused by the special cases in spatiotemporal data inference, especially using both intra- and inter-data, because of the following challenges: 1) the sparsity of the sensed data, 2) the complex spatiotemporal relations and 3) the uncertain scale of a spatiotemporal fracture. To this end, focusing on the large-scale spatiotemporal fracture, we propose a data completion method that exploits both intra- and inter-data correlations for enhancing performance. Specifically, for the purpose of generating spatiotemporal fracture data, there is S tacked G enerative M atrix C ompletion (SGMC) by combining previous Stacked Deep Matrix Factorization (SDMF) and Generative Adversarial Networks (GANs), which improves a lot. Along this line, we extract the features of spatiotemporal data and further efficiently complete and predict the unsensed data. Finally, we conduct both qualitative and quantitative experiments on two different datasets, and the results demonstrate that the performance of our method outperforms the state-of-the-art baselines.
更多查看译文
关键词
Spatiotemporal phenomena,Crowdsensing,Sparse matrices,Correlation,Sensors,Mobile computing,Task analysis,Data inference,intra- and inter-data correlation,mobile CrowdSensing,spatiotemporal fracture data
AI 理解论文
溯源树
样例
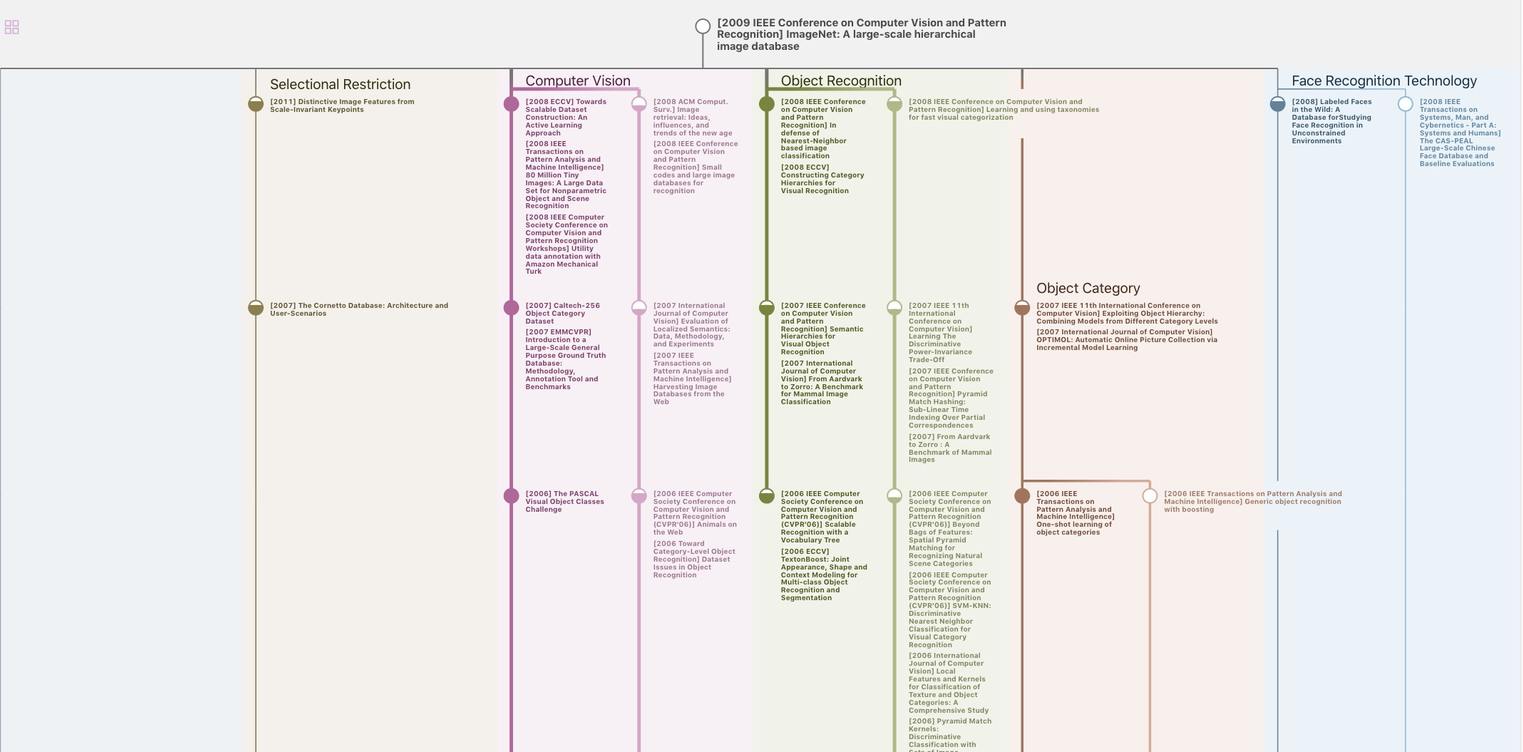
生成溯源树,研究论文发展脉络
Chat Paper
正在生成论文摘要