Never train an LSTM on a single basin
crossref(2023)
摘要
Machine learning (ML) has an increasing role in the hydrological sciences, and in particular, certain types of time series modeling strategies are popular for rainfall-runoff modeling. A large majority of studies that use this type of model do not follow best practices, and there is one mistake in particular that is very common: training deep learning models on small, homogeneous data sets (i.e., data from one or a small number of watersheds). In this position paper, we argue why it is not a good idea to train a Long Short Term Memory (LSTM) model on data from a single watershed. Instead, deep learning streamflow models are best when trained with a large amount of hydrologically diverse data.
更多查看译文
AI 理解论文
溯源树
样例
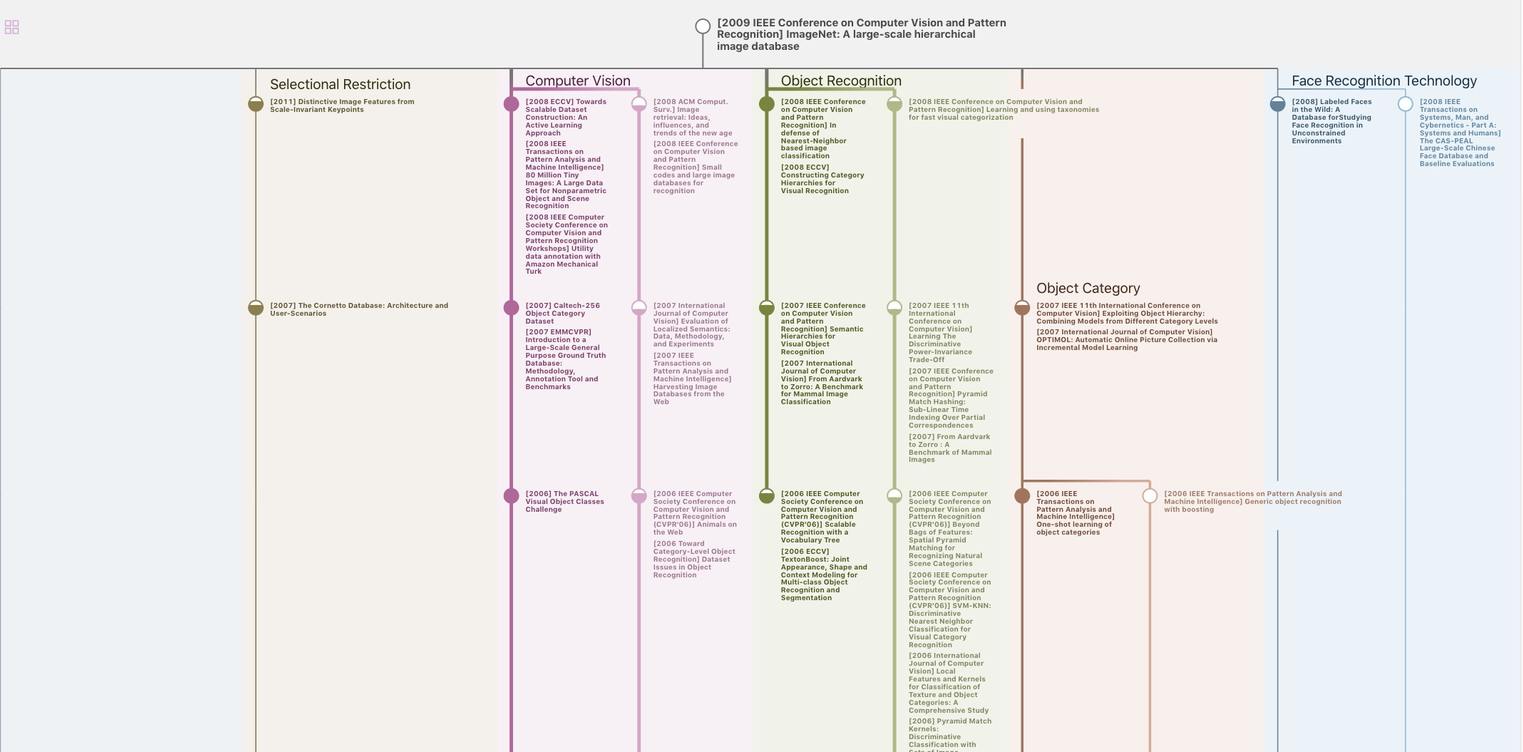
生成溯源树,研究论文发展脉络
Chat Paper
正在生成论文摘要