SAGDTI: self-attention and graph neural network with multiple information representations for the prediction of drug-target interactions
BIOINFORMATICS ADVANCES(2023)
摘要
Motivation Accurate identification of target proteins that interact with drugs is a vital step in silico, which can significantly foster the development of drug repurposing and drug discovery. In recent years, numerous deep learning-based methods have been introduced to treat drug-target interaction (DTI) prediction as a classification task. The output of this task is binary identification suggesting the absence or presence of interactions. However, existing studies often (i) neglect the unique molecular attributes when embedding drugs and proteins, and (ii) determine the interaction of drug-target pairs without considering biological interaction information.Results In this study, we propose an end-to-end attention-derived method based on the self-attention mechanism and graph neural network, termed SAGDTI. The aim of this method is to overcome the aforementioned drawbacks in the identification of DTI. SAGDTI is the first method to sufficiently consider the unique molecular attribute representations for both drugs and targets in the input form of the SMILES sequences and three-dimensional structure graphs. In addition, our method aggregates the feature attributes of biological information between drugs and targets through multi-scale topologies and diverse connections. Experimental results illustrate that SAGDTI outperforms existing prediction models, which benefit from the unique molecular attributes embedded by atom-level attention and biological interaction information representation aggregated by node-level attention. Moreover, a case study on severe acute respiratory syndrome coronavirus 2 (SARS-CoV-2) shows that our model is a powerful tool for identifying DTIs in real life.Availability and implementation The data and codes underlying this article are available in Github at https://github.com/lixiaokun2020/SAGDTI.
更多查看译文
关键词
multiple information representations,graph,prediction,self-attention,drug-target
AI 理解论文
溯源树
样例
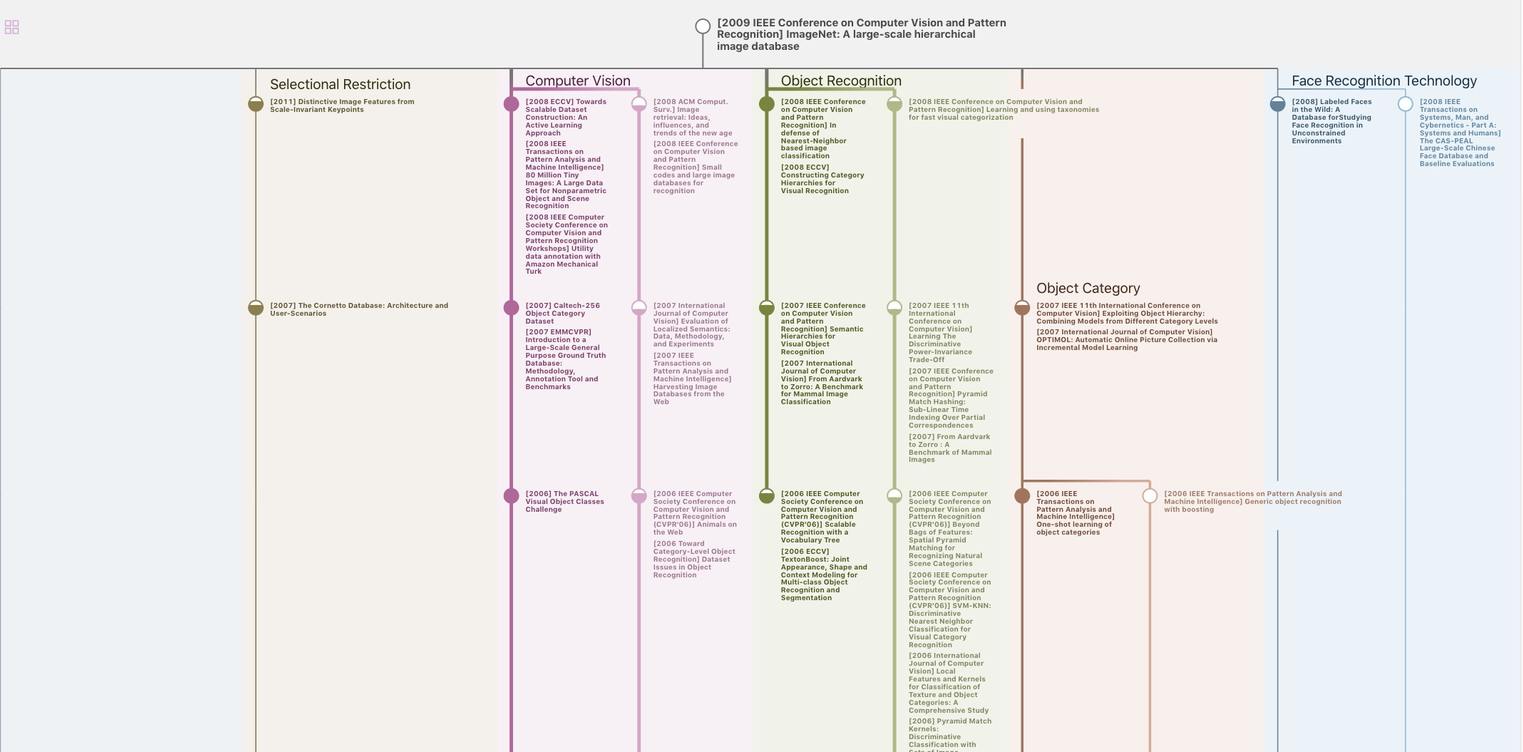
生成溯源树,研究论文发展脉络
Chat Paper
正在生成论文摘要