Deep Learning-Based Robust DC Fault Protection Scheme for Meshed HVDC Grids
CSEE JOURNAL OF POWER AND ENERGY SYSTEMS(2023)
摘要
Fast and reliable detection of faults is a significant technical challenge in transient-based protection for a modular multi-level converter (MMC) based high voltage direct current (HVDC) system. This is because existing protection schemes rely heavily upon setting a complicated protective threshold, the failure of which causes high DC-fault currents in HVDC grids, and MMC is prone to such strong transient currents. In this context, this paper proposes a DC-line fault diagnosis technique based on a tuned long-short-term memory (LSTM) algorithm to improve the response and accuracy of transient-based protection. The discrete wavelet transform (DWT) extracts the transient features of DC-line voltages in the frequency-time domain. Many healthy and faulty samples are incorporated during training even by considering the noise influence. After training, numerous test samples are run to evaluate the proposed algorithm's robustness under various fault conditions. Test results show the proposed algorithm can detect DC faults and has a high recognition accuracy of 98.6%. Compared to contemporary techniques, it can perform well to identify DC-line faults because of the efficient training of characteristic features.
更多查看译文
关键词
Training,Discrete wavelet transforms,Logic gates,Computer architecture,HVDC transmission,Feature extraction,Voltage,High voltage direct current (HVDC),long-short-term-memory (LSTM),primary protection,voltage source converters (VSC)
AI 理解论文
溯源树
样例
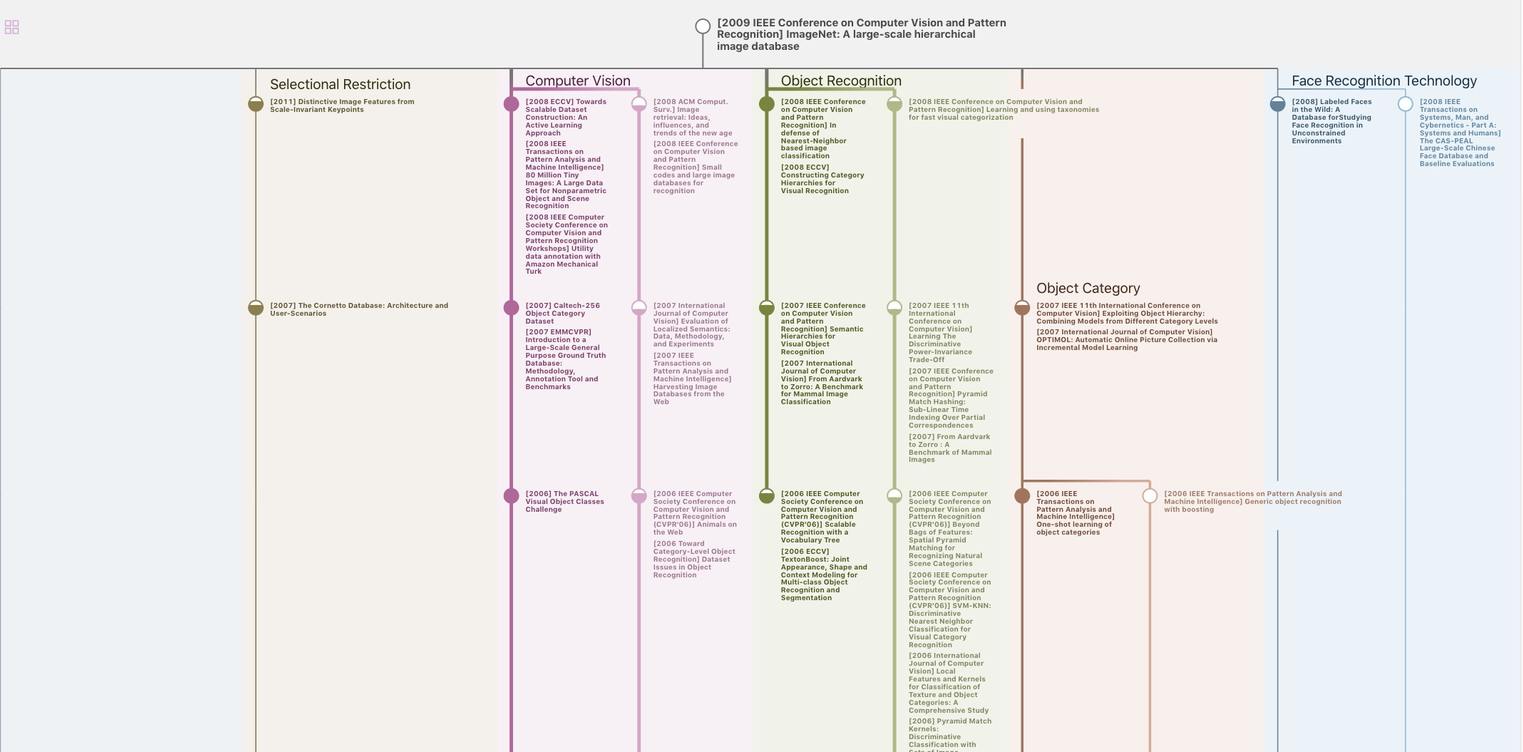
生成溯源树,研究论文发展脉络
Chat Paper
正在生成论文摘要