Co-MDA: Federated Multisource Domain Adaptation on Black-Box Models
IEEE TRANSACTIONS ON CIRCUITS AND SYSTEMS FOR VIDEO TECHNOLOGY(2023)
摘要
Federated domain adaptation (FDA) is an effective method for performing learning tasks over distributed networks, which well improves data privacy and portability in unsupervised multi-source domain adaptation (UMDA) tasks. Despite the impressive gains achieved, two common limitations exist in current FDA works. First, most previous studies require access to the model parameters or gradient details of each source party. However, the raw source data can be reconstructed from the model gradients or parameters, which may leak individual information. Second, these works assume that different parties share an identical network architecture, which is impractical and not desirable for low- or high-resource target users. To address these issues, in this work, we propose a more practical UMDA setting, called Federated Multi-source Domain Adaptation on Black-box Models (B2FDA), where all data are stored locally and only the input-output interface of the source model is available. To tackle B2FDA, we propose an effective method, termed Co2-Learning with Multi-Domain Attention (Co-MDA). Experiments on multiple benchmark datasets demonstrate the effectiveness of our proposed method. Notably, Co-MDA performs comparably with traditional UMDA methods where the source data or the trained model are fully available.
更多查看译文
关键词
Multi-source domain adaptation,transfer learning,federated learning
AI 理解论文
溯源树
样例
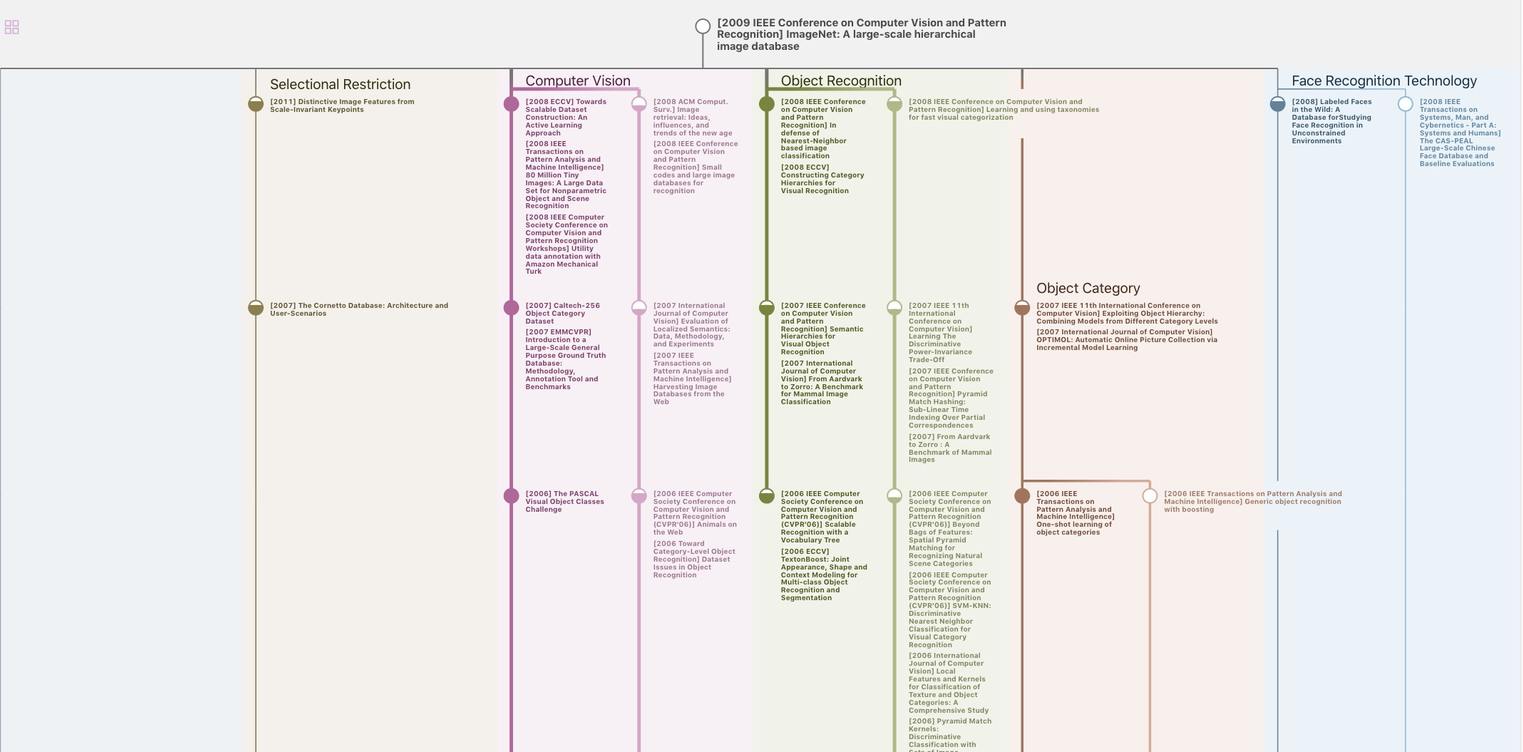
生成溯源树,研究论文发展脉络
Chat Paper
正在生成论文摘要