Toward Optimal Real-Time Volumetric Video Streaming: A Rolling Optimization and Deep Reinforcement Learning Based Approach
IEEE TRANSACTIONS ON CIRCUITS AND SYSTEMS FOR VIDEO TECHNOLOGY(2023)
摘要
Volumetric video provides users with a good viewing experience of six degrees of freedom (DoF) and has wide applications in many fields such as teleconferencing and online games. However, the huge data volume and strict latency requirements of point cloud video, the most popular representative of volumetric video, pose a challenge to its transmission. Existing point cloud video transmission algorithms usually segment a long video by every one or several group of frames, predict network bandwidth and field of view (FoV) information, then perform adaptive transmission by solving the quality of experience (QoE) optimization problem. However, such segmentation neglects the impact of current optimization decisions on the subsequent video streaming process, as well as the accumulated prediction error across a long interval, severely degrading user's QoE. Moreover, the complex constrained optimization problem makes the solution time too long to meet the real-time video streaming requirements. To this end, in this paper, we propose a rolling prediction-optimization-transmission (POT) framework, which makes predictions of network bandwidth and FoV in each short rolling window to reduce prediction error. And our framework takes into account the upper bounded QoE contribution of the subsequent point cloud video to improve the system performance. In addition, we design a deep reinforcement learning based real-time solver to make decisions for the fixed structure optimization problem in each roll, allowing our system to run in real-time. We have performed simulations and experiments, and the results show that our solution outperforms existing methods.
更多查看译文
关键词
Point cloud video,video streaming,real-time transmission,QoE model,deep reinforcement learning,rolling optimization
AI 理解论文
溯源树
样例
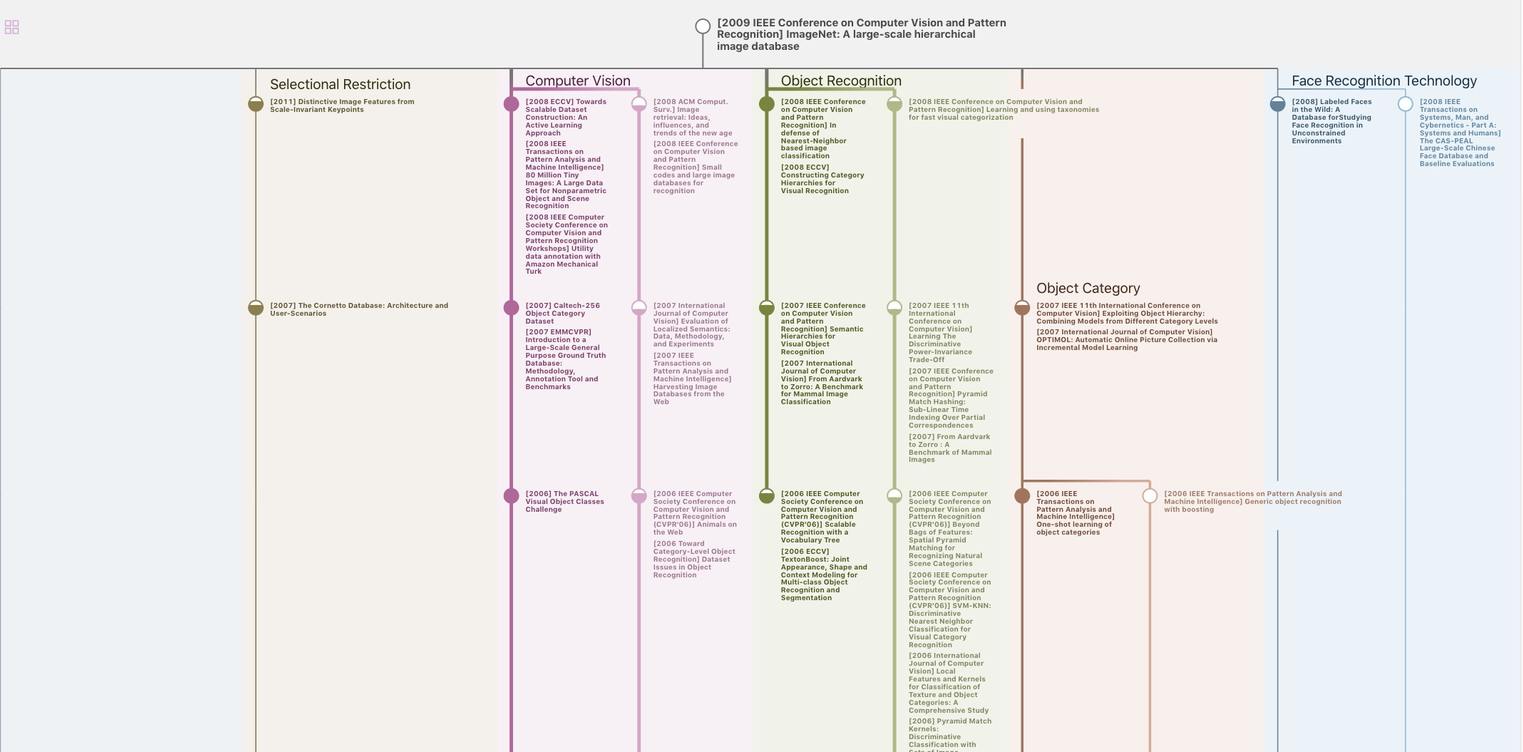
生成溯源树,研究论文发展脉络
Chat Paper
正在生成论文摘要