Research on the Cough-Related Disease Classification and Recognition Method Combining Attention Mechanism with Residual Neural Networks (Preprint)
crossref(2023)
摘要
BACKGROUND Coughing, a common clinical symptom, is a protective respiratory reflex associated with various respiratory disorders. Due to its diverse acoustic characteristics resulting from different underlying causes, the analysis of a patient's cough sounds provides valuable insights for physicians in diagnosing associated diseases. OBJECTIVE The aim of this study is to present an improved method for the detection of cough-related diseases based on deep learning, aimed at assisting in the diagnosis of relevant diseases. METHODS In this study, we conducted extensive data collection, which included voluntary cough audio recordings from patients with well-diagnosed respiratory diseases (such as chronic obstructive pulmonary disease, lung cancer, COVID-19, and pneumonia), as well as healthy participants,We collected a total of 2,610 audio samples.We added the Channel Attention Mechanism CAM to the last convolutional block of each residual block in the ResNet18 neural network, and later constructed the CAM-ResNet18 neural network model. We transformed the collected cough audio recordings into spectrograms and established the corresponding dataset, utilizing these spectrograms as input data for the CAM-ResNet18 neural network model. Subsequently, we trained the CAM-ResNet18 neural network model using the training set within this dataset,adjust the model parameters and train the model until it converges. Finally, we input the spectrograms from the test set into the pre-trained neural network model for precise classification. RESULTS Through experiments conducted on the audio dataset we collected, our proposed improvement method achieved an accuracy of 83.9% in classifying these five types of cough audio, while the traditional ResNet18 model had an accuracy of 78.16%. CONCLUSIONS The experimental results of this study validate the effectiveness of the proposed method. The uniqueness of this model lies in its enhanced ability to focus on critical features, effectively reducing overfitting issues. As we continue to expand the cough audio dataset in future work, we anticipate further improvements in classification accuracy. The findings of this study hold significant potential application value in clinical diagnosis.
更多查看译文
AI 理解论文
溯源树
样例
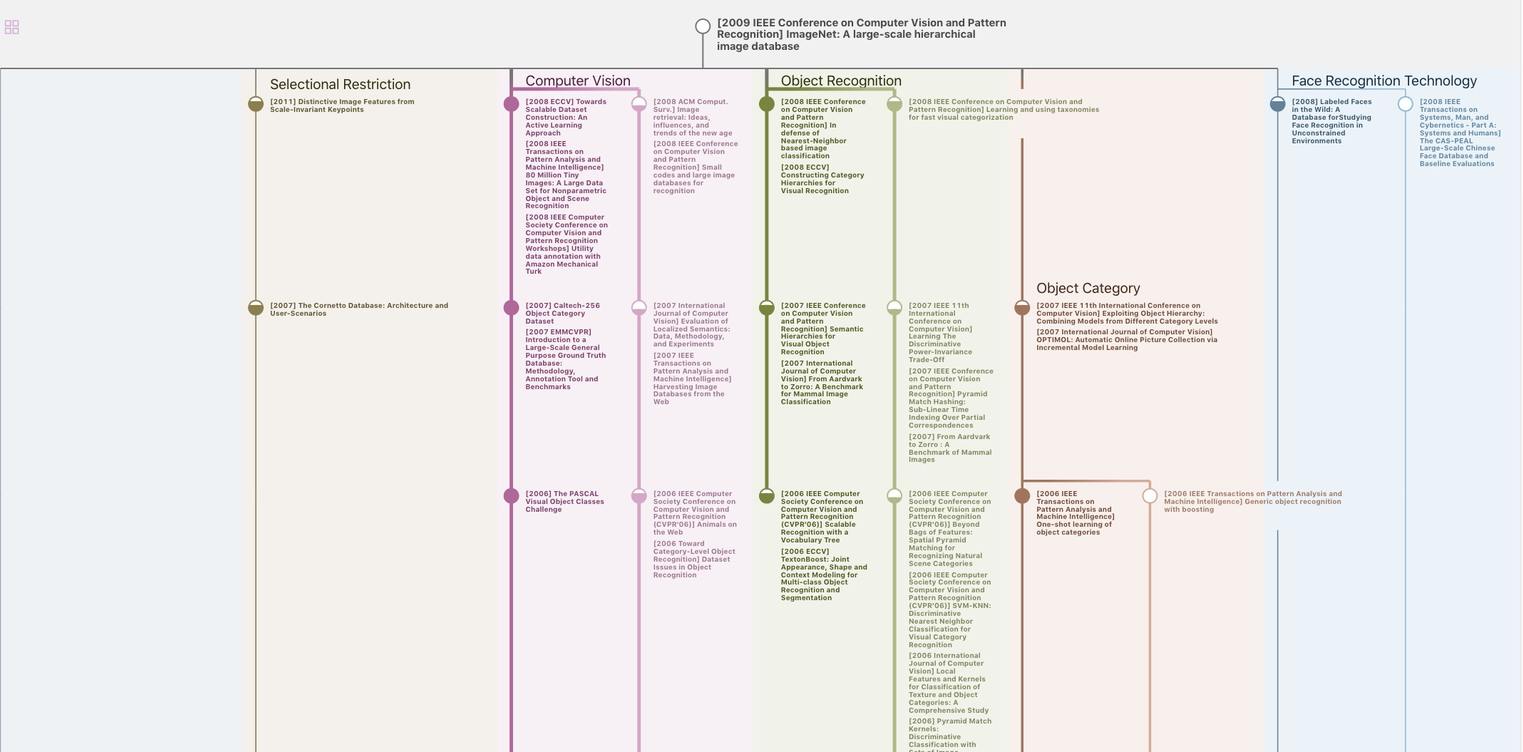
生成溯源树,研究论文发展脉络
Chat Paper
正在生成论文摘要