Classifying hypertension mediated left ventricular hypertrophy phenotypes from the 12-lead electrocardiogram using machine learning
European Heart Journal - Cardiovascular Imaging(2023)
摘要
Abstract Funding Acknowledgements Type of funding sources: Foundation. Main funding source(s): British Heart Foundation Pat Merriman Clinical Research Training Fellowship Background Hypertension is the commonest cause of left ventricular hypertrophy (LVH), an established independent predictor of cardiovascular morbidity and mortality. Four distinct LVH phenotypes with varying prognostic implications have been reported using cardiac magnetic resonance (CMR) LV mass to volume ratio: normal LV, LV remodelling, eccentric LVH and concentric LVH. Current electrocardiogram (ECG) criteria can detect LVH but their ability to differentiate between hypertension mediated LVH phenotypes is unknown. Purpose The aim of this study was to classify hypertension mediated LVH phenotypes using 12-lead electrocardiogram (ECG) and clinical data for cost-effective patient stratification. Methods 24,535 hypertensive participants from UK Biobank imaging study were categorised into the CMR-defined LVH phenotypes. ECG biomarkers with a physiological association with LVH (including QRS biomarkers, Sokolow-Lyon and Cornell voltage) were extracted from the 12-lead ECG of each participant using in-house algorithms. Vascular risk factors known to be associated with LVH were also ascertained from the clinical data of each participant. Classification models integrating ECG biomarkers and clinical data were built using logistic regression, support vector machine (SVM) and random forest (RF). The dataset was split into 80% train and 20% test sets for performance measurement. Ten-fold cross validation was applied and the training set was downsampled to the minority class. Results Among the participants (mean age ± standard deviation 65.3 ± 7.4 years), 23,312 (95.0%) had normal LV, 900 (3.7%) LV remodelling, 220 (0.8%) eccentric LVH and 103 (0.4%) concentric LVH. R wave amplitude V5, S wave amplitude V3 and QT duration V6 (P<0.001) were the top ECG biomarkers and systolic blood pressure (p<0.001) the top clinical predictor of LVH phenotypes (Figure 1). Classification with logistic regression had an accuracy of 77% (sensitivity 55%, specificity 85%, Area under the receiver operator curve [AUC] 0.76), SVM 80% accuracy (sensitivity 61%, specificity 87%, AUC 0.75) and RF 75% accuracy (sensitivity 49%, specificity 83%, AUC 0.75). ECG biomarkers enhanced model performance of all classifiers, compared to using clinical data alone. Conclusion A combination of automatically extracted ECG biomarkers and clinical data were able to discriminate the hypertension mediated LVH phenotypes. An advanced classification approach improves selection of hypertensive patients with LVH, thereby adding prognostic value to traditional risk models. We are currently validating our findings in an external population and using deep learning for comparison.
更多查看译文
关键词
ventricular hypertrophy phenotypes,hypertension,machine learning
AI 理解论文
溯源树
样例
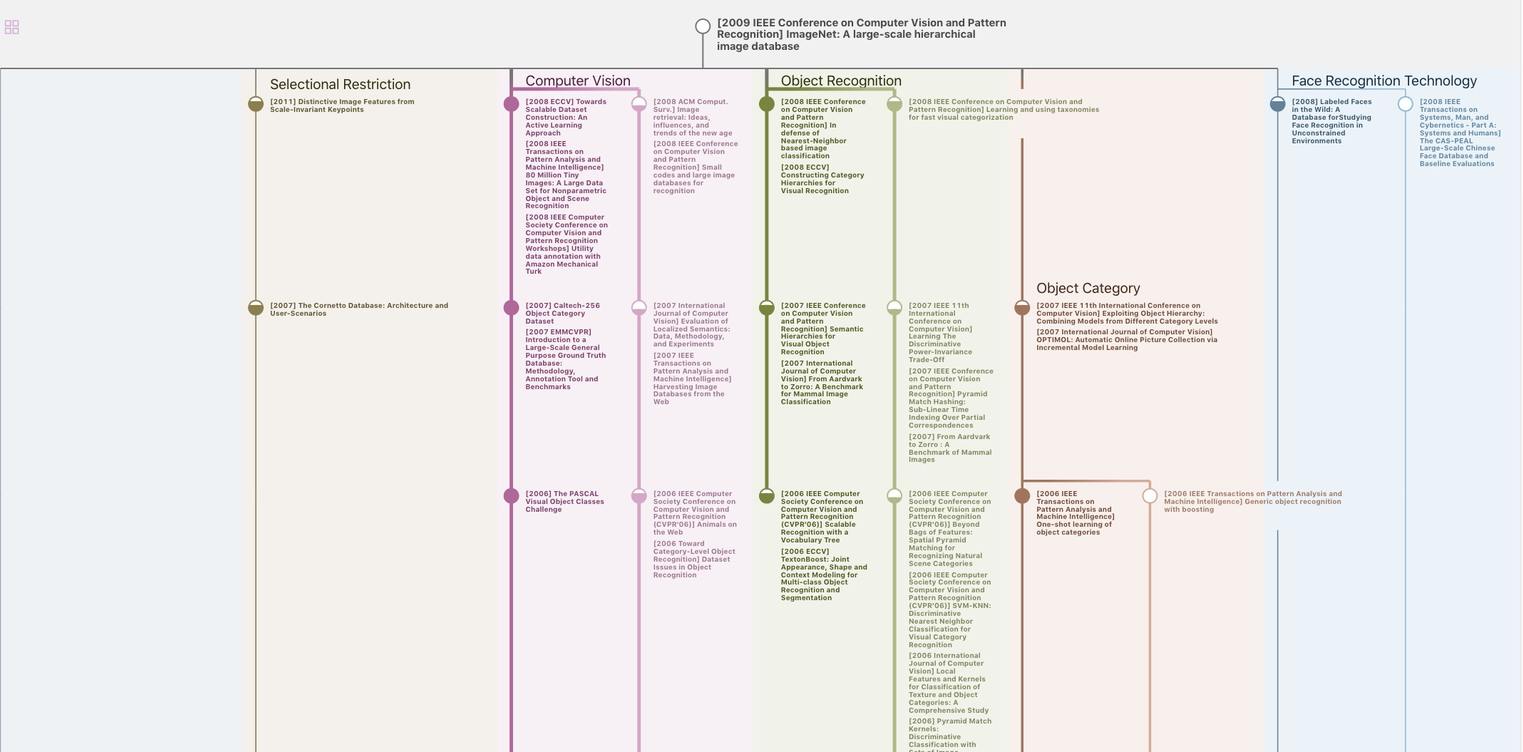
生成溯源树,研究论文发展脉络
Chat Paper
正在生成论文摘要