Reconstructing 2-D Basement Relief Using Gravity Data by Deep Neuron Network: An Application on Poyang Basin
IEEE TRANSACTIONS ON GEOSCIENCE AND REMOTE SENSING(2024)
摘要
The stark contrast in density between geological layers is a fundamental aspect in the examination of basic geological structures. The delineation between the crystalline basement and sedimentary layers, moreover, is pivotal in the pursuit of strategic energy resources, such as petroleum and natural gas. Traditional full space density inversion, however, is beleaguered by issues of stability and resolution, impeding the accurate characterization of the sharp density interface. To rectify these shortcomings, we introduce an innovative methodology for estimating 2-D depth-to-basement and overlying density distribution, employing a deep neural network with a leaky rectified linear unit as an activation function. Evaluation of the proposed method on simulated sedimentary basin models underscores its superior ability to discern complex geometries of basin boundaries and overlying density, despite the presence of various degrees of Gaussian noise. In practical application to the Poyang basin, the relief of the Cretaceous basement is proficiently recovered through vertical gravity field data, with validation provided by corresponding seismic sections and well-established stratigraphic markers.
更多查看译文
关键词
Basement relief,deep neuron network,inversion,vertical gravity data
AI 理解论文
溯源树
样例
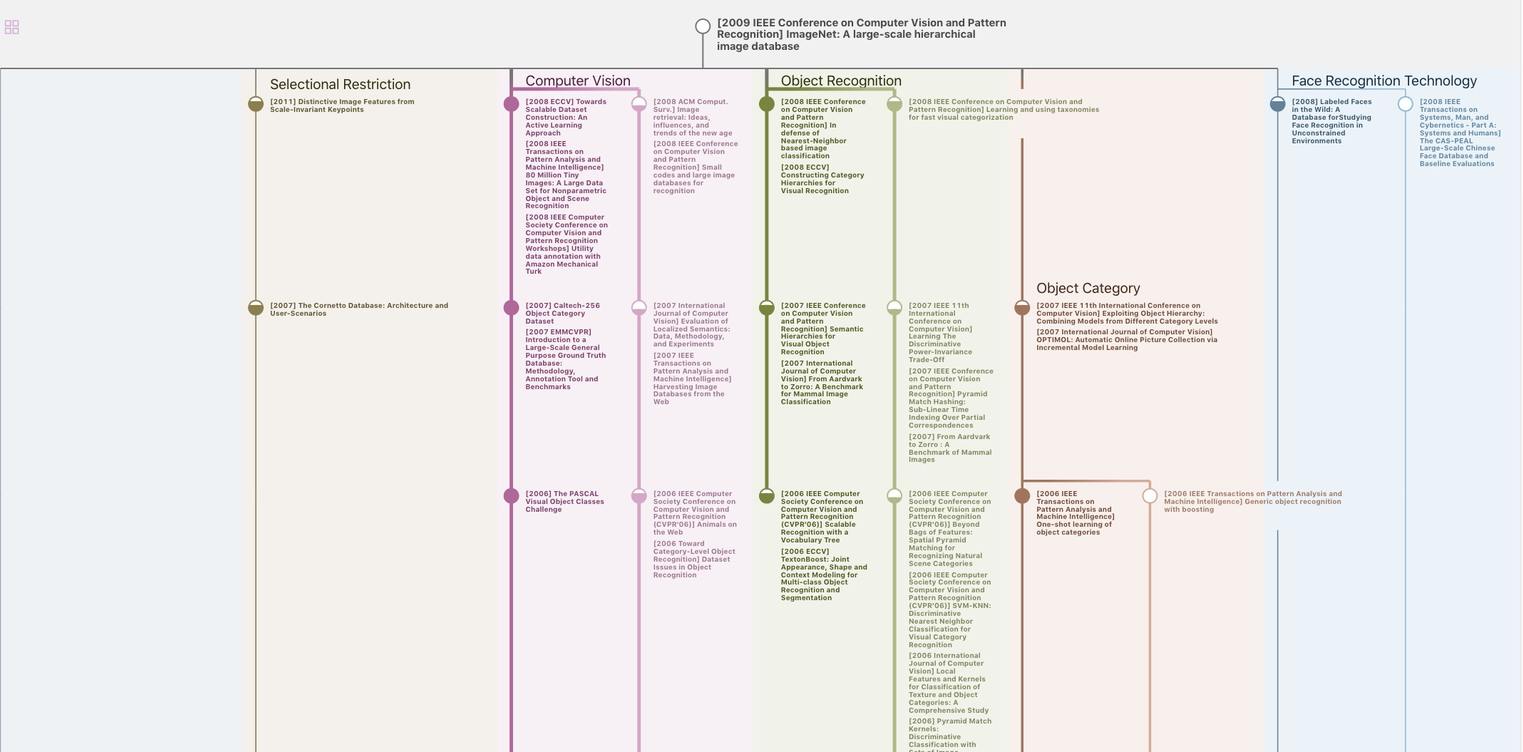
生成溯源树,研究论文发展脉络
Chat Paper
正在生成论文摘要