More Reliable Neighborhood Contrastive Learning for Novel Class Discovery in Sensor-Based Human Activity Recognition
SENSORS(2023)
摘要
Human Activity Recognition (HAR) systems have made significant progress in recognizing and classifying human activities using sensor data from a variety of sensors. Nevertheless, they have struggled to automatically discover novel activity classes within massive amounts of unlabeled sensor data without external supervision. This restricts their ability to classify new activities of unlabeled sensor data in real-world deployments where fully supervised settings are not applicable. To address this limitation, this paper presents the Novel Class Discovery (NCD) problem, which aims to classify new class activities of unlabeled sensor data by fully utilizing existing activities of labeled data. To address this problem, we propose a new end-to-end framework called More Reliable Neighborhood Contrastive Learning (MRNCL), which is a variant of the Neighborhood Contrastive Learning (NCL) framework commonly used in visual domain. Compared to NCL, our proposed MRNCL framework is more lightweight and introduces an effective similarity measure that can find more reliable k-nearest neighbors of an unlabeled query sample in the embedding space. These neighbors contribute to contrastive learning to facilitate the model. Extensive experiments on three public sensor datasets demonstrate that the proposed model outperforms existing methods in the NCD task in sensor-based HAR, as indicated by the fact that our model performs better in clustering performance of new activity class instances.
更多查看译文
关键词
human activity recognition,novel class discovery,neighborhood,contrastive learning,similarity,sensor
AI 理解论文
溯源树
样例
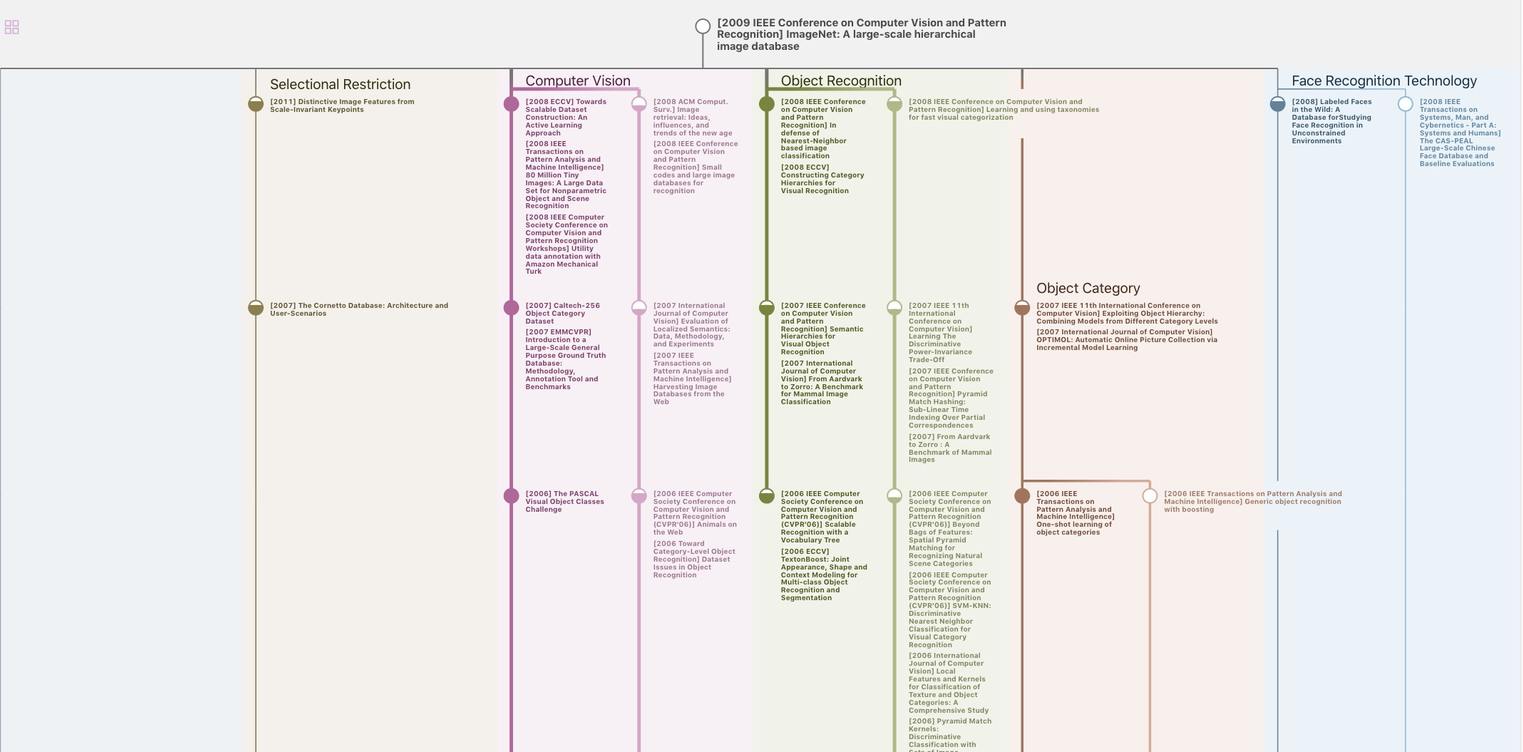
生成溯源树,研究论文发展脉络
Chat Paper
正在生成论文摘要