Combining Feature Selection Techniques and Neurofuzzy Systems for the Prediction of Total Viable Counts in Beef Fillets Using Multispectral Imaging
SENSORS(2023)
摘要
In the food industry, quality and safety issues are associated with consumers' health condition. There is a growing interest in applying various noninvasive sensorial techniques to obtain quickly quality attributes. One of them, hyperspectral/multispectral imaging technique has been extensively used for inspection of various food products. In this paper, a stacking-based ensemble prediction system has been developed for the prediction of total viable counts of microorganisms in beef fillet samples, an essential cause to meat spoilage, utilizing multispectral imaging information. As the selection of important wavelengths from the multispectral imaging system is considered as an essential stage to the prediction scheme, a features fusion approach has been also explored, by combining wavelengths extracted from various feature selection techniques. Ensemble sub-components include two advanced clustering-based neuro-fuzzy network prediction models, one utilizing information from average reflectance values, while the other one from the standard deviation of the pixels' intensity per wavelength. The performances of neurofuzzy models were compared against established regression algorithms such as multilayer perceptron, support vector machines and partial least squares. Obtained results confirmed the validity of the proposed hypothesis to utilize a combination of feature selection methods with neurofuzzy models in order to assess the microbiological quality of meat products.
更多查看译文
关键词
neural networks,ensemble systems,fuzzy logic,beef,total viable counts,regression,multispectral imaging,machine learning
AI 理解论文
溯源树
样例
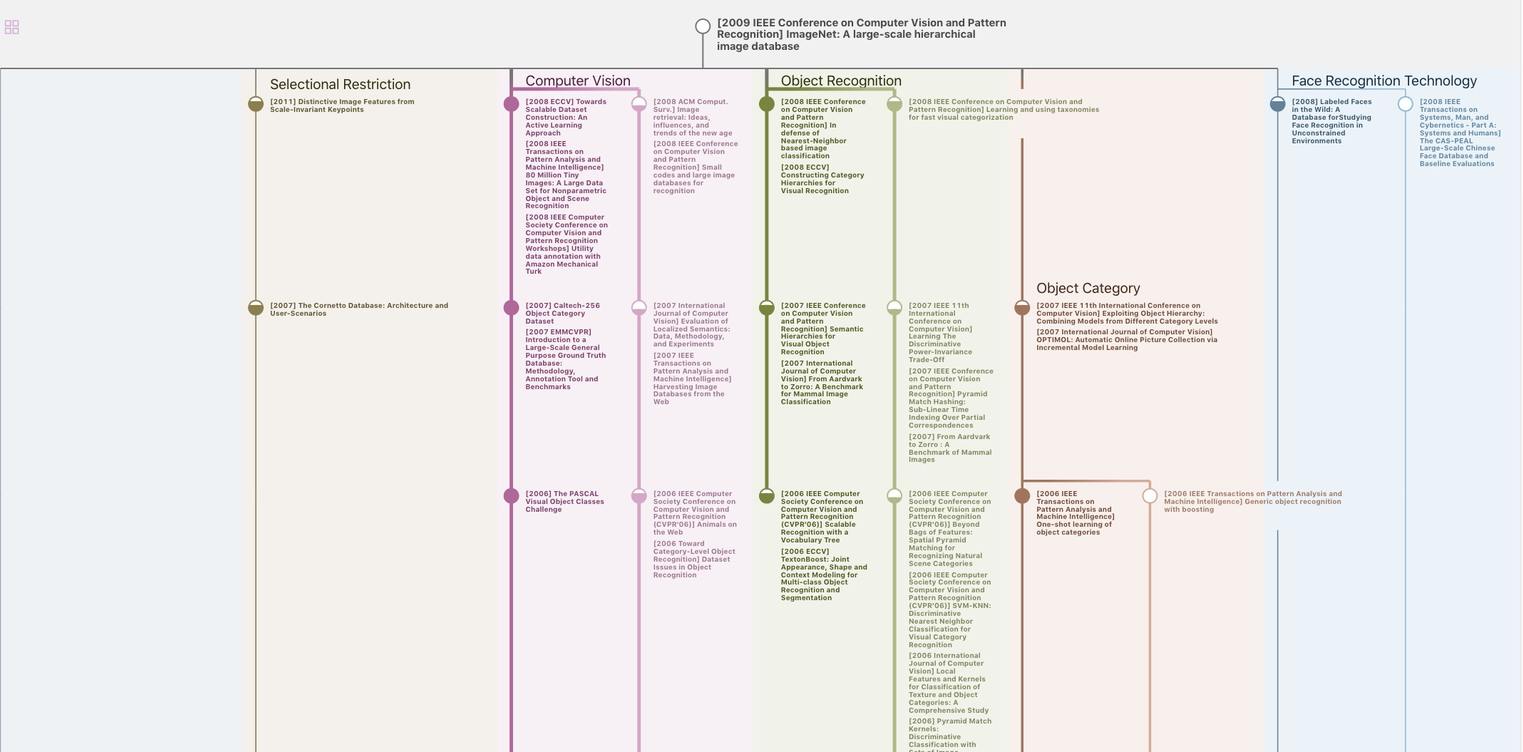
生成溯源树,研究论文发展脉络
Chat Paper
正在生成论文摘要