Multi-Institutional Development and Validation of a Radiomic Model to Predict Prostate Cancer Recurrence Following Radical Prostatectomy
JOURNAL OF CLINICAL MEDICINE(2023)
摘要
The use of multiparametric magnetic resonance imaging (mpMRI)-derived radiomics has the potential to offer noninvasive, imaging-based biomarkers for the identification of subvisual characteristics indicative of a poor oncologic outcome. The present study, therefore, seeks to develop, validate, and assess the performance of an MRI-derived radiomic model for the prediction of prostate cancer (PC) recurrence following radical prostatectomy (RP) with curative intent. mpMRI imaging was obtained from 251 patients who had undergone an RP for the treatment of localized prostate cancer across two institutions and three surgeons. All patients had a minimum of 2 years follow-up via prostate-specific antigen serum testing. Each prostate mpMRI was individually reviewed, and the prostate was delineated as a single slice (ROI) on axial T2 high-resolution image sets. A total of 924 radiomic features were extracted and tested for stability via intraclass correlation coefficient (ICC) following image normalization via histogram matching. Fourteen important and nonredundant features were found to be predictors of PC recurrence at a mean +/- SD of 3.2 +/- 2.2 years post-RP. Five-fold, ten-run cross-validation of the model containing these fourteen features yielded an area under the curve (AUC) of 0.89 +/- 0.04 in the training set (n = 225). In comparison, the University of California San Fransisco Cancer of the Prostate Risk Assessment score (UCSF-CAPRA) and Memorial Sloan Kettering Cancer Center (MSKCC) Pre-Radical prostatectomy nomograms yielded AUC of 0.66 +/- 0.05 and 0.67 +/- 0.05, respectively (p < 0.01). When the radiomic model was applied to the test set (n = 26), AUC was 0.78; sensitivity, specificity, positive predictive value, and negative predictive value were 60%, 86%, 52%, and 89%, respectively. Accuracy in predicting PC recurrence was 81%.
更多查看译文
关键词
prostate cancer,radiomics,recurrence,machine learning
AI 理解论文
溯源树
样例
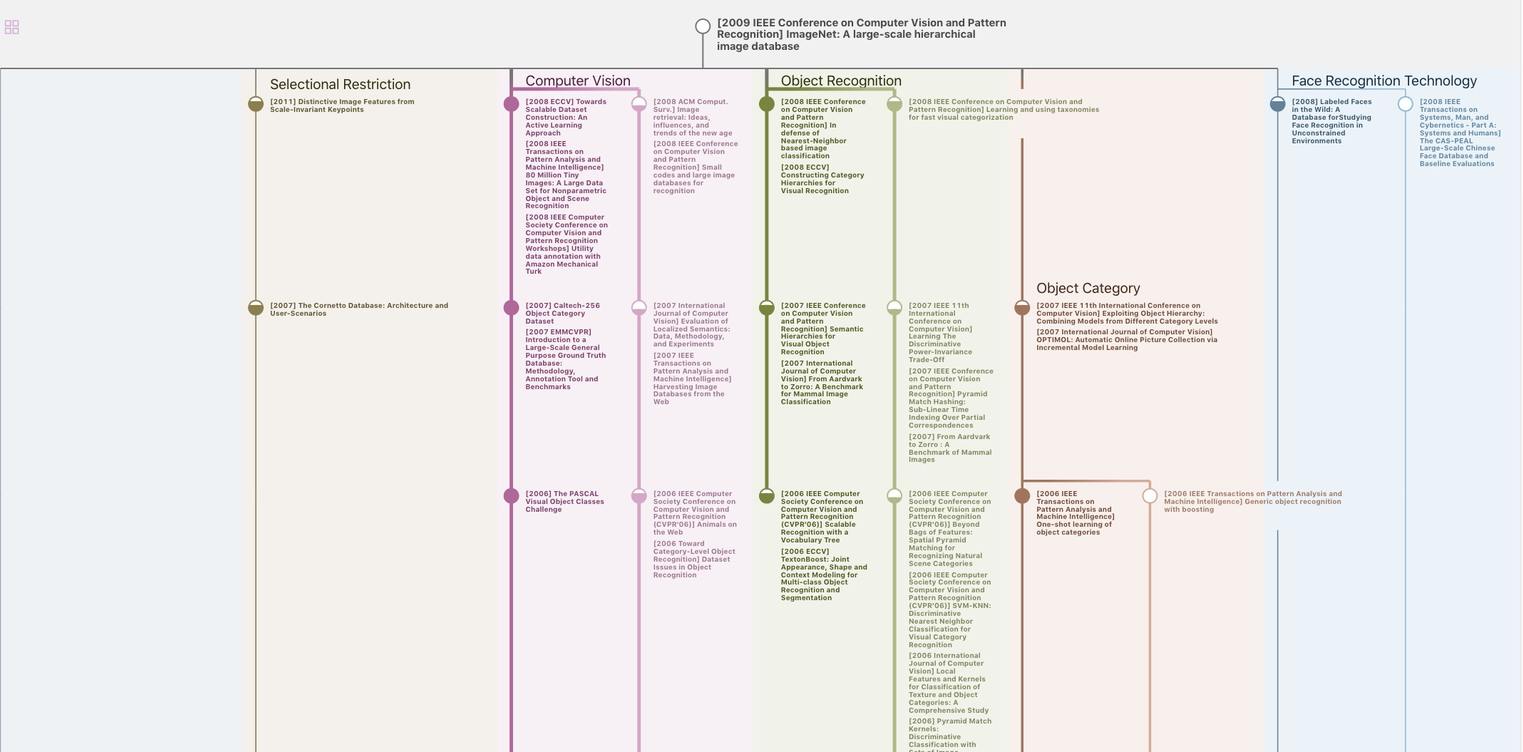
生成溯源树,研究论文发展脉络
Chat Paper
正在生成论文摘要