Progressive Feature Reconstruction and Fusion to Accelerate MRI Imaging: Exploring Insights across Low, Mid, and High-Order Dimensions
ELECTRONICS(2023)
摘要
Magnetic resonance imaging (MRI) faces ongoing challenges associated with prolonged acquisition times and susceptibility to motion artifacts. Compressed Sensing (CS) principles have emerged as a significant advancement, addressing these issues by subsampling k-space data points and enabling rapid imaging. Nevertheless, the recovery of intricate details from under-sampled data remains a complex endeavor. In this study, we introduce an innovative deep learning approach tailored to the restoration of high-fidelity MRI images from under-sampled k-space data. Our method employs a cascaded reconstruction strategy that progressively restores hierarchical features and fuses them to achieve the final reconstruction. This cascade encompasses low, intermediate, and high orders of reconstruction, which is followed by a return through intermediate and low orders. At distinct reconstruction stages, we introduce a novel reconstruction block to recapture diverse frequency information crucial for image reconstruction. The other core innovation of our proposal lies in a fusion algorithm that harmonizes results from various reconstruction tiers into the final MRI image. Our methodology is validated using two distinct datasets. Notably, our algorithm achieves impressive PSNR values of 32.60 and 31.02 at acceleration factors of 4x and 8x in the FastMRI dataset along with SSIM scores of 0.818 and 0.771, outperforming current state-of-the-art algorithms. Similarly, on the Calgary-Campinas dataset, our algorithm achieves even higher PSNR values, reaching 37.68 and 33.44, which is accompanied by substantial SSIM scores of 0.954 and 0.901. It is essential to highlight that our algorithm achieves these remarkable results with a relatively lower parameter count, underscoring its efficiency. Comparative analyses against analogous methods further emphasize the superior performance of our approach, providing robust evidence of its effectiveness.
更多查看译文
关键词
MRI reconstruction,compress sensing,deep learning,cascaded reconstruction,transformer-based fusion
AI 理解论文
溯源树
样例
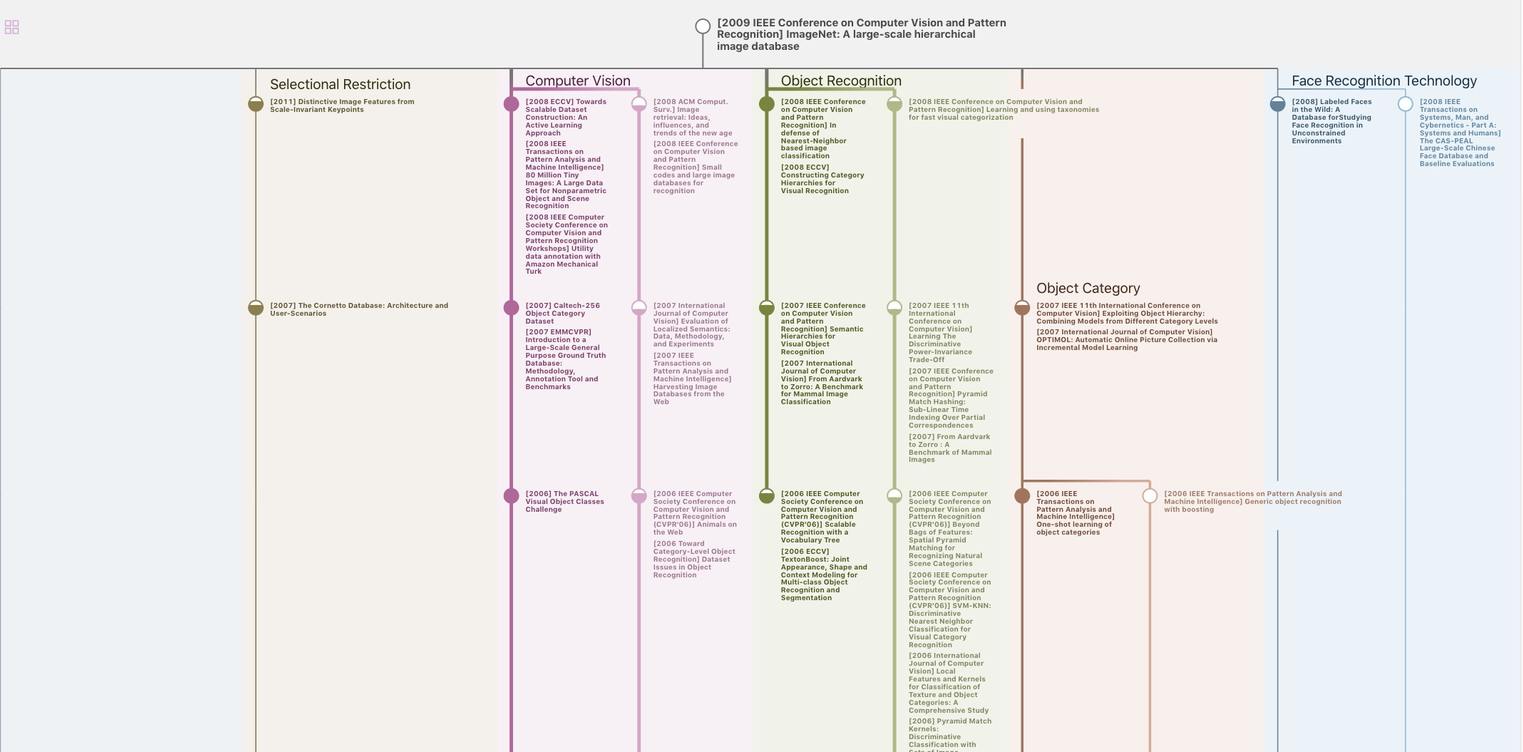
生成溯源树,研究论文发展脉络
Chat Paper
正在生成论文摘要