A Machine Anomalous Sound Detection Method Using the lMS Spectrogram and ES-MobileNetV3 Network
APPLIED SCIENCES-BASEL(2023)
摘要
Unsupervised anomalous sound detection by machines holds significant importance within the realm of industrial automation. Currently, the task of machine-based anomalous sound detection in complex industrial settings is faced with issues such as the challenge of extracting acoustic feature information and an insufficient feature extraction capability within the detection network. To address these challenges, this study proposes a machine anomalous sound detection method using the lMS spectrogram and ES-MobileNetV3 network. Firstly, the log-Mel spectrogram feature and the SincNet spectrogram feature are extracted from the raw wave, and the new lMS spectrogram is formed after fusion, serving as network input features. Subsequently, based on the MobileNetV3 network, an improved detection network, ES-MobileNetV3, is proposed in this paper. This network incorporates the Efficient Channel Attention module and the SoftPool method, which collectively reduces the loss of feature information and enhances the feature extraction capability of the detection network. Finally, experiments are conducted on the dataset provided by DCASE 2020 Task 2. Our proposed method attained an averaged area under the receiver operating characteristic curve (AUC) of 96.67% and an averaged partial AUC (pAUC) of 92.38%, demonstrating superior detection performance compared to other advanced methods.
更多查看译文
关键词
anomalous sound detection,acoustic features,convolutional neural network,attention mechanism
AI 理解论文
溯源树
样例
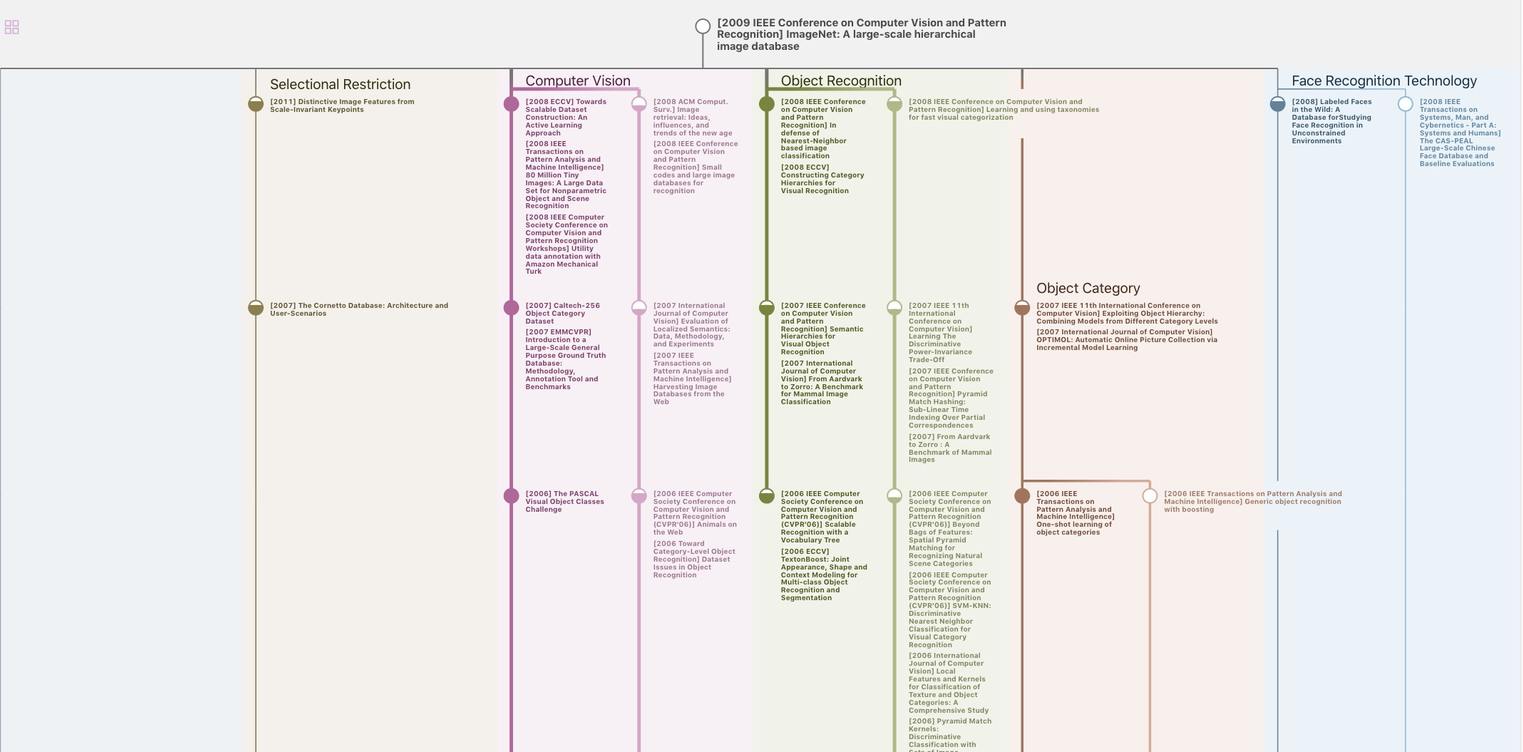
生成溯源树,研究论文发展脉络
Chat Paper
正在生成论文摘要