Speeding Up the Cocrystallization Process: Machine Learning-Combined Methods for the Prediction of Multicomponent Systems
CRYSTAL GROWTH & DESIGN(2023)
摘要
Pharmaceutical cocrystals are crystalline materials composed of at least two molecules, i.e., an active pharmaceutical ingredient (API) and a coformer, assembled by noncovalent forces. Cocrystallization is successfully applied to improve the physicochemical properties of APIs, such as solubility, dissolution profile, pharmacokinetics, and stability. However, choosing the ideal coformer is a challenging task in terms of time, efforts, and laboratory resources. Several computational tools and machine learning (ML) models have been proposed to mitigate this problem. However, the challenge of achieving a robust and generalizable predictive method is still open. In this study, we propose a new approach to quickly predict the formation of cocrystals, employing partial least squares-discriminant analysis, random forest, and neural networks. The models were based on the data sets of 13 structurally different APIs with both positive and negative cocrystallization outcomes. At the same time, the features were specially selected from a variety of molecular descriptors to explain the phenomenon of the cocrystallization. All of the proposed ML models showed a cross-validation accuracy higher than 83%. Furthermore, this approach was successfully applied to drive the cocrystallization experimental tests of 2-phenylpropionic acid, showcasing the high potential of the ML models in practice.
更多查看译文
关键词
cocrystallization process,prediction,systems,learning-combined
AI 理解论文
溯源树
样例
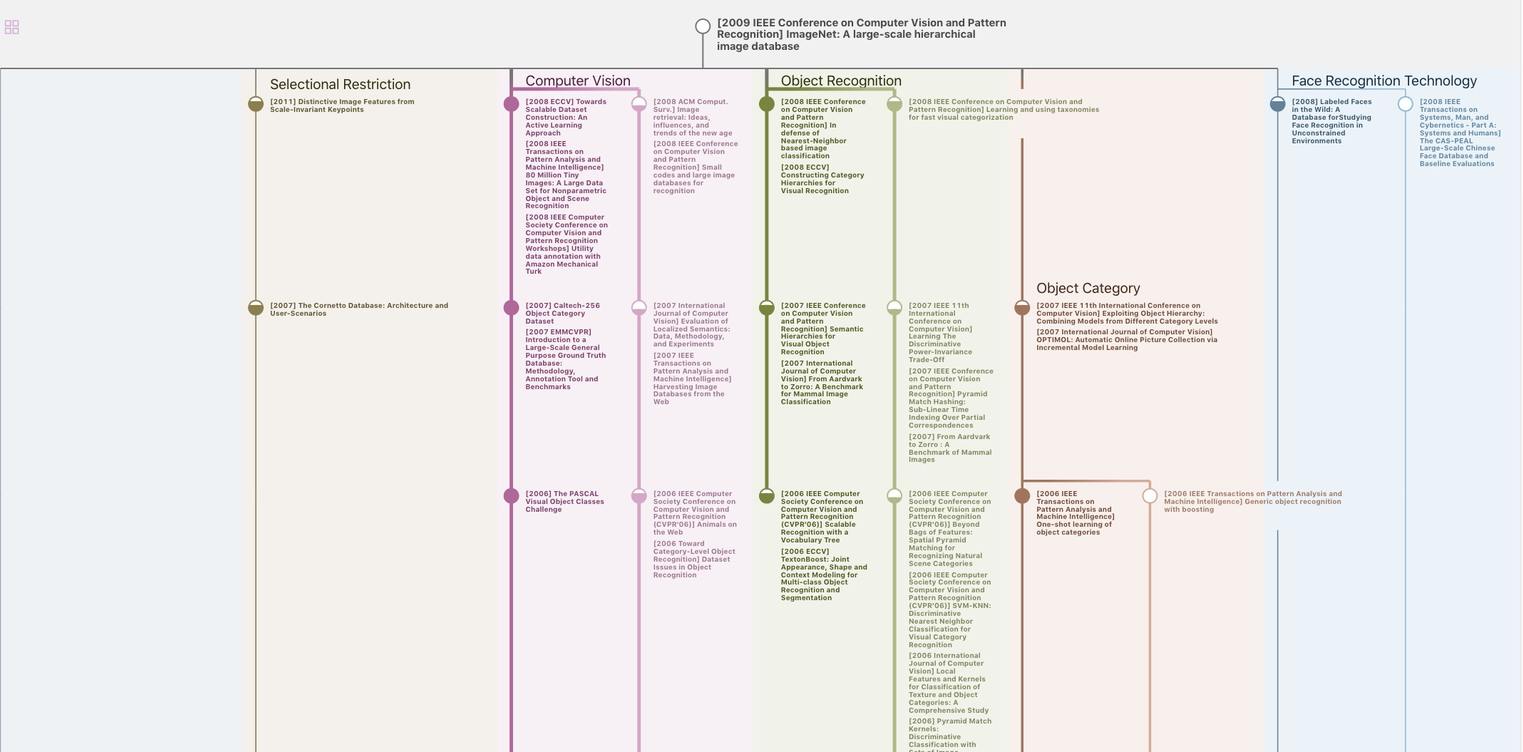
生成溯源树,研究论文发展脉络
Chat Paper
正在生成论文摘要