Printable Polar Silicone Elastomers for Healable Supercapacitive Strain Sensors
ADVANCED MATERIALS TECHNOLOGIES(2023)
摘要
Soft strain sensors with high sensitivity and the ability to recover from damages are required in the emerging field of self-healing soft robotics. Herein, printable supercapacitive strain sensors that can heal upon moderate heating (75 degrees C for 10 min) and exhibit a 30 times higher sensitivity than PDMS-based sensors are developed. For the sensor's core layer and electrode, a nitrile-functional polysiloxane that contains an active ionic initiator and can heal by siloxane equilibration at elevated temperatures is used. Supercapacitive strain sensors prepared from the elastomer are highly sensitive at low strains of 0-30%, enabled by the electric double-layer formation of the ionic initiator. After healing, the sensors exhibit nearly unaltered performance in tensile testing. Due to the thermoreversible nature of the elastomer network, patterned core layers with different microstructures can be printed by direct ink writing. The capacitive sensors based on these microstructured films reach a higher sensitivity and linearity than those based on unstructured films. Finally, the sensor is integrated into a soft robotic finger and the sensor's ability to determine the bending angle is validated by motion capture. This technology can provide new opportunities to equip soft robotic devices with custom-printed, healable strain sensors. Healable supercapacitive strain sensors with high sensitivity based on a functional silicone elastomer with polar side groups and an active ionic initiator are reported. Microstructured sensor core layers of the elastomer can be printed, which increases the sensitivity and linearity of the resulting sensors. This technology can be used to prepare custom-printed sensors for self-healing soft robots.image
更多查看译文
关键词
3D-printing, high-permittivity elastomers, polar polysiloxanes, self-healing, soft robotics, supercapacitive strain sensors
AI 理解论文
溯源树
样例
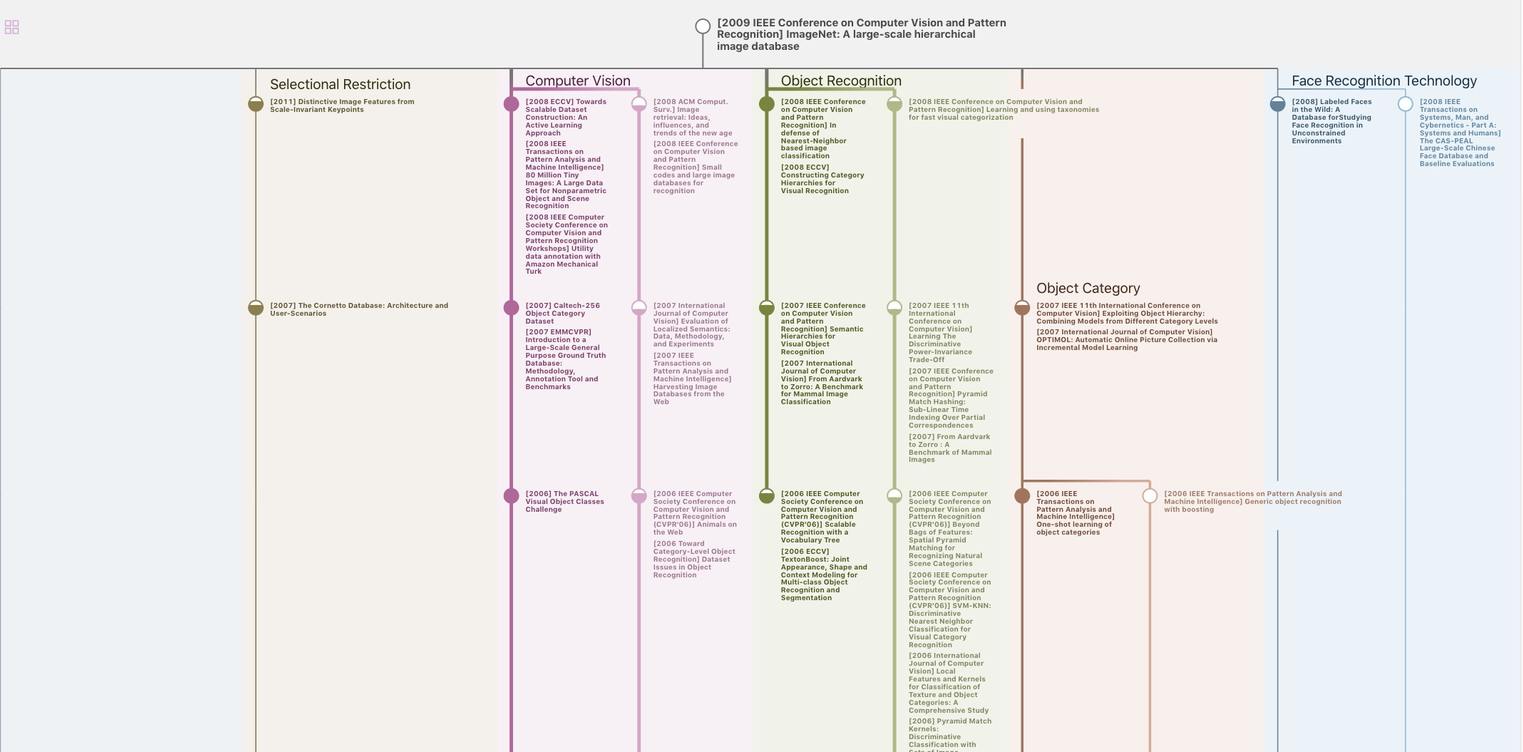
生成溯源树,研究论文发展脉络
Chat Paper
正在生成论文摘要