Optimized data-driven approach for remaining useful life prediction of Lithium-ion batteries based on sliding window and systematic sampling
JOURNAL OF ENERGY STORAGE(2023)
摘要
The prediction of remaining useful life (RUL) in lithium-ion batteries (LIB) serves as a critical health index for evaluating battery parameters, including efficiency, robustness, and accuracy. Existing RUL prediction techniques encounter challenges related to data extraction based on battery parameters and data samples, as well as the selection of appropriate model hyperparameters to achieve accurate outcomes. To address these issues, this article presents a novel framework for RUL prediction using a hybrid optimized data-driven approach, which combines a cascaded forward neural network (CFNN) with the innovative jellyfish optimization (JFO) technique. Initially, a comprehensive 46-data feature-based framework is developed for training the proposed hybrid JFO-CFNN model, employing a mathematical systematic sampling (SS) method. The SS method facilitates the selection of 15 relevant data features, from the LIB parameters such as temperature, current, and voltage, from each charging cycle. Additionally, the developed framework mitigates the capacity regeneration effect present with the capacity degradation profile by employing the overlapping sliding window (OSW) technique. Moreover, the CFNN model parameters, such as hidden neurons and learning rate, are optimized using the newly introduced meta-heuristic JFO technique. To validate the performance of the proposed hybrid JFO-CFNN model, comparisons are made with other models such as the JFO-optimized artificial neural network (ANN) and deep neural network (DNN) model as well as with another battery dataset obtained from the Massachusetts Institute of Technology (MIT) Stanford battery database. The outcomes based on the NASA battery suggested the average RMSE of the proposed JFO-CFNN model below 0.1 and 0.15 for NASA and MIT Stanford battery datasets. The experimental results demonstrate the high prediction accuracy and applicability of the proposed hybrid JFO-CFNN model.
更多查看译文
关键词
Optimization technique,Lithium-ion batteries,Data-driven method,Remaining useful life (RUL),Systematic sampling (SS),Overlapping sliding window (OSW)
AI 理解论文
溯源树
样例
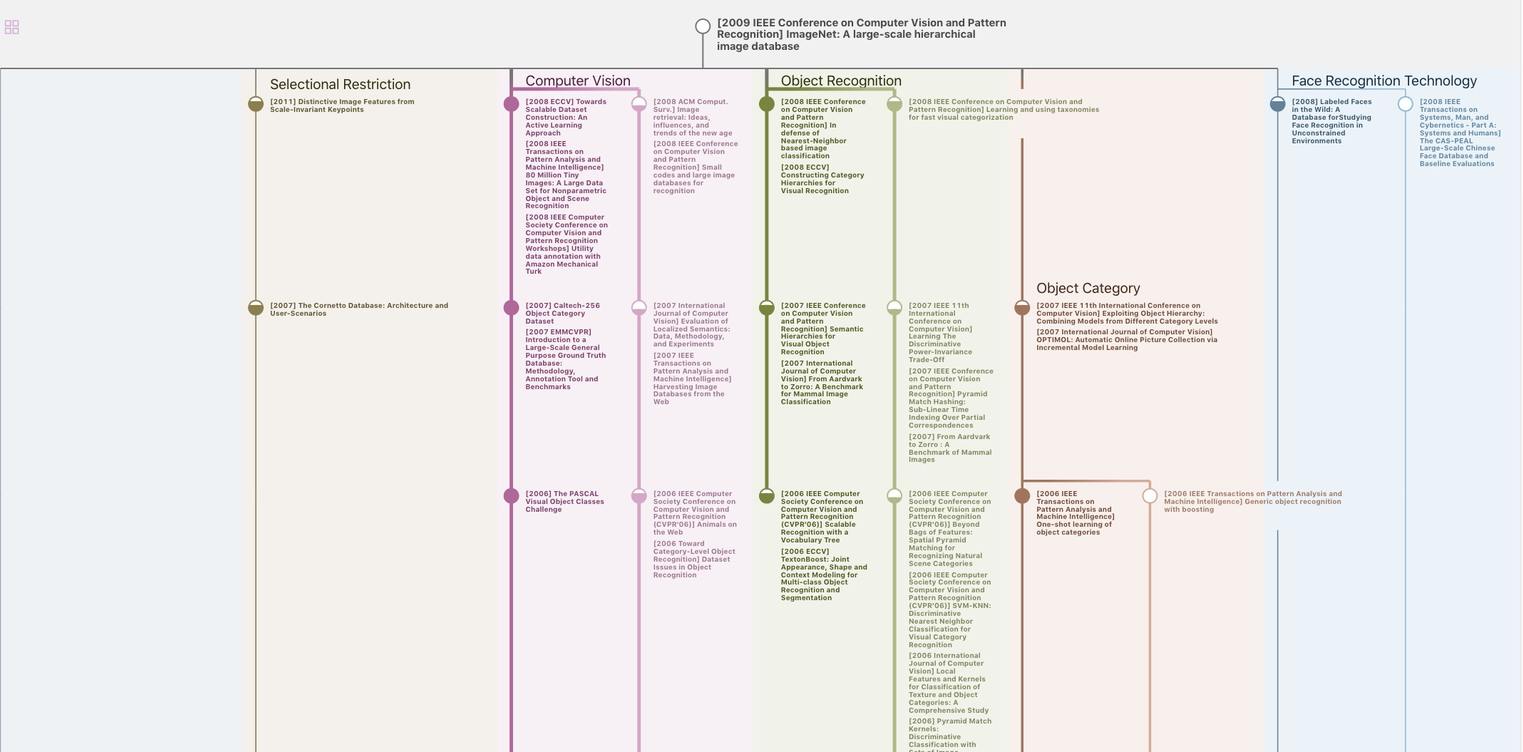
生成溯源树,研究论文发展脉络
Chat Paper
正在生成论文摘要