Deep-Net: Fine-Tuned Deep Neural Network Multi-Features Fusion for Brain Tumor Recognition
CMC-COMPUTERS MATERIALS & CONTINUA(2023)
摘要
Manual diagnosis of brain tumors using magnetic resonance images (MRI) is a hectic process and time-consuming. Also, it always requires an expert person for the diagnosis. Therefore, many computer-controlled methods for diagnosing and classifying brain tumors have been introduced in the literature. This paper proposes a novel multimodal brain tumor classification framework based on two-way deep learning feature extraction and a hybrid feature optimization algorithm. NasNet-Mobile, a pre-trained deep learning model, has been fine-tuned and two-way trained on original and enhanced MRI images. The haze-convolutional neural network (haze-CNN) approach is developed and employed on the original images for contrast enhancement. Next, transfer learning (TL) is utilized for training two-way fine-tuned models and extracting feature vectors from the global average pooling layer. Then, using a multiset canonical correlation analysis (CCA) method, features of both deep learning models are fused into a single feature matrix-this technique aims to enhance the information in terms of features for better classification. Although the information was increased, computational time also jumped. This issue is resolved using a hybrid feature optimization algorithm that chooses the best classification features. The experiments were done on two publicly available datasets-BraTs2018 and BraTs2019-and yielded accuracy rates of 94.8% and 95.7%, respectively. The proposed method is compared with several recent studies and outperformed in accuracy. In addition, we analyze the performance of each middle step of the proposed approach and find the selection technique strengthens the proposed framework.
更多查看译文
关键词
brain tumor recognition,deep-net neural network,fusion,fine-tuned,multi-features
AI 理解论文
溯源树
样例
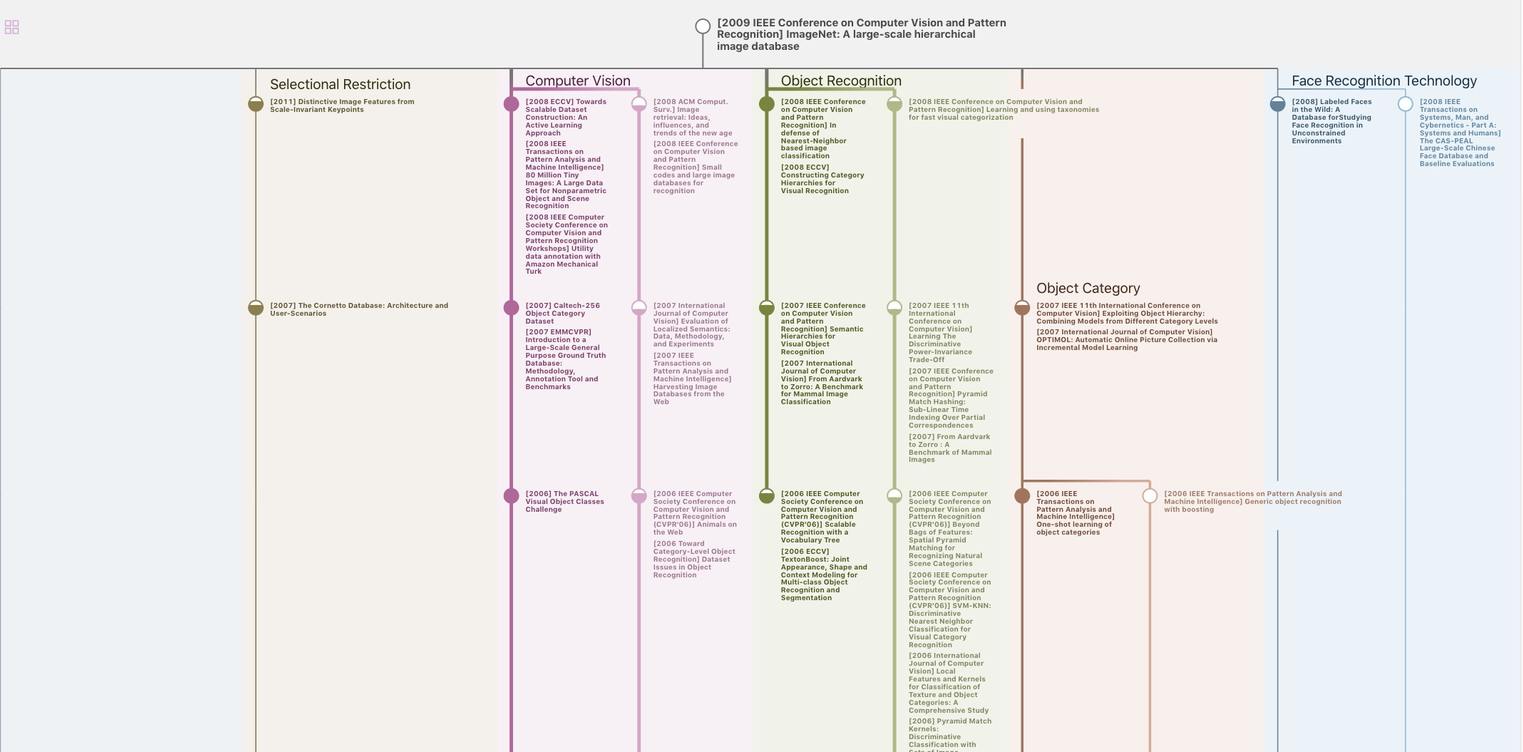
生成溯源树,研究论文发展脉络
Chat Paper
正在生成论文摘要