Adaptive Horizon Seeking for Generalized Predictive Control via Deep Reinforcement Learning With Application to DC/DC Converters
IEEE TRANSACTIONS ON CIRCUITS AND SYSTEMS I-REGULAR PAPERS(2023)
摘要
For enhancing the capacity to optimize the control performance of the generalized predictive control (GPC) method, this paper proposes an adaptive horizon mechanism design methodology based on deep reinforcement learning (DRL). To handle the systems with the presence of mismatched disturbances, a baseline and secure environment for DRL training is established by constructing an offset-free GPC design framework. Furthermore, the proposed method incorporates a multi-objective reward function design into the twin-delayed deep deterministic policy gradient (TD3) algorithm, which explicitly considers multiple comprehensive performance indexes. This enables the ability to attain the desired level of control precision and meet diverse control requirements across various operating conditions. Besides, it attains the capability to adapt to various system parameters effectively. The proposed method is validated through a numerical simulation of a three-order numerical system and an application to a DC/DC boost converter feeding constant power loads (CPLs). Simulation and experimental comparison results with other controllers demonstrate the efficacy and performance improvements.
更多查看译文
关键词
Generalized predictive control,deep reinforcement learning,matched and mismatched disturbance,adaptive horizon,power electronics
AI 理解论文
溯源树
样例
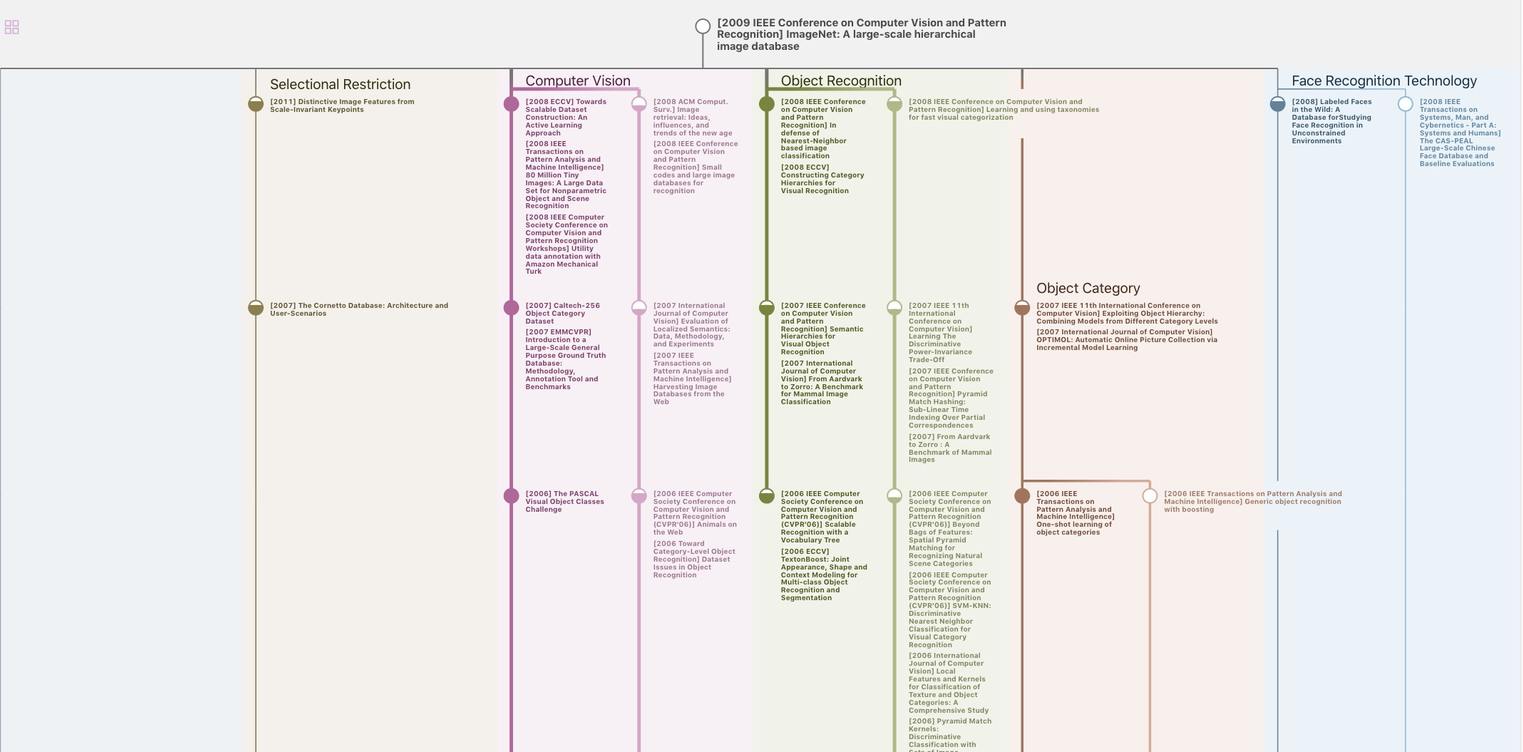
生成溯源树,研究论文发展脉络
Chat Paper
正在生成论文摘要