Radar-based Human Activity Recognition Using Denoising Techniques to Enhance Classification Accuracy
IET RADAR SONAR AND NAVIGATION(2024)
摘要
Radar-based human activity recognition is considered as a competitive solution for the elderly care health monitoring problem, compared to alternative techniques such as cameras and wearable devices. However, raw radar signals are often contaminated with noise, clutter, and other artifacts that significantly impact recognition performance, which highlights the importance of prepossessing techniques that enhance radar data quality and improve classification model accuracy. In this study, two different human activity classification models incorporated with pre-processing techniques have been proposed. The authors introduce wavelet denoising methods into a cyclostationarity-based classification model, resulting in a substantial improvement in classification accuracy. To address the limitations of conventional pre-processing techniques, a deep neural network model called Double Phase Cascaded Denoising and Classification Network (DPDCNet) is proposed, which performs end-to-end signal-level classification and achieves state-of-the-art accuracy. The proposed models significantly reduce false detections and would enable robust activity monitoring for older individuals with radar signals, thereby bringing the system closer to a practical implementation for deployment. Radar-based Human activity recognition using denoising techniques is used to enhance classification accuracy. Two methods are evaluated; cyclostationarity-based with wavelet-based denoising and a deep neural network model called Double Phase Cascaded Denoising and Classifi-cation Network (DPDCNet). The proposed models significantly reduce false detections and would enable robust activity monitoring for older individuals with radar signals, thereby bringing the system closer to a practical implementation for deployment.image
更多查看译文
关键词
radar,signal classification,signal denoising
AI 理解论文
溯源树
样例
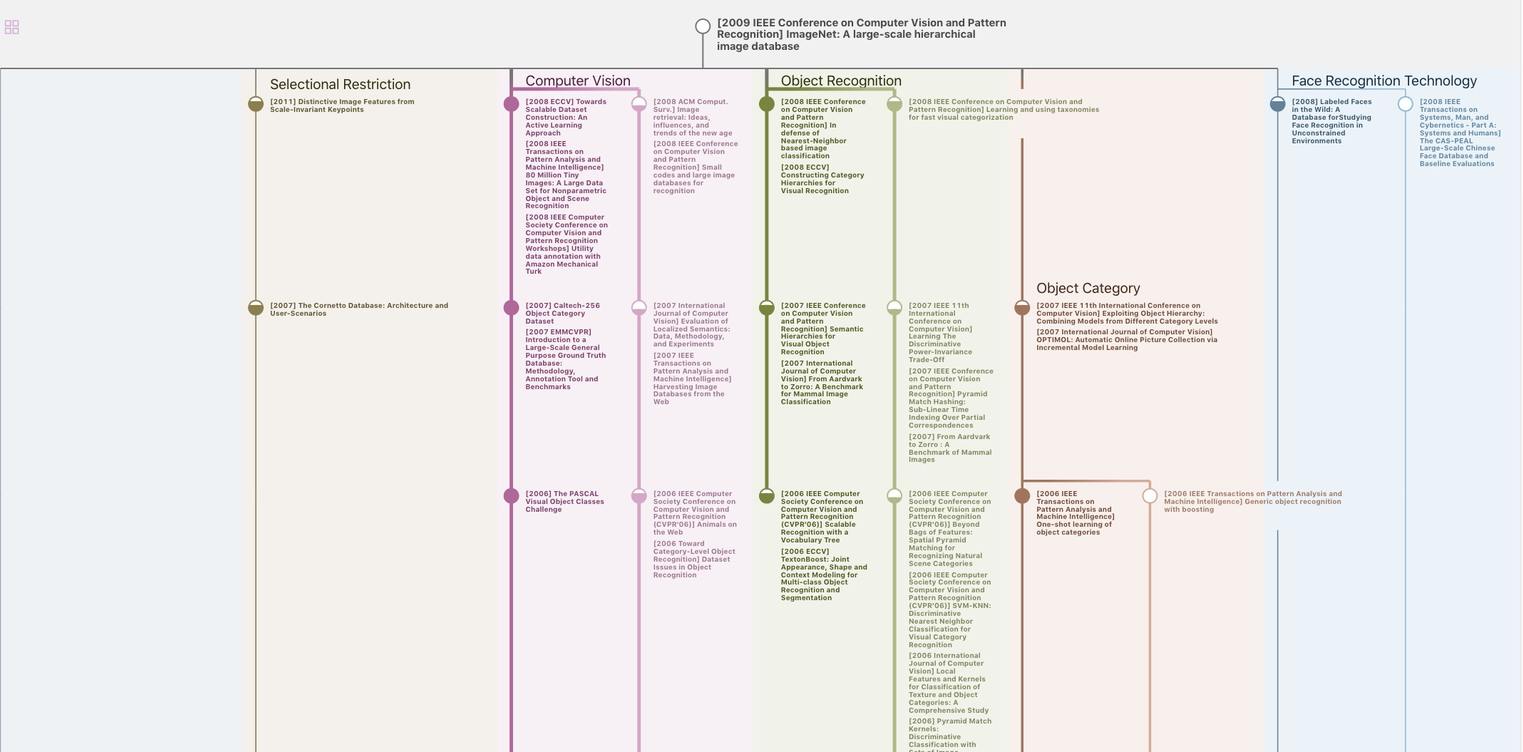
生成溯源树,研究论文发展脉络
Chat Paper
正在生成论文摘要