Analysis of abnormal data in sensor networks based on improved LSTM in the Internet of Things environment
INTERNATIONAL JOURNAL OF COMMUNICATION SYSTEMS(2024)
摘要
The proposed method addresses the challenge of online detection of high-dimensional data in the IoT environment by introducing an anomaly data analysis technique based on improved LSTM. The method involves normalizing both normal and abnormal data using the correlation between multidimensional data and transforming them into gray image representations for input. Additionally, an enhanced abnormal data detection approach is presented through the construction of two parallel network models: a "two-layer model" and a "single-layer model." This approach aims to improve stability in modeling normal data and enhance the detection capability for abnormal data. The proposed method was evaluated on the Human Activity Recognition (HAR) dataset, which consists of 561 dimensions. The experimental results showcased the effectiveness of this method, achieving a detection rate of 94.12% and a recall rate of 95.21%. These rates surpassed the performance of existing techniques in the field of abnormal data detection. Consequently, this method has demonstrated significant advancements and offers improved system performance when compared to current methods. Schematic diagram of improved LSTM model. This improved LSTM model constructs two networks of parallel processing structures: one is a "two-layer model" network, and the other is a "single-layer model" network.image
更多查看译文
关键词
abnormal data,deep learning,Internet of Things (IoT),parallel network,power grid business data,wireless sensor network
AI 理解论文
溯源树
样例
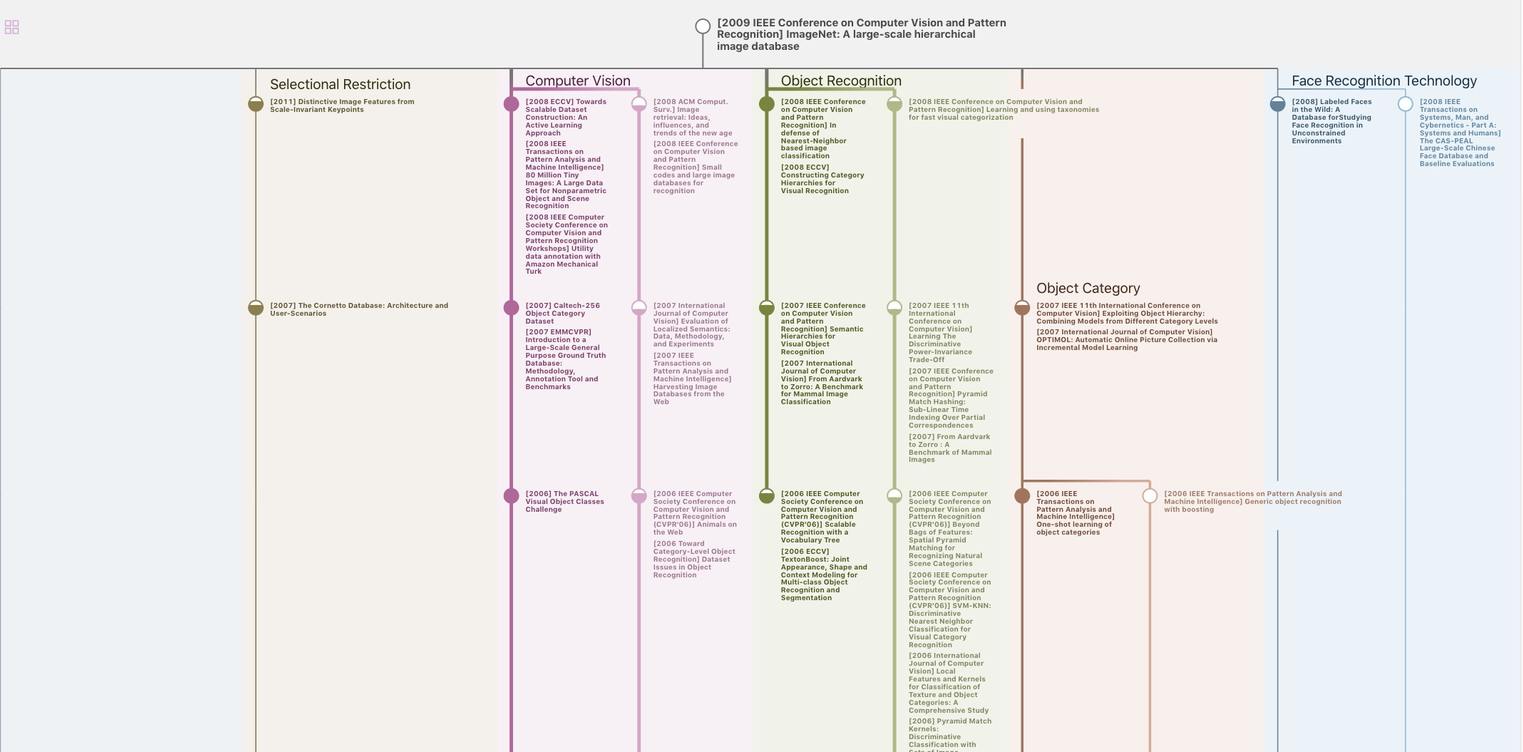
生成溯源树,研究论文发展脉络
Chat Paper
正在生成论文摘要