The Role of Social Capital, Resilience, and Network Complexity in Attaining Supply Chain Sustainability
BUSINESS STRATEGY AND THE ENVIRONMENT(2024)
Univ Technol Sydney | Torrens Univ | CQUniv | Curtin Univ
Abstract
Supply chain social capitals argued to be the driver for attaining sustainability along the supply chain. However, the role of network complexity and supply chain resilience to transform supply chain social capitals into sustainability remains poorly understood. We draw from social capital theory to argue that supply chain resilience is the mechanism to curb negative effects of network complexity to transform supply chain social capital into supply chain sustainability. Hypotheses were tested employing structural equation modeling technique on a sample ( n = 274) of Bangladeshi apparel suppliers, and supplemented by the fuzzy set qualitative comparative analysis to identify all plausible underlying causal configurations. Our results suggest that supply chain social capitals positively influence supply chain sustainability both directly and indirectly via supply chain resilience. Contrary to our hypothesis, we found that network complexity in the presence of supply chain resilience positively influences social capitals to bolster supply chain sustainability. Findings of this research imply that supply chain resilience is a crucial conduit that facilitates leveraging supply chain social capitals as well as withstanding challenges that emerge from network complexity to embolden supply chain sustainability. Findings of this research provide novel insights to existing literature by exploring the complex dynamisms and reaffirming the interrelationships among the four vital constructs of supply chain literature. Our finding can help supply chain managers to improve resilience and sustainability.
MoreTranslated text
Key words
fsQCA,network complexity,resilience,social capital,supply chain,sustainability
求助PDF
上传PDF
View via Publisher
AI Read Science
AI Summary
AI Summary is the key point extracted automatically understanding the full text of the paper, including the background, methods, results, conclusions, icons and other key content, so that you can get the outline of the paper at a glance.
Example
Background
Key content
Introduction
Methods
Results
Related work
Fund
Key content
- Pretraining has recently greatly promoted the development of natural language processing (NLP)
- We show that M6 outperforms the baselines in multimodal downstream tasks, and the large M6 with 10 parameters can reach a better performance
- We propose a method called M6 that is able to process information of multiple modalities and perform both single-modal and cross-modal understanding and generation
- The model is scaled to large model with 10 billion parameters with sophisticated deployment, and the 10 -parameter M6-large is the largest pretrained model in Chinese
- Experimental results show that our proposed M6 outperforms the baseline in a number of downstream tasks concerning both single modality and multiple modalities We will continue the pretraining of extremely large models by increasing data to explore the limit of its performance
Upload PDF to Generate Summary
Must-Reading Tree
Example
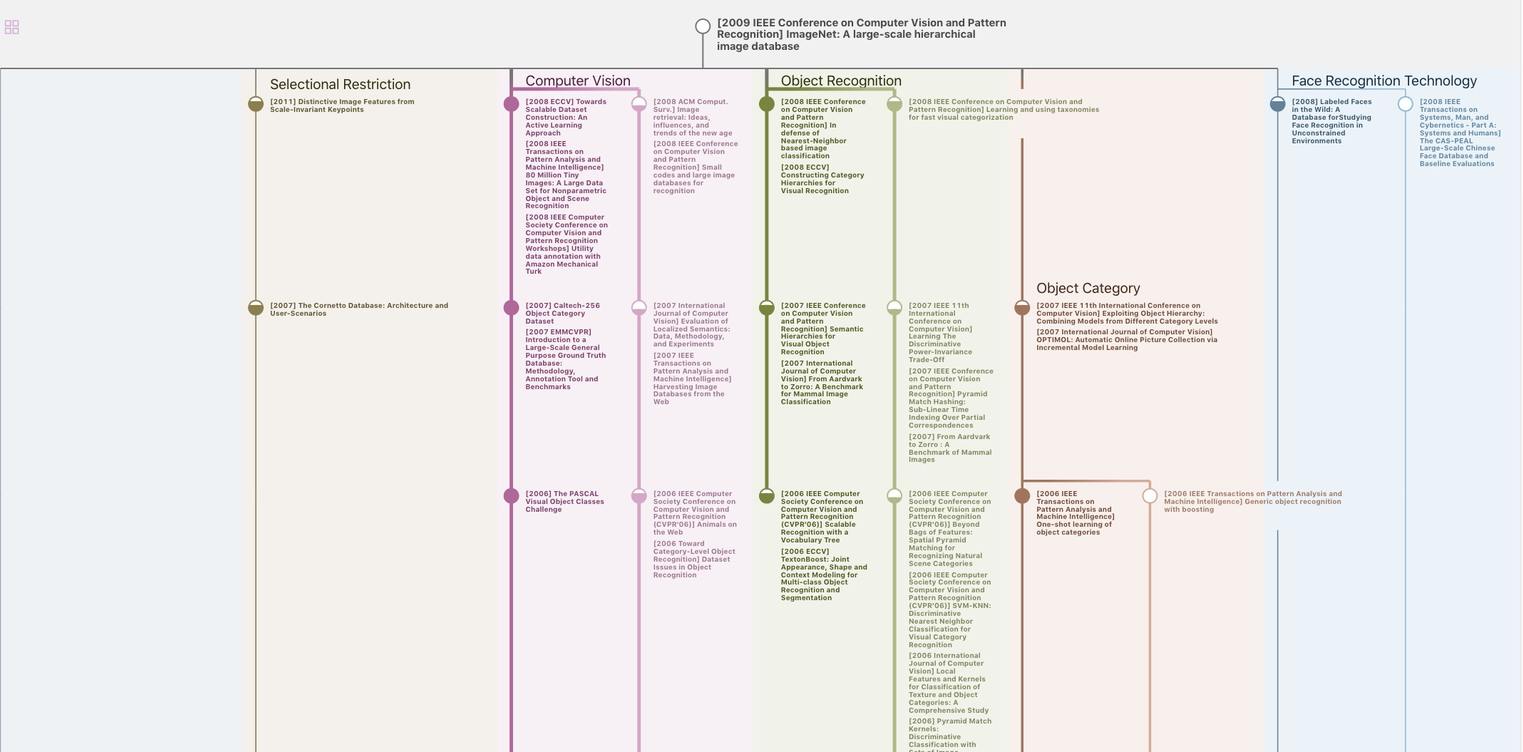
Generate MRT to find the research sequence of this paper
Related Papers
2008
被引用940 | 浏览
2009
被引用1294 | 浏览
2009
被引用113 | 浏览
2003
被引用82331 | 浏览
2015
被引用129 | 浏览
2015
被引用146 | 浏览
2012
被引用343 | 浏览
2016
被引用203 | 浏览
2018
被引用53 | 浏览
2018
被引用52 | 浏览
2018
被引用250 | 浏览
2019
被引用146 | 浏览
2019
被引用136 | 浏览
2022
被引用20 | 浏览
2023
被引用9 | 浏览
Data Disclaimer
The page data are from open Internet sources, cooperative publishers and automatic analysis results through AI technology. We do not make any commitments and guarantees for the validity, accuracy, correctness, reliability, completeness and timeliness of the page data. If you have any questions, please contact us by email: report@aminer.cn
Chat Paper
GPU is busy, summary generation fails
Rerequest