Spatiotemporal Fracture Data Inference in Sparse Mobile Crowdsensing: A Graph-and Attention-Based Approach
IEEE-ACM TRANSACTIONS ON NETWORKING(2024)
摘要
Mobile Crowdsensing (MCS) is a sensing paradigm that enables large-scale smart city applications, such as environmental sensing and traffic monitoring. However, traditional MCS often suffers from performance degradation due to the limited spatiotemporal coverage of collected data. In this context, Sparse MCS has been proposed, which utilizes data inference algorithms to recover full data from sparse data collected by users. However, existing Sparse MCS approaches often overlook spatiotemporal fractures, where no data is observed either for a sensing subarea across all sensing time slots (temporal fracture), or for a sensing time slot in all sensing subarea (spatial fracture). Such spatiotemporal fractures pose great challenges to the data inference algorithms, as it is difficult to capture the complex spatiotemporal correlations of the sensing data from very limited observations. To address this issue, we propose a Graph-and Attention-based Matrix Completion (GAMC) method for the spatiotemporal fracture data inference problem in Sparse MCS. Specifically, we first pre-fill the general missing values using the classical Matrix Factorization (MF) technique. Then, we propose a neural network architecture based on Graph Attention Networks (GAT) and Transformer to capture complex spatiotemporal dependencies in the sensing data. Finally, we recover the complete data with a projection layer. We conduct extensive experiments on three real-world urban sensing datasets. The experimental results show the effectiveness of the proposed method.
更多查看译文
关键词
Mobile crowdsensing,spatiotemporal fracturedata inference,graph attention networks,transformer
AI 理解论文
溯源树
样例
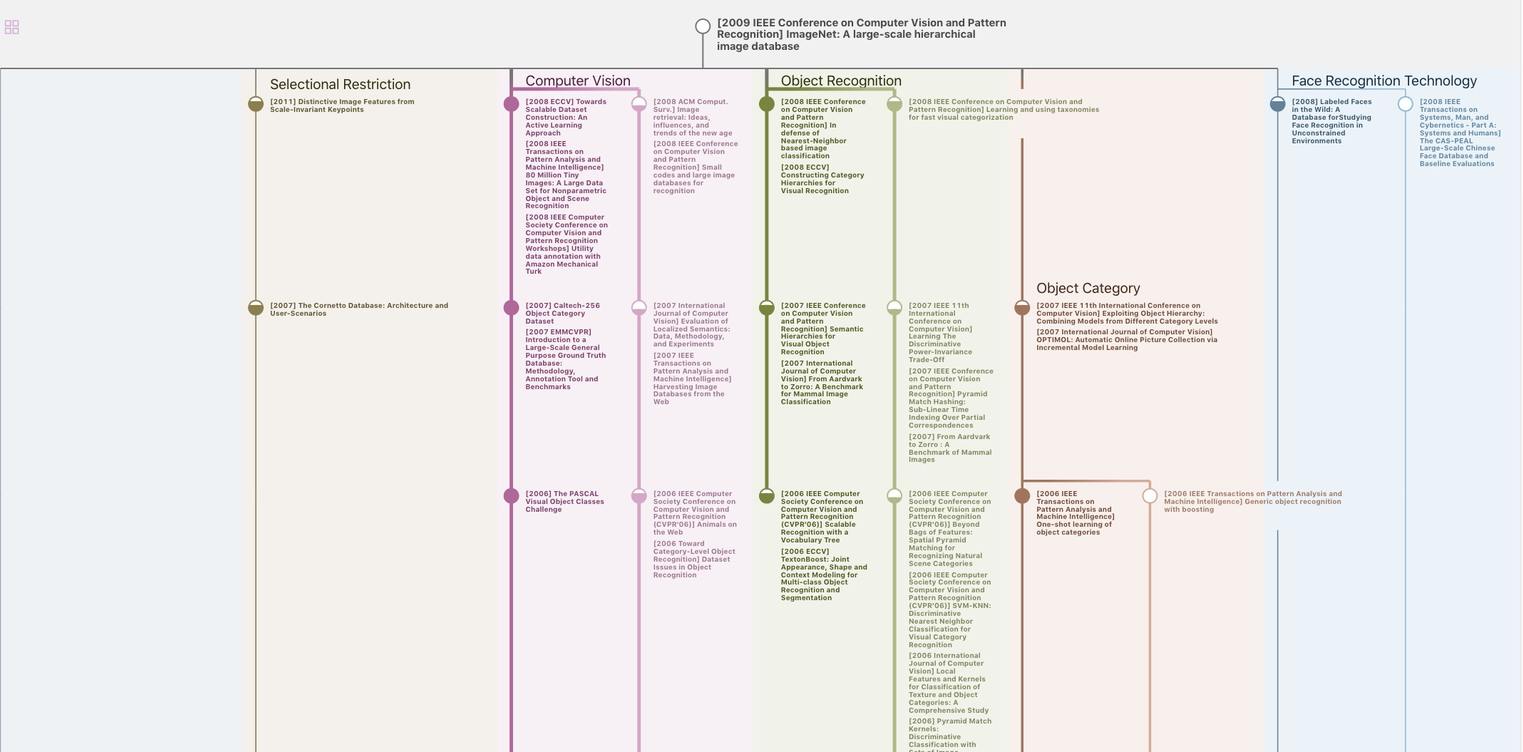
生成溯源树,研究论文发展脉络
Chat Paper
正在生成论文摘要