Using an artificial intelligence model to detect and localize visible clinically significant prostate cancer in prostate magnetic resonance imaging: a multicenter external validation study
QUANTITATIVE IMAGING IN MEDICINE AND SURGERY(2023)
摘要
Background: An increasing number of patients with suspected clinically significant prostate cancer (csPCa) are undergoing prostate multiparametric magnetic resonance imaging (mpMRI). The role of artificial intelligence (AI) algorithms in interpreting prostate mpMRI needs to be tested with multicenter external
data. This study aimed to investigate the diagnostic efficacy of an AI model in detecting and localizing visible csPCa on mpMRI a multicenter external data set.Methods: The data of 2,105 patients suspected of having prostate cancer from four hospitals were retrospectively collected to develop an AI model to detect and localize suspicious csPCa. The lesions were annotated based on pathology records by two radiologists. Diffusion-weighted imaging (DWI) and apparent diffusion coefficient (ADC) values were used as the input for the three-dimensional U-Net framework. Subsequently, the model was validated using an external data set comprising the data of 557 patients from three hospitals. Sensitivity, specificity, and accuracy were employed to evaluate the diagnostic efficacy of the model.
Results: At the lesion level, the model had a sensitivity of 0.654. At the overall sextant level, the model had a sensitivity, specificity, and accuracy of 0.846, 0.884, and 0.874, respectively. At the patient level, the model had a sensitivity, specificity, and accuracy of 0.943, 0.776, and 0.849, respectively. The AI-predicted accuracy for the csPCa patients (231/245, 0.943) was significantly higher than that for the non-csPCa patients (242/312, 0.776) (P<0.001). The lesion number and tumor volume were greater in the correctly diagnosed patients than the incorrectly diagnosed patients (both P<0.001). Among the positive patients, those with lower average ADC values had a higher rate of correct diagnosis than those with higher average ADC values (P=0.01).Conclusions: The AI model exhibited acceptable accuracy in detecting and localizing visible csPCa at the patient and sextant levels. However, further improvements need to be made to enhance the sensitivity of the model at the lesion level.
更多data. This study aimed to investigate the diagnostic efficacy of an AI model in detecting and localizing visible csPCa on mpMRI a multicenter external data set.Methods: The data of 2,105 patients suspected of having prostate cancer from four hospitals were retrospectively collected to develop an AI model to detect and localize suspicious csPCa. The lesions were annotated based on pathology records by two radiologists. Diffusion-weighted imaging (DWI) and apparent diffusion coefficient (ADC) values were used as the input for the three-dimensional U-Net framework. Subsequently, the model was validated using an external data set comprising the data of 557 patients from three hospitals. Sensitivity, specificity, and accuracy were employed to evaluate the diagnostic efficacy of the model.
Results: At the lesion level, the model had a sensitivity of 0.654. At the overall sextant level, the model had a sensitivity, specificity, and accuracy of 0.846, 0.884, and 0.874, respectively. At the patient level, the model had a sensitivity, specificity, and accuracy of 0.943, 0.776, and 0.849, respectively. The AI-predicted accuracy for the csPCa patients (231/245, 0.943) was significantly higher than that for the non-csPCa patients (242/312, 0.776) (P<0.001). The lesion number and tumor volume were greater in the correctly diagnosed patients than the incorrectly diagnosed patients (both P<0.001). Among the positive patients, those with lower average ADC values had a higher rate of correct diagnosis than those with higher average ADC values (P=0.01).Conclusions: The AI model exhibited acceptable accuracy in detecting and localizing visible csPCa at the patient and sextant levels. However, further improvements need to be made to enhance the sensitivity of the model at the lesion level.
查看译文
关键词
Clinically significant prostate cancer (csPCa),artificial intelligence (AI),magnetic resonance imaging (MRI)
AI 理解论文
溯源树
样例
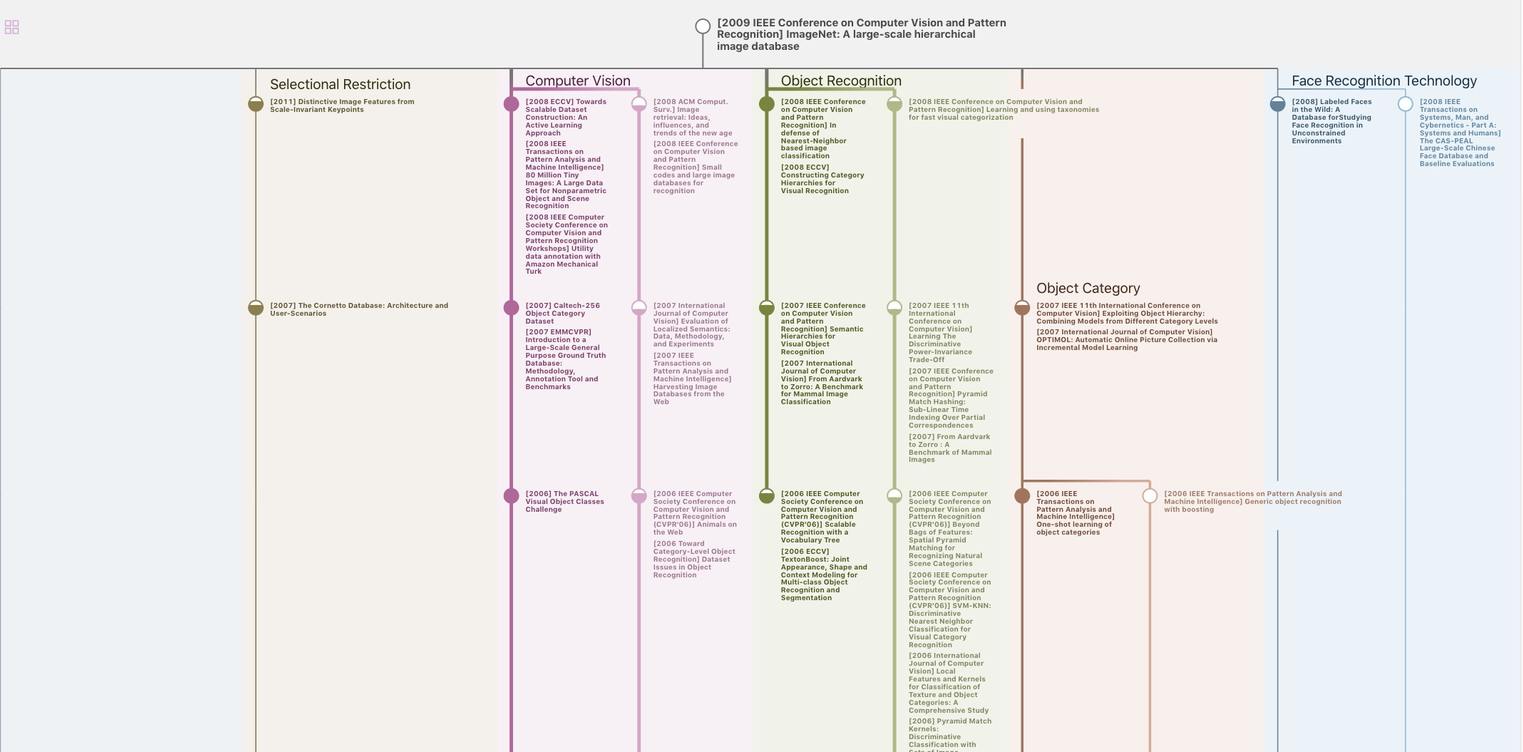
生成溯源树,研究论文发展脉络
Chat Paper
正在生成论文摘要