Anomalous variable-length subsequence detection in time series: mathematical formulation and a novel evolutionary algorithm based on clustering and swarm intelligence
APPLIED INTELLIGENCE(2023)
摘要
Variable-length anomalous subsequence detection in time series has many important applications in the real world, yet the methods presented in existing studies are computationally expensive, as the detection techniques are mostly brute-force approaches. In this work, we formalize the detection problem into a subsequence segmentation problem (SSP) optimization task, in which the time series is segmented by a set of cutting points into subsequences with minimized total distances to the representative motif. The anomalous subsequences can then be accurately located by reducing the dissimilarity among all subsequences, and this technique, when compared to existing techniques, can reduce the number of comparisons required for search. We further introduce a new clustering-based and swarm intelligence-based evolutionary algorithm (CBSI) in this work to solve the highly complex SSP efficiently. The proposed method balances the scopes of exploration and exploitation under a local-global search strategy. The CBSI clusters the solutions in the search space into groups, allowing frequent information sharing among solutions in the same cluster for their exploitation within their own search spaces. Furthermore, the best local solutions are promoted by the global-search strategy to explore the remaining search regions. Through a comparison with existing state-of-the-art techniques in solving both synthetic and real-world problems, we show that any optimization methods under our proposed SSP bring significant computational savings and comparable searching accuracy compared to existing techniques for the detection task. Our proposed CBSI also has the highest searching capability compared to existing and related optimization methods. The experimental results also highlight the scalability of our study to longer time series, larger anomaly sizes and wider search ranges.
更多查看译文
关键词
Anomaly detection, Data mining, Anomalous subsequence discovery, Evolutionary computation, Knowledge discovery, Time series analysis
AI 理解论文
溯源树
样例
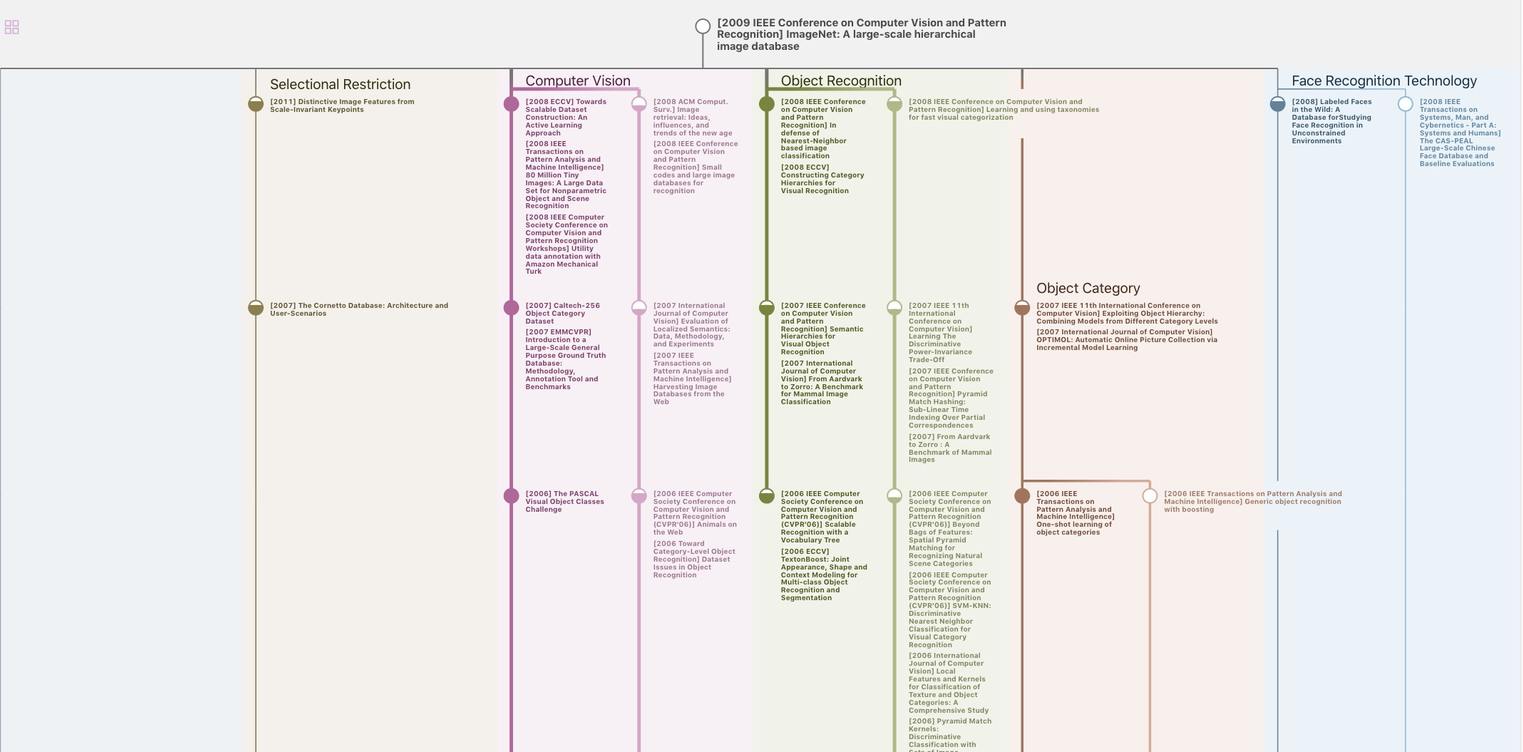
生成溯源树,研究论文发展脉络
Chat Paper
正在生成论文摘要