Bayesian estimation of parameters and uncertainty of a 3-D dipping fault using gravity anomalies
GEOPHYSICAL JOURNAL INTERNATIONAL(2023)
摘要
Many significant geophysical anomalies are generated by fault systems, and effectively estimating fault parameters is a key step to understand the characteristics of these field sources. In general, the 2-D dipping fault is frequently utilized to simplify the representation of fault geometry. However, this approach could lead to larger errors when comparing realistic scenarios with simplifications. In this study, we use a parametrized 3-D dipping model to represent fault systems and compute gravity anomalies using an analytic method in the wavenumber domain. To estimate the marginal distribution of the 3-D dipping fault parameters, we utilize the Bayesian theory that integrates the likelihood function and prior information. Subsequently, we use the Markov chain Monte Carlo sampling method to assess the marginal distribution of the parameters. Compared with other deterministic inversion approaches, our method provides an effective means of quantifying parameter uncertainty. We first use synthetic data to validate the effectiveness of the proposed method. The results demonstrate the efficiency of our method in revealing 3-D dipping fault parameters and evaluating their uncertainty. We subsequently apply our method to the Pudu River fault, located in Yunnan Province, China, to demonstrate its practical applicability. Two alternative realistic fault models referred the prior seismic profile have been proposed. The optimal parameters and uncertainty with the specific model have been estimated. The results show that the fault characteristics retrieved by our method deviate slightly from existing knowledge. Specifically, the estimated depth of the bottom surface is greater than that reported in previous studies. This could potentially offer us new perspectives on the geological evolution and structural attributes of this fault.
更多查看译文
关键词
Gravity anomalies and Earth structure, Inverse theory, Probability distributions, Statistical methods
AI 理解论文
溯源树
样例
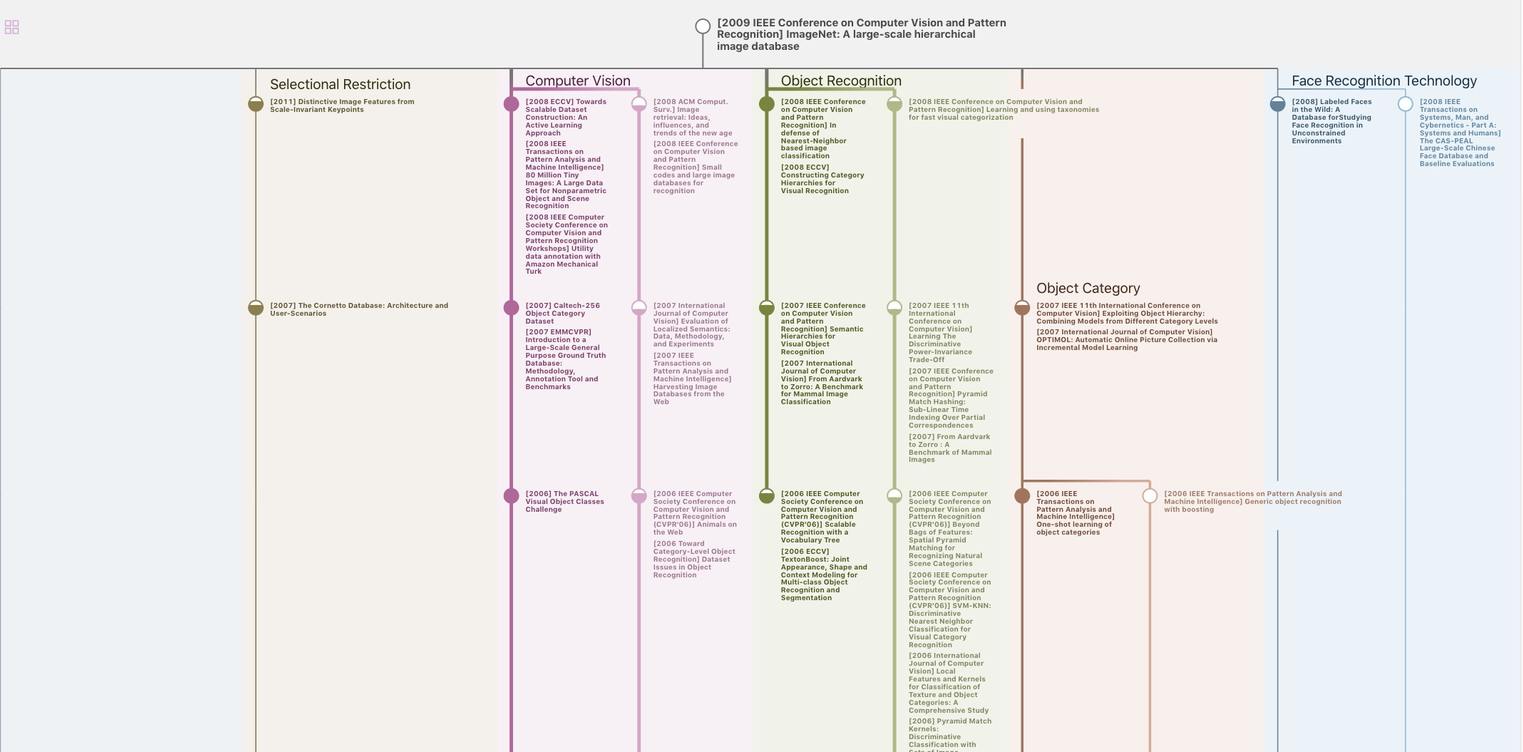
生成溯源树,研究论文发展脉络
Chat Paper
正在生成论文摘要