KMSA-Net: A Knowledge-Mining-Based Semantic-Aware Network for Cross-Domain Industrial Process Fault Diagnosis
IEEE TRANSACTIONS ON INDUSTRIAL INFORMATICS(2024)
摘要
Process fault diagnosis is of great importance to ensure the safe and stable operation of industrial systems. Many existing deep-learning-based process fault diagnosis methods assume that the samples are sufficient and obey the same distribution; however, it is almost impossible to achieve in practical industrial applications due to changing working conditions and the high cost of acquiring fault samples, which leads to a prominent performance degradation. In essence, those methods do not fully exploit the intrinsic and relevant knowledge under different working conditions. To address the above issue, a knowledge-mining-based semantic-aware network (KMSA-Net) is proposed in this article. First, a self-correlation knowledge mining subnet is proposed, where unshared attention mechanism is designed to extract knowledge inherent in each working condition so that the discriminative features can be captured. Second, a cross-correlation knowledge mining subnet is proposed, where we develop a fault relational knowledge graph so as to explicitly constrain the local consistency between the source domain, target domain, and cross-domain. Third, a semantic-aware knowledge transfer subnet is designed to impose a semantic constraint during knowledge transfer by encouraging the output of KMSA-Net to be consistent and distinguishable. These three subnets are jointly trained and then applied for cross-domain industrial process fault diagnosis. Finally, benchmark simulated experiments and real-world application experiments are conducted, and the experimental results validate the effectiveness and superiority of the proposed method.
更多查看译文
关键词
Feature extraction,Fault diagnosis,Knowledge engineering,Data mining,Sensitivity,Knowledge transfer,Knowledge graphs,Cross-domain industrial process fault diagnosis,domain adaptation (DA),knowledge mining,transfer learning (TL)
AI 理解论文
溯源树
样例
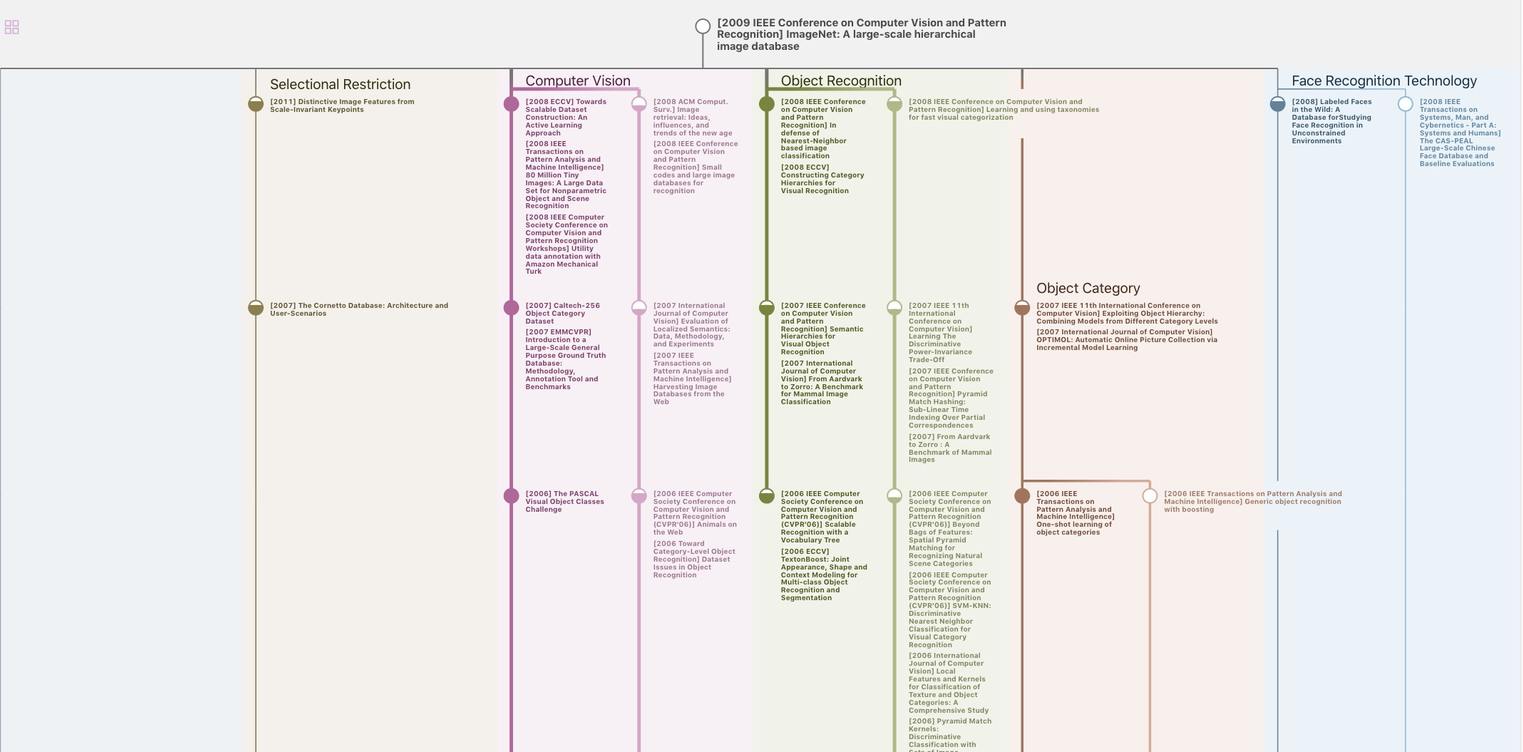
生成溯源树,研究论文发展脉络
Chat Paper
正在生成论文摘要