Data-Driven Flow and Injection hstimation in PMU-Unobservable Transmission Systems
2023 IEEE POWER & ENERGY SOCIETY GENERAL MEETING, PESGM(2023)
摘要
Fast and accurate knowledge of power flows and power injections is needed for a variety of applications in the electric grid. Phasor measurement units (PMUs) can be used to directly compute them at high speeds; however, a large number of PMUs will be needed for computing all the flows and injections. Similarly, if they are calculated from the outputs of a linear state estimator, then their accuracy will deteriorate due to the quadratic relationship between voltage and power. This paper employs machine learning to perform fast and accurate flow and injection estimation in power systems that are sparsely observed by PMUs. We train a deep neural network (DNN) to learn the mapping function between PMU measurements and power flows/injections. The relation between power flows and injections is incorporated into the DNN by adding a linear constraint to its loss function. The results obtained using the IEEE 118-bus system indicate that the proposed approach performs more accurate Clow/injection estimation in severely unobservable power systems compared to other data-driven methods.
更多查看译文
关键词
Terms Flow and Injection estimation, Machine learning (ML), Phasor measurement unit (PMU), Unobservability
AI 理解论文
溯源树
样例
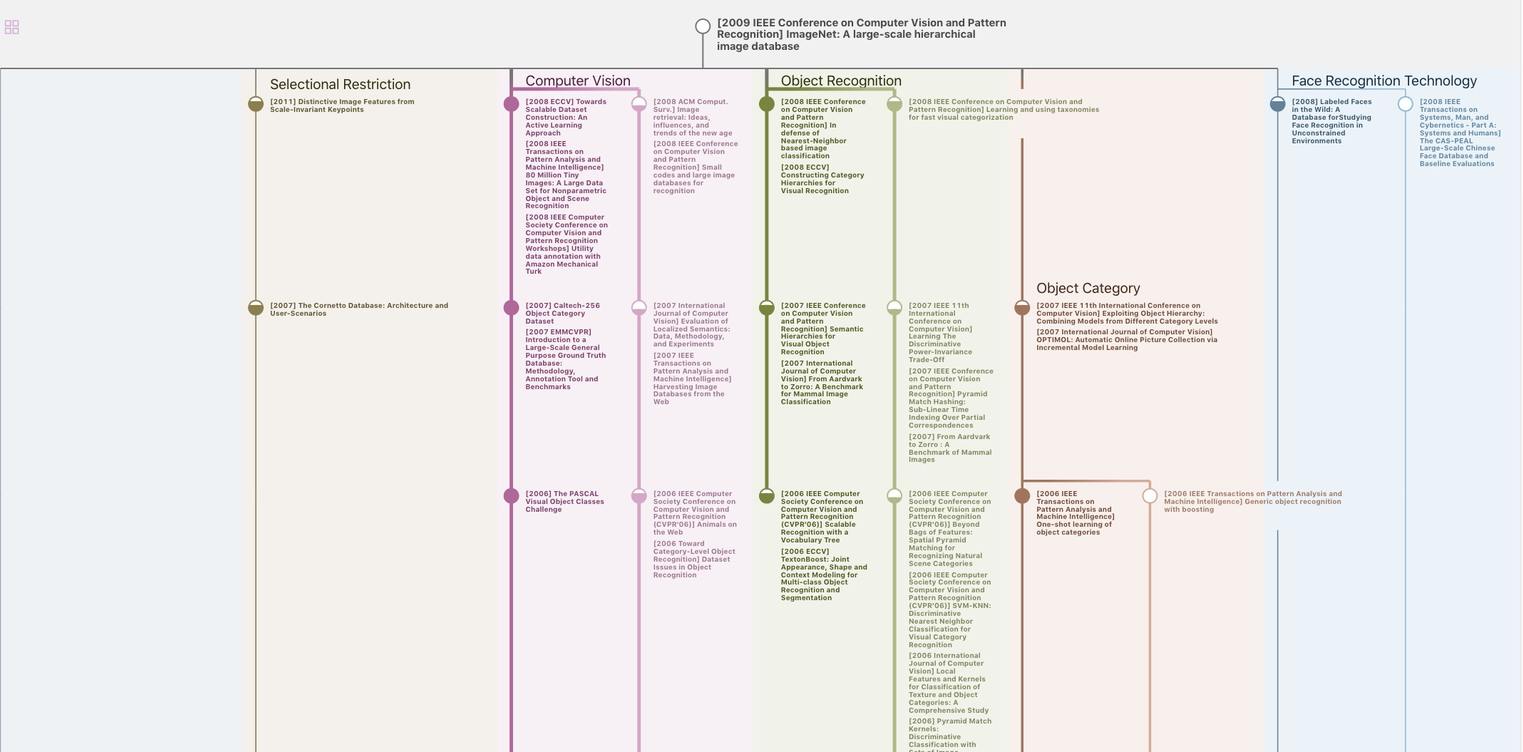
生成溯源树,研究论文发展脉络
Chat Paper
正在生成论文摘要