A SSIM Guided Cgan Architecture for Clinically Driven Generative Image Synthesis of Multiplexed Spatial Proteomics Channels
2023 IEEE CONFERENCE ON COMPUTATIONAL INTELLIGENCE IN BIOINFORMATICS AND COMPUTATIONAL BIOLOGY, CIBCB(2023)
摘要
Histopathological work in clinical labs often relies on immunostaining of proteins, which can be time-consuming and costly. Multiplexed spatial proteomics imaging can increase interpretive power, but current methods cannot cost-effectively sample the entire proteomic retinue important to diagnostic medicine or drug development. To address this challenge, we developed a conditional generative adversarial network (cGAN) that performs image-to-image (i2i) synthesis to generate accurate biomarker channels in multiplexed spatial proteomics images(1).We approached this problem as missing biomarker expression generation, where we assumed that a given n-channel multiplexed image has p channels (biomarkers) present and q channels (biomarkers) absent, with p+q=n, and we aimed to generate the missing q channels. To improve accuracy, we selected p and q channels based on their structural similarity, as measured by a structural similarity index measure (SSIM). We demonstrated the effectiveness of our approach using spatial proteomic data from the Human BioMolecular Atlas Program (HuBMAP)(2), which we used to generate spatial representations of missing proteins through a U-Net based image synthesis pipeline. Channels were hierarchically clustered by SSIM to obtain the minimal set needed to recapitulate the underlying biology represented by the spatial landscape of proteins. We also assessed the scalability of our algorithm using regression slope analysis, which showed that it can generate increasing numbers of missing biomarkers in multiplexed spatial proteomics images. Furthermore, we validated our approach by generating a new spatial proteomics data set from human lung adenocarcinoma tissue sections and showed that our model could accurately synthesize the missing channels from this new data set. Overall, our approach provides a cost-effective and time-efficient alternative to traditional immunostaining methods for generating missing biomarker channels, while also increasing the amount of data that can be generated through experiments. This has important implications for the future of medical diagnostics and drug development, and raises important questions about the ethical implications of utilizing data produced by generative image synthesis in the clinical setting.
更多查看译文
关键词
cancer imaging,image synthesis,CODEX,multiplexed image synthesis
AI 理解论文
溯源树
样例
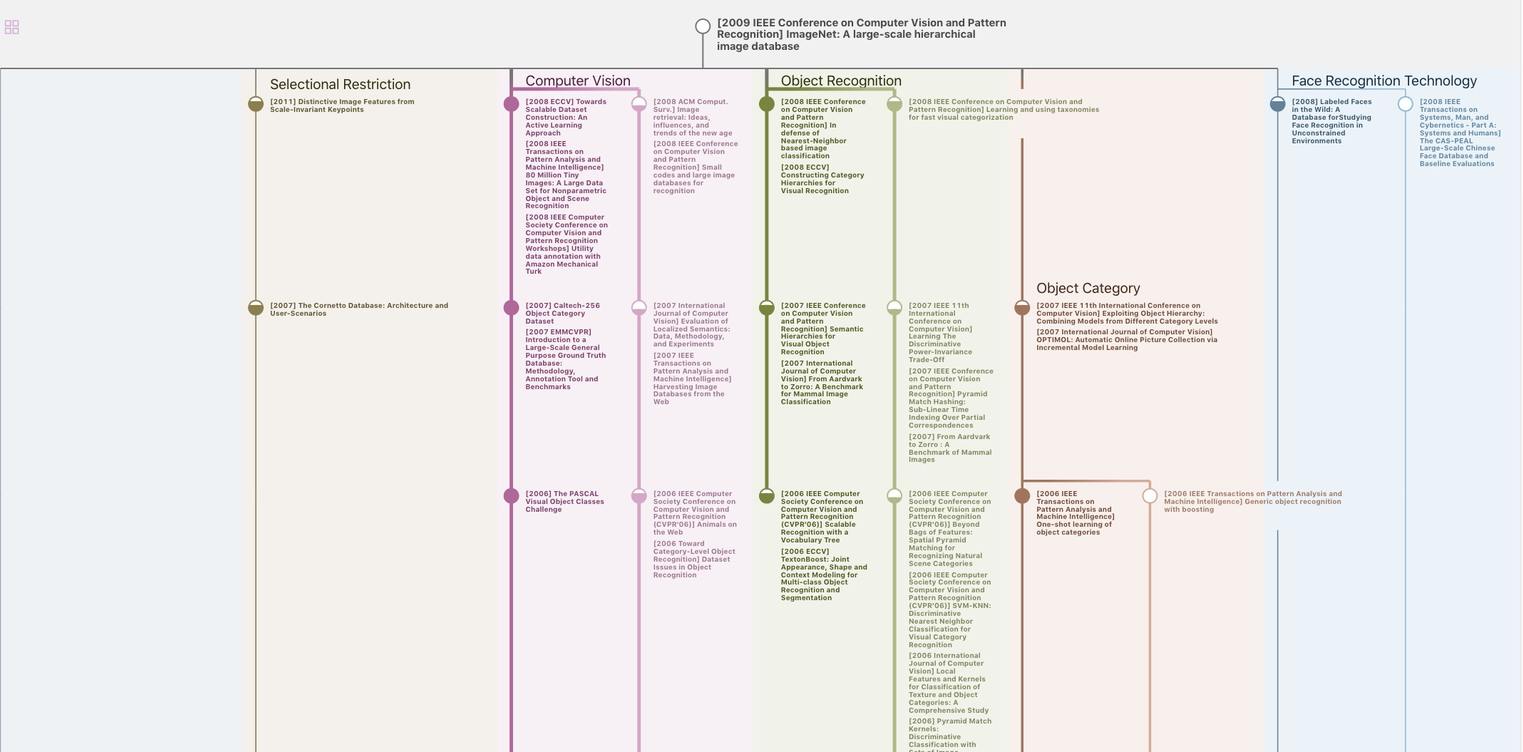
生成溯源树,研究论文发展脉络
Chat Paper
正在生成论文摘要