NLP-Crowdsourcing Hybrid Framework for Inter-Researcher Similarity Detection
IEEE TRANSACTIONS ON HUMAN-MACHINE SYSTEMS(2023)
摘要
Visualizing and examining the intellectual landscape and evolution of scientific communities to support collaboration is crucial for multiple research purposes. In some cases, measuring similarities and matching patterns between research publication document sets can help to identify people with similar interests for building research collaboration networks and university–industry linkages. The premise of this work is assessing feasibility for resolving ambiguous cases in similarity detection to determine authorship with natural language processing (NLP) techniques so that crowdsourcing is applied only in instances that require human judgment. Using an NLP-crowdsourcing convergence strategy, we can reduce the costs of microtask crowdsourcing while saving time and maintaining disambiguation accuracy over large datasets. This article contributes a next-gen crowd-artificial intelligence framework that used an ensemble of term frequency-inverse document frequency and bidirectional encoder representation from transformers to obtain similarity rankings for pairs of scientific documents. A sequence of content-based similarity tasks was created using a crowd-powered interface for solving disambiguation problems. Our experimental results suggest that an adaptive NLP-crowdsourcing hybrid framework has advantages for inter-researcher similarity detection tasks where fully automatic algorithms provide unsatisfactory results, with the goal of helping researchers discover potential collaborators using data-driven approaches.
更多查看译文
关键词
Adaptive human–machine systems,bidirectional language models,crowdsourcing,human–computer interaction (HCI),natural language processing (NLP),text-based similarity algorithms,term frequency-inverse document frequency (TF-IDF)
AI 理解论文
溯源树
样例
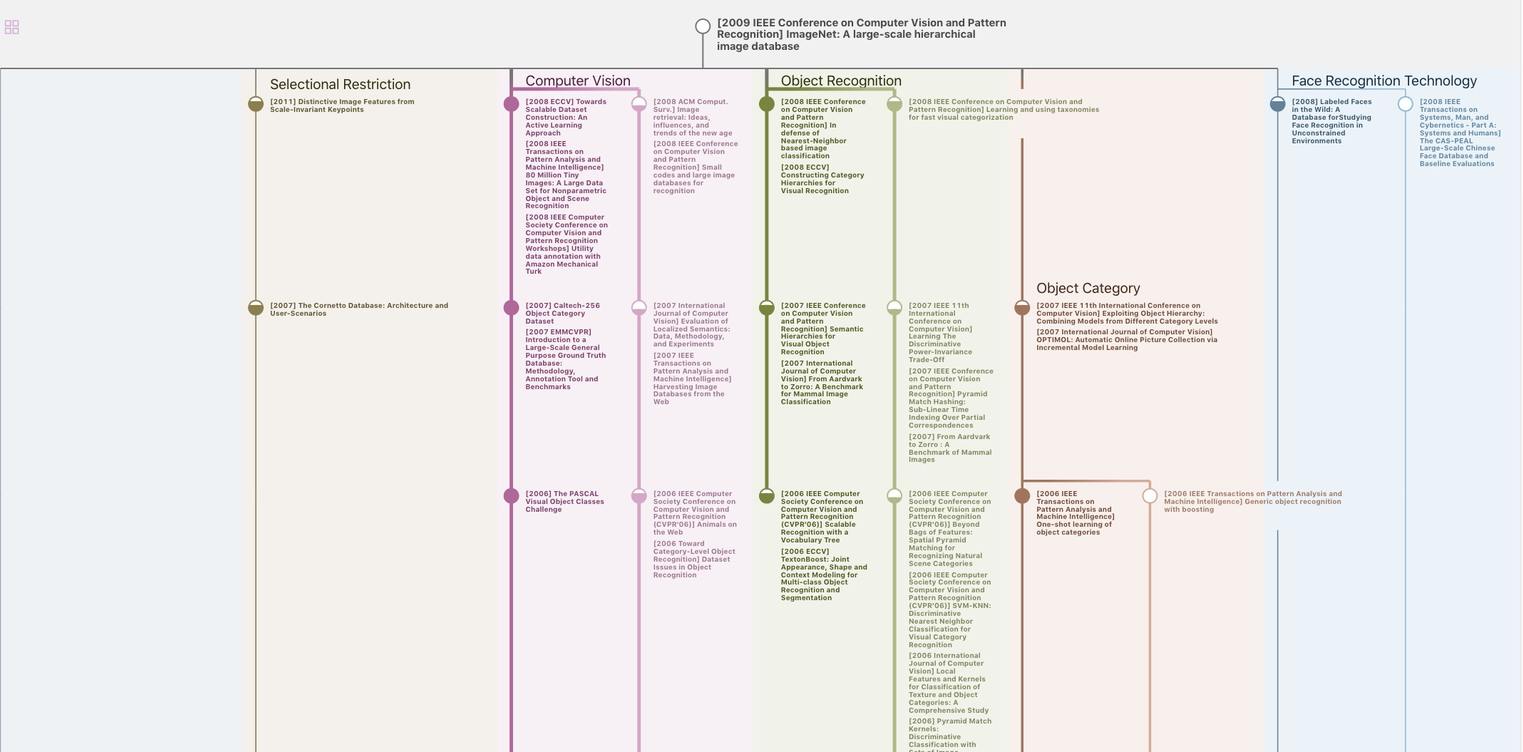
生成溯源树,研究论文发展脉络
Chat Paper
正在生成论文摘要