Transfer Learning with Prior Data- Driven Models from Multiple Unconventional Fields
SPE JOURNAL(2023)
摘要
Constructing reliable data-driven models to predict well production performance (e.g., estimated ultimate recovery, cumulative produc-tion, production curves, etc.) for unconventional reservoirs requires large amounts of data. However, when considering unconventional reservoirs in their early stages of development, where data and the wells drilled are limited, one may benefit from leveraging available data and/or pretrained models from other more developed fields. Transfer learning, the process of storing knowledge gained while solving one problem (source data) and applying it to solve a different but related problem (target data), provides a workflow for alleviating data needs in training a data-driven model in fields with limited data. However, a pitfall in the application of transfer learning is the possibility of negative transfer, that is, transferring incorrect or irrelevant knowledge to the target data. In particular, the black -box nature of most data-driven models, e.g., neural networks, support vector machines, and random forest, makes it difficult to completely interpret the con-tribution of different source models used for knowledge transfer. Hence, ranking the viability of source models for transfer learning can reduce the risk of negative transfer and improve the prediction performance. In this paper, we illustrate the impact of negative transfer and how it can be identified, and present a new approach for ranking multiple source models based on their positive transfer contribution. Finally, we propose a framework to build a reliable model to predict well production performance by combining multiple sources of in-formation into one network to be transferred and retrained with limited data in fields at their early stages of development.
更多查看译文
关键词
transfer learning,multiple unconventional fields,models,data-driven
AI 理解论文
溯源树
样例
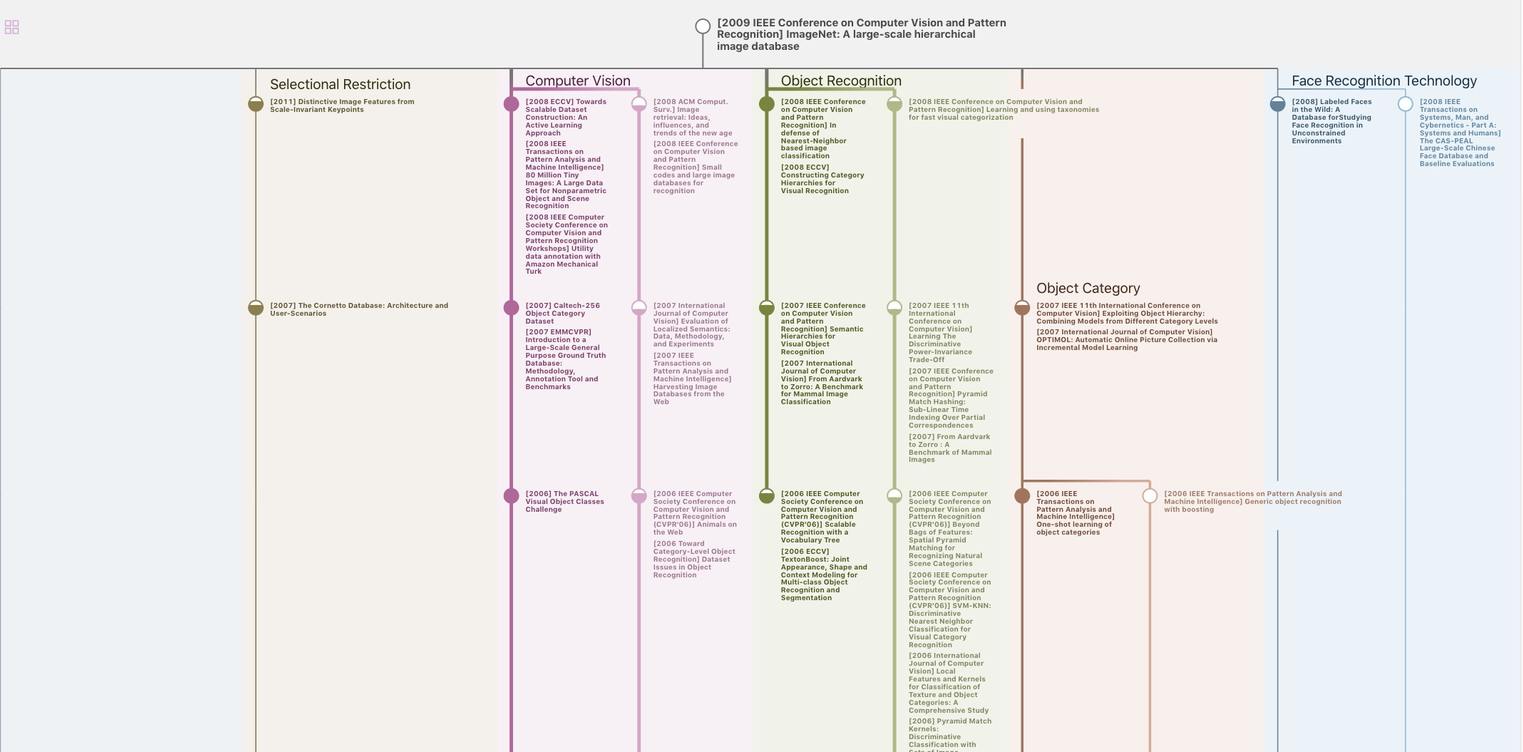
生成溯源树,研究论文发展脉络
Chat Paper
正在生成论文摘要