Differential Evolution with a Level-Based Learning Strategy for Multimodal Optimization
INTERNATIONAL JOURNAL OF INTELLIGENT SYSTEMS(2023)
摘要
Multimodal optimization aims at efficiently finding multiple optimal solutions of a problem. Owing to the population-based search mechanism, evolutionary algorithms (EAs) are becoming increasingly popular in solving multimodal optimization problems (MOPs). Most existing work focuses on designing and incorporating niching techniques into EAs so that multiple subpopulations can be formed and assigned to locate different optima. To further enhance the exploration and exploitation abilities of existing EAs, this paper developed a multimodal level-based learning strategy. The basic idea is that individuals should be treated differently according to their positions in the subpopulation. In the evolutionary process, a subpopulation is formed for each candidate solution by grouping its neighboring solutions. Then, individuals in the subpopulation are sorted according to their fitness. Subsequently, the multimodal level-based learning strategy applies different mutation operators to different individuals according to their rankings. Experiments are conducted on a set of benchmark problems to verify the efficacy of the multimodal level-based learning strategy. The results show that the proposed learning strategy can significantly enhance the performance of the existing algorithm. In addition, the algorithm integrated with the proposed strategy is applied to the task of finding multiple roots of nonlinear equation systems (NESs). The results indicate that with the support of the proposed learning strategy, the integrated algorithm compares favorably with state-of-the-art root finding algorithms.
更多查看译文
关键词
multimodal optimization,learning strategy,evolution,level-based
AI 理解论文
溯源树
样例
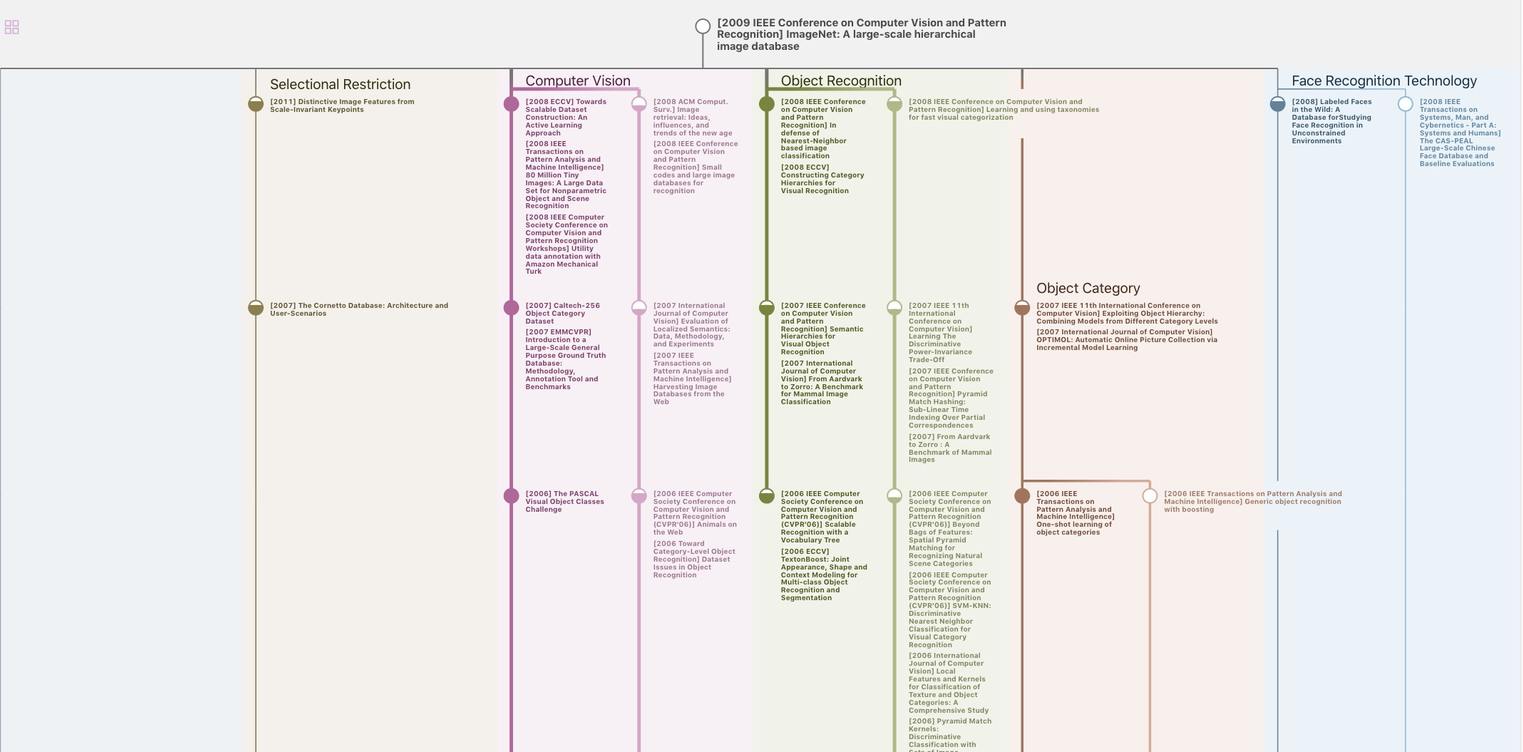
生成溯源树,研究论文发展脉络
Chat Paper
正在生成论文摘要