Single-Agent Reinforcement Learning for Scalable Earth-Observing Satellite Constellation Operations
JOURNAL OF SPACECRAFT AND ROCKETS(2024)
摘要
This work explores single-agent reinforcement learning for the multi-satellite agile Earth-observing scheduling problem. The objective of the problem is to maximize the weighted sum of imaging targets collected and downlinked while avoiding resource constraint violations on board the spacecraft. To avoid the computational complexity associated with multi-agent deep reinforcement learning while creating a robust and scalable solution, a policy is trained in a single satellite environment. This policy is then deployed on board each satellite in a Walker-delta constellation. A global set of targets is distributed to each satellite based on target access. The satellites communicate with one another to determine whether an imaging target is imaged or downlinked. Free communication, line-of-sight communication, and no communication are explored to determine how the communication assumptions and constellation design impact performance. Free communication is shown to produce the best performance, and no communication is shown to produce the worst performance. Line-of-sight communication performance is shown to depend heavily on the design of the constellation and how frequently the satellites can communicate with one another. To explore how higher-level coordination can impact performance, a centralized mixed-integer programming optimization approach to global target distribution is explored and compared to a decentralized approach. A genetic algorithm is also implemented for comparison purposes, and the proposed method is shown to achieve higher reward on average at a fraction of the computational cost.
更多查看译文
关键词
Agile Satellite Scheduling,Resource Allocation,Space-Terrestrial Integration
AI 理解论文
溯源树
样例
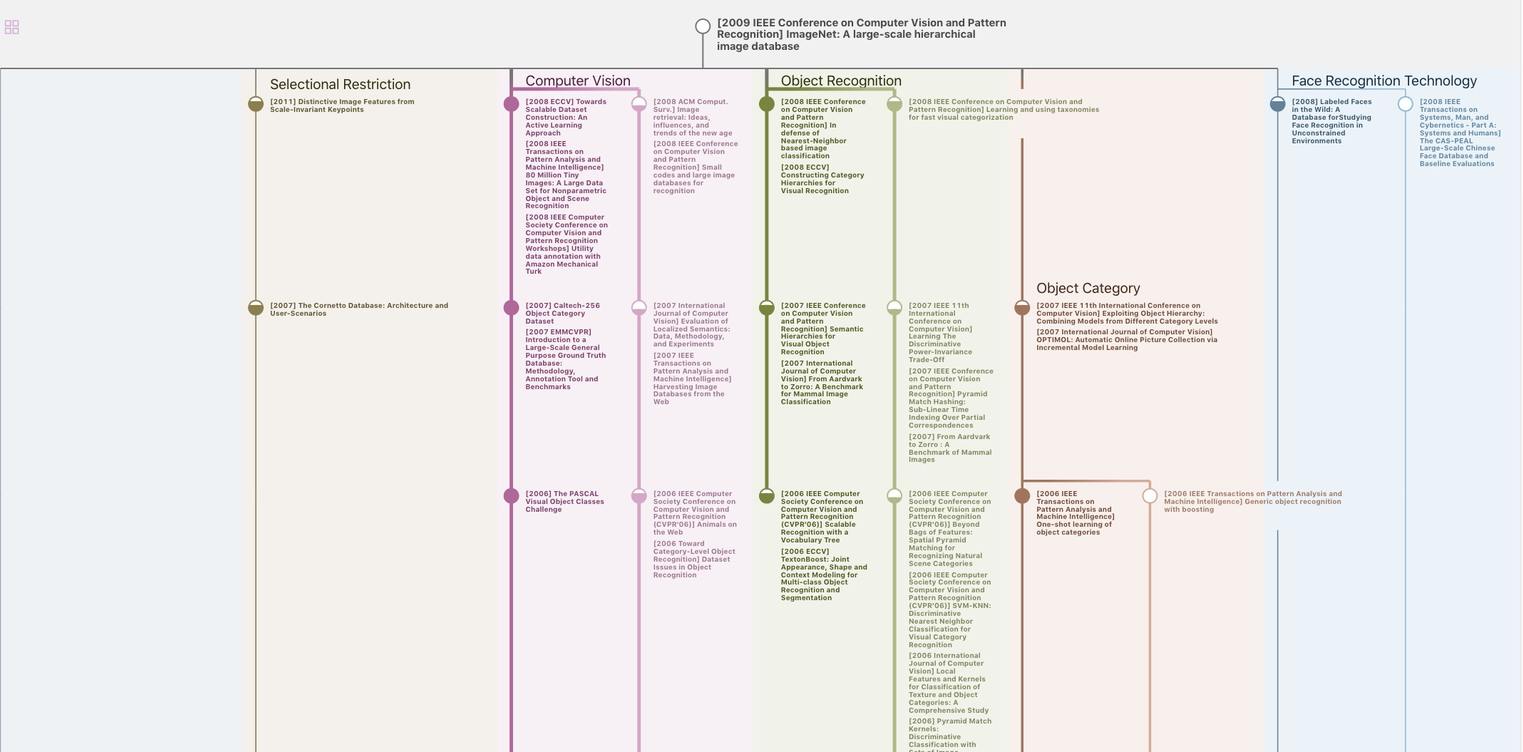
生成溯源树,研究论文发展脉络
Chat Paper
正在生成论文摘要