On propensity score misspecification in double/debiased machine learning for causal inference: ensemble and stratified approaches
COMMUNICATIONS IN STATISTICS-SIMULATION AND COMPUTATION(2023)
摘要
Causal inference based on double/debiased machine learning (DML) requires a choice of a machine learning method. Using an inappropriate machine learning method to fit the propensity score function can result in bias when estimating the average treatment effect (ATE). We consider two possible solutions to this issue. The ensemble approach involves aggregating propensity scores from various machine learning methods to estimate the ATE. The stratified approach involves utilizing one of the two propensity score estimators for calculating inverse propensity weights within each stratum, while the other is employed to determine the strata boundaries. Through Monte Carlo simulation experiments, we assess the performance of the ensemble DML and the stratified DML, as well as the original DML. The results demonstrate comparable performance between ensemble DML and stratified DML overall, yet the latter may provide advantages in specific scenarios.
更多查看译文
关键词
Causal inference, machine learning, model selection, propensity score, stratification
AI 理解论文
溯源树
样例
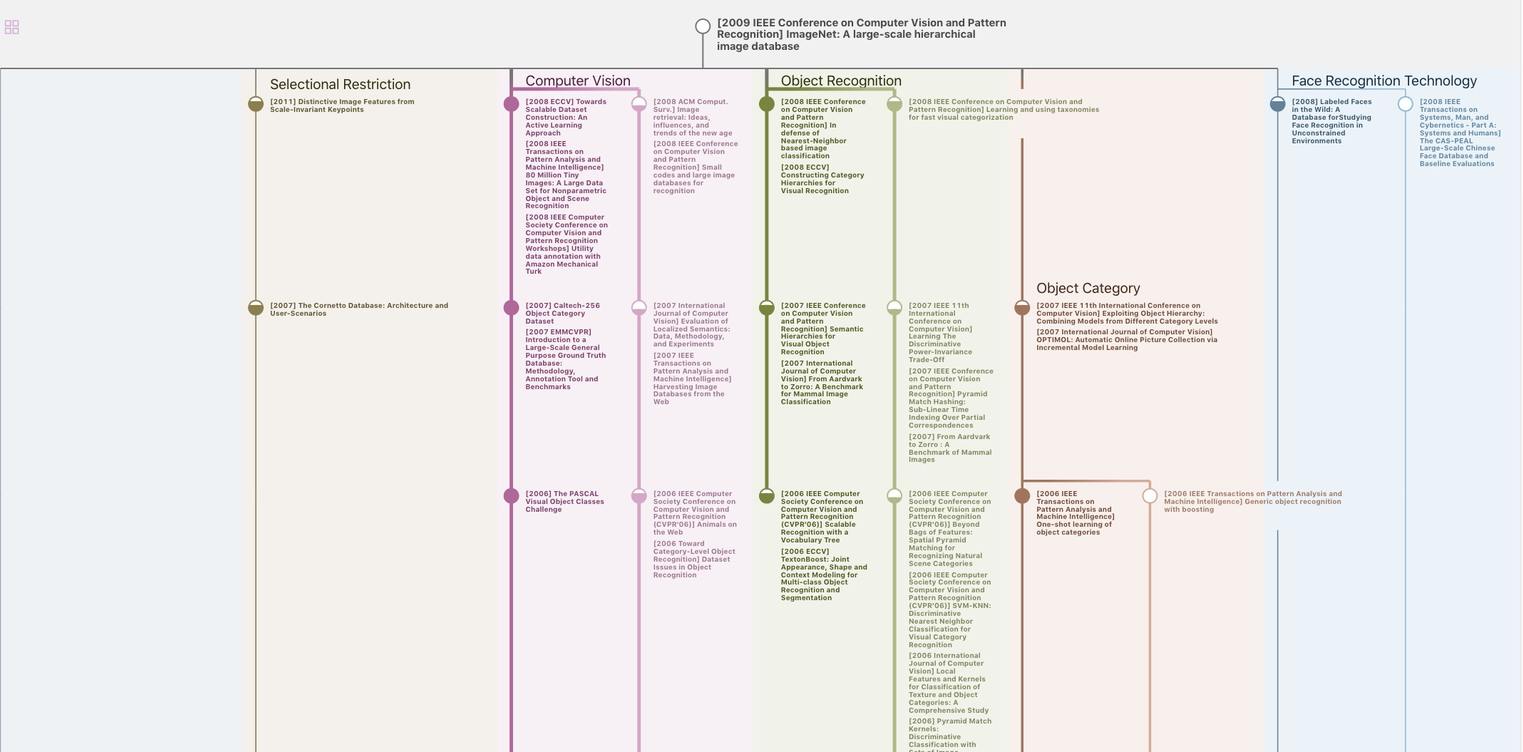
生成溯源树,研究论文发展脉络
Chat Paper
正在生成论文摘要