Low visibility event prediction using random forest and K-nearest neighbor methods
THEORETICAL AND APPLIED CLIMATOLOGY(2024)
摘要
Low visibility events at King Khalid airport in Riyadh, Saudi Arabia, are investigated using hourly time series of meteorological and air pollution data from April 2015 to December 2017. The analysis of binary classification is based on two machine learning classifiers (random forest (RF) and K-nearest neighbors (KNN)). Six models based on the feature selection methods of RF feature importance and Pearson correlation matrix are presented. The classification tasks include two resampling approaches (random oversampling and random undersampling) to address the problem of an imbalanced dataset of the visibility event classes. An important finding is that oversampling outperforms undersampling for the evaluated classifiers and achieves higher scores in terms of accuracy and F1 score metrics. The RF classifier has a better performance compared to the KNN in both sampling approaches. The RF classifier with oversampling approach provides the best overall performance in terms of accuracy, F1 score, and area under the receiver operating characteristics (AUROC). The best model has scores above 0.95 based on all the evaluation metrics considered in the study. Air temperature and dewpoint temperature have minimal impact on the performance, whereas the particulate matter with aerodynamic diameter <10 mu m (PM10) has a profound impact on the performance. It is found that the PM10 has the highest importance (52%) for the low visibility events based on the analysis of RF feature importance. Other pollutants and meteorological variables show relative importance between 5 and 10% for low visibility events. Overall, the best model is found when all variables, except temperature and dewpoint temperature, are employed to predict the visibility classes.
更多查看译文
AI 理解论文
溯源树
样例
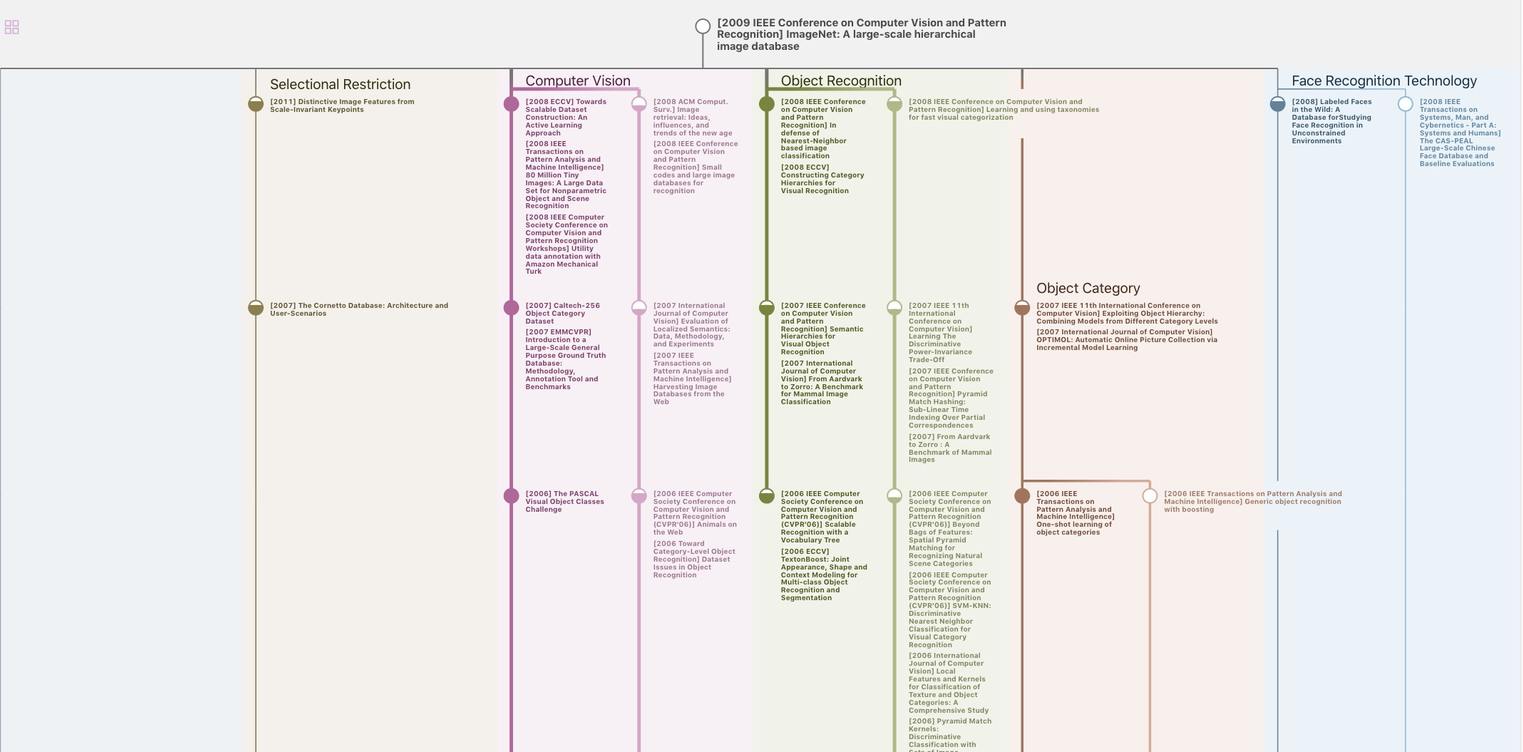
生成溯源树,研究论文发展脉络
Chat Paper
正在生成论文摘要