ReferPose: Distance Optimization-Based Reference Learning for Human Pose Estimation and Monitoring
IEEE TRANSACTIONS ON INDUSTRIAL INFORMATICS(2024)
摘要
Existing deep learning models for human pose estimation (HPE) have shown satisfactory performance in monitoring human actions. However, they usually face a dilemma between complexity and accuracy. To address this challenge, we propose an effective reference learning method for HPE (namely ReferPose), which is based on a new distance optimization strategy. Specifically, we utilize a reference model for pose learning and representation. The pose representation learned from the entire database is merged into the reference model, providing continuous reference learning guidance for an in-training model. In addition, we design a new cosine annealing-based reference guidance for temporal denoising and further develop a distance optimization strategy to provide joint guidance from pose knowledge, model representation, and temporal experience. Experimental results on two benchmark databases and a human fall monitoring system demonstrate that our ReferPose not only achieves promising accuracy improvement compared with several representative HPE models, but also offers low cost and high efficiency.
更多查看译文
关键词
Distance optimization,human action monitoring,reference learning
AI 理解论文
溯源树
样例
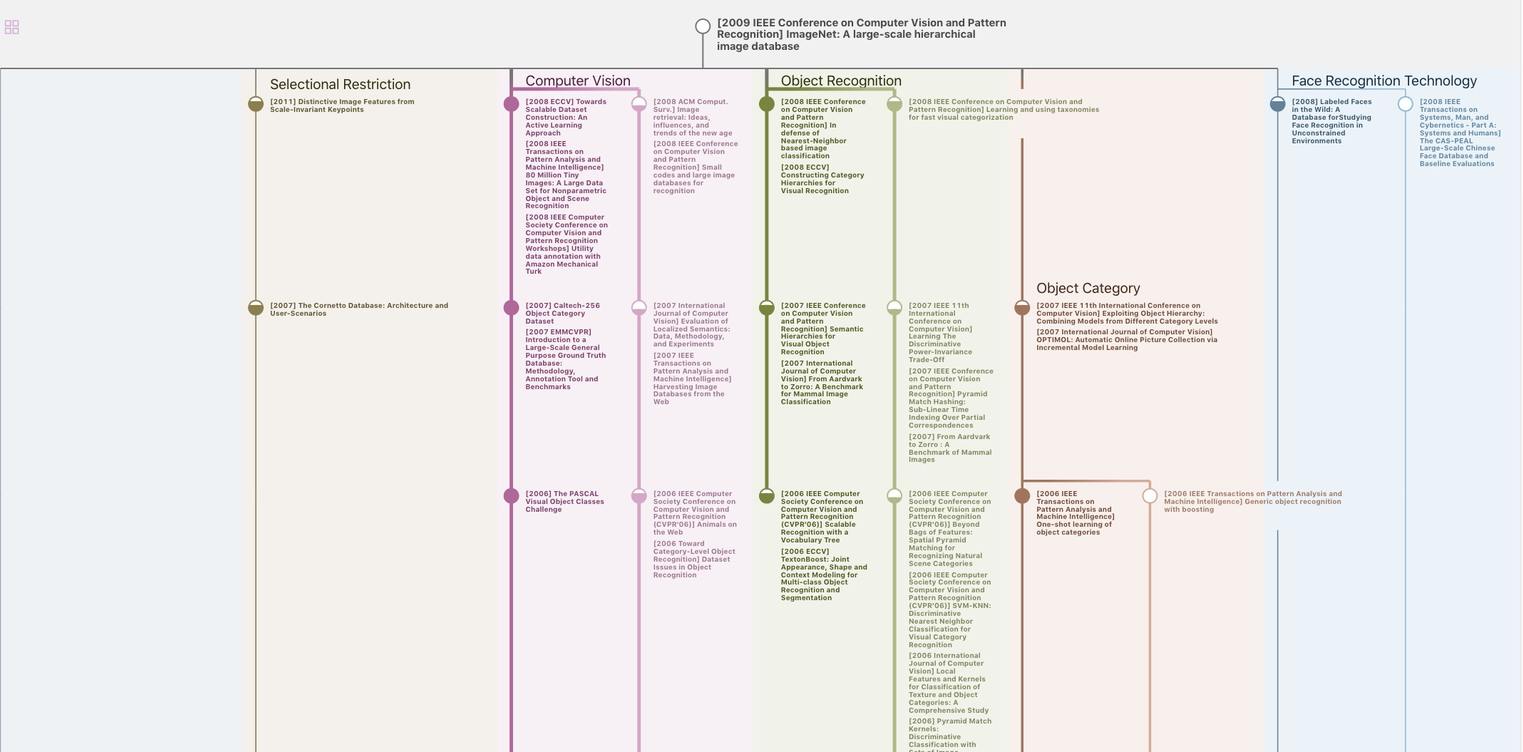
生成溯源树,研究论文发展脉络
Chat Paper
正在生成论文摘要