A General Knowledge-Guided Framework Based on Deep Probabilistic Network for Enhancing Industrial Process Modeling
IEEE TRANSACTIONS ON INDUSTRIAL INFORMATICS(2024)
摘要
Deep learning models are increasingly being used as effective techniques for industrial process modeling. However, decisions generated from deep learning models can hardly to interpret and cannot provide convinced results to users. To break the traditional tradeoff between accuracy and interpretability of deep neural network for industrial process modeling, we propose a deep probabilistic network-based knowledge-guided framework, which injects external knowledge into the deep neural network to guide its training process. In this framework, a deep probabilistic regression model (DPRM) is first developed to learn Gaussian latent feature representation yet establish regression relationship. Then, the external knowledge represented by fuzzy rules, which can evaluate the fitness of current network output and characterize constraints of the process, is encoded into a same structured Gaussian latent feature representation. We propose to inject the feature representation of external knowledge into DPRM using Kullback-Leibler divergence between two Gaussian distributions. The proposed knowledge-guided framework is evaluated on the Tennessee Eastman Process and the real-world aluminum electrolysis process. Experimental results highlight that our proposed approach achieves better prediction performance than the compared methods, also interprets the results.
更多查看译文
关键词
Knowledge engineering,Process modeling,Process control,Probabilistic logic,Data models,Knowledge based systems,Artificial neural networks,Aluminum electrolysis process (AEP),deep probabilistic regression model,knowledge expression model,knowledge-guided framework
AI 理解论文
溯源树
样例
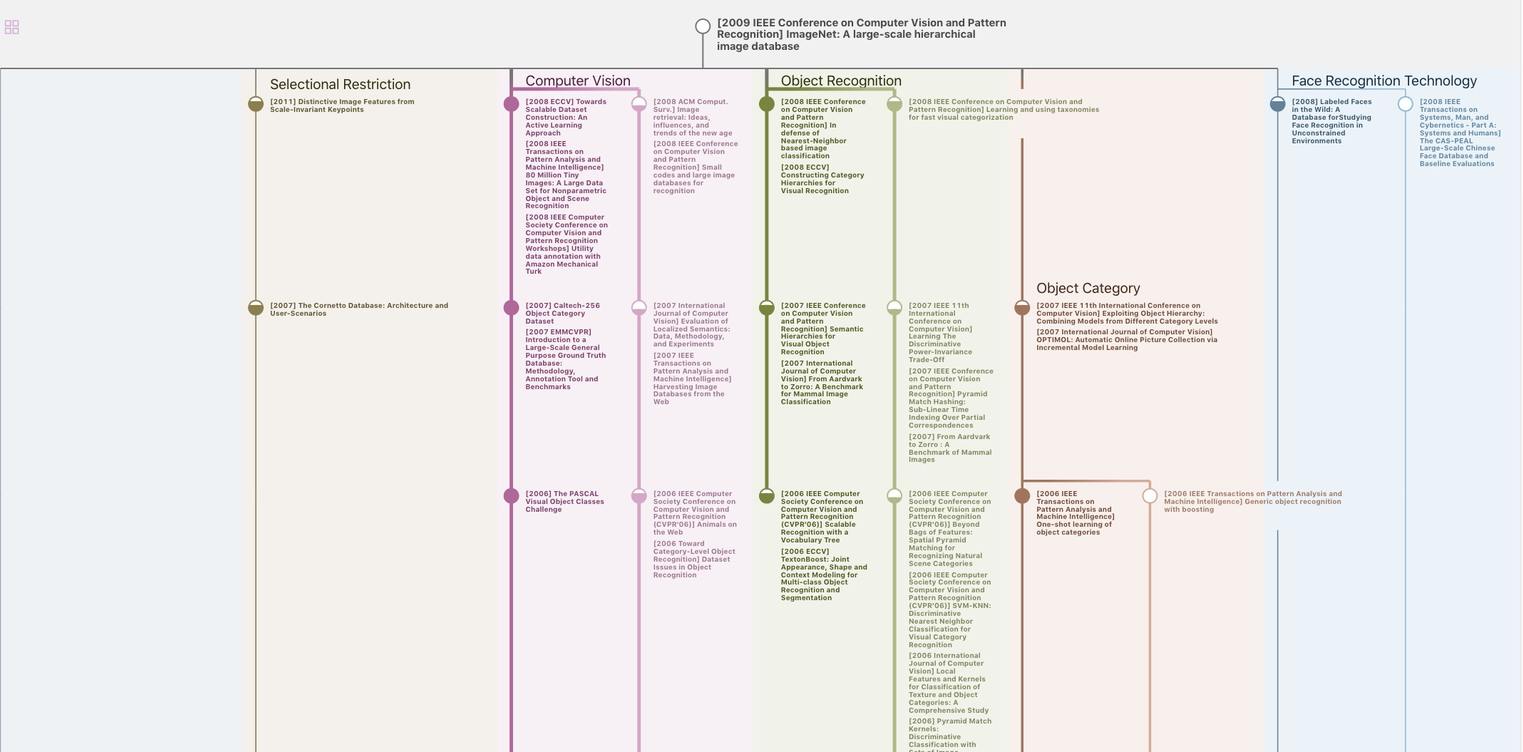
生成溯源树,研究论文发展脉络
Chat Paper
正在生成论文摘要