Towards explainable metaheuristics: Feature extraction from trajectory mining
EXPERT SYSTEMS(2023)
摘要
Explaining the decisions made by population-based metaheuristics can often be considered difficult due to the stochastic nature of the mechanisms employed by these optimisation methods. As industries continue to adopt these methods in areas that increasingly require end-user input and confirmation, the need to explain the internal decisions being made has grown. In this article, we present our approach to the extraction of explanation supporting features using trajectory mining. This is achieved through the application of principal components analysis techniques to identify new methods of tracking population diversity changes post-runtime. The algorithm search trajectories were generated by solving a set of benchmark problems with a genetic algorithm and a univariate estimation of distribution algorithm and retaining all visited candidate solutions which were then projected to a lower dimensional sub-space. We also varied the selection pressure placed on high fitness solutions by altering the selection operators. Our results show that metrics derived from the projected sub-space algorithm search trajectories are capable of capturing key learning steps and how solution variable patterns that explain the fitness function may be captured in the principal component coefficients. A comparative study of variable importance rankings derived from a surrogate model built on the same dataset was also performed. The results show that both approaches are capable of identifying key features regarding variable interactions and their influence on fitness in a complimentary fashion.
更多查看译文
关键词
trajectory mining,explainable metaheuristics,feature extraction
AI 理解论文
溯源树
样例
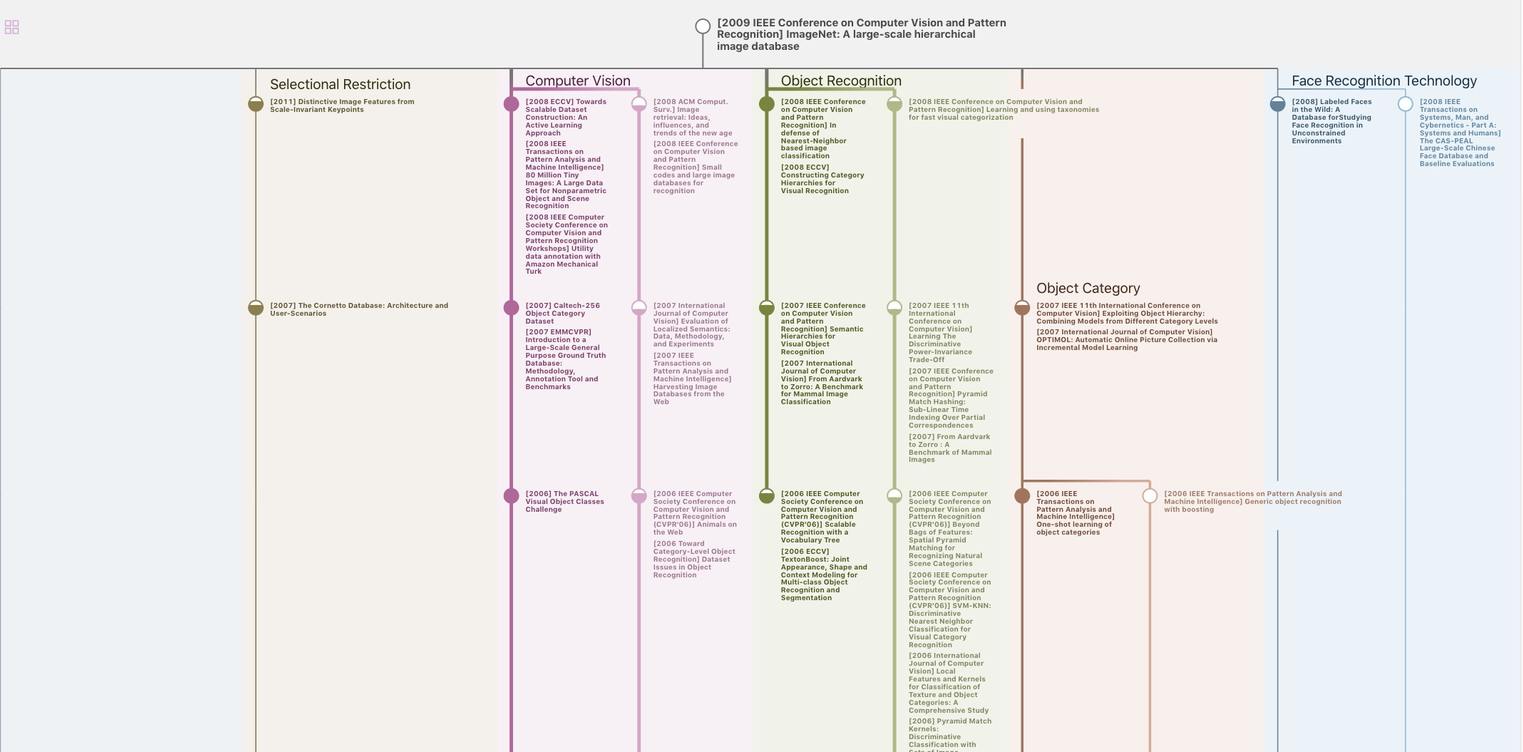
生成溯源树,研究论文发展脉络
Chat Paper
正在生成论文摘要