A semi-supervised approach to dark matter searches in direct detection data with machine learning
JOURNAL OF COSMOLOGY AND ASTROPARTICLE PHYSICS(2022)
摘要
The dark matter sector remains completely unknown. It is therefore crucial to keep an open mind regarding its nature and possible interactions. Focusing on the case of Weakly Interacting Massive Particles, in this work we make this general philosophy more concrete by applying modern machine learning techniques to dark matter direct detection. We do this by encoding and decoding the graphical representation of background events in the XENONnT experiment with a convolutional variational autoencoder. We describe a methodology that utilizes the 'anomaly score' derived from the reconstruction loss of the convolutional variational autoencoder as well as a pre-trained standard convolutional neural network, in a semi-supervised fashion. Indeed, we observe that optimum results are obtained only when both unsupervised and supervised anomaly scores are considered together. A data set that has a higher proportion of anomaly score is deemed anomalous and deserves further investigation. Contrary to classical analyses, in principle all information about the events is used, preventing unnecessary information loss. Lastly, we demonstrate the reach of learning-focused anomaly detection in this context by comparing results with classical inference, observing that, if tuned properly, these techniques have the potential to outperform likelihood-based methods.
更多查看译文
关键词
Machine learning, dark matter simulations, dark matter experiments, dark matter detectors
AI 理解论文
溯源树
样例
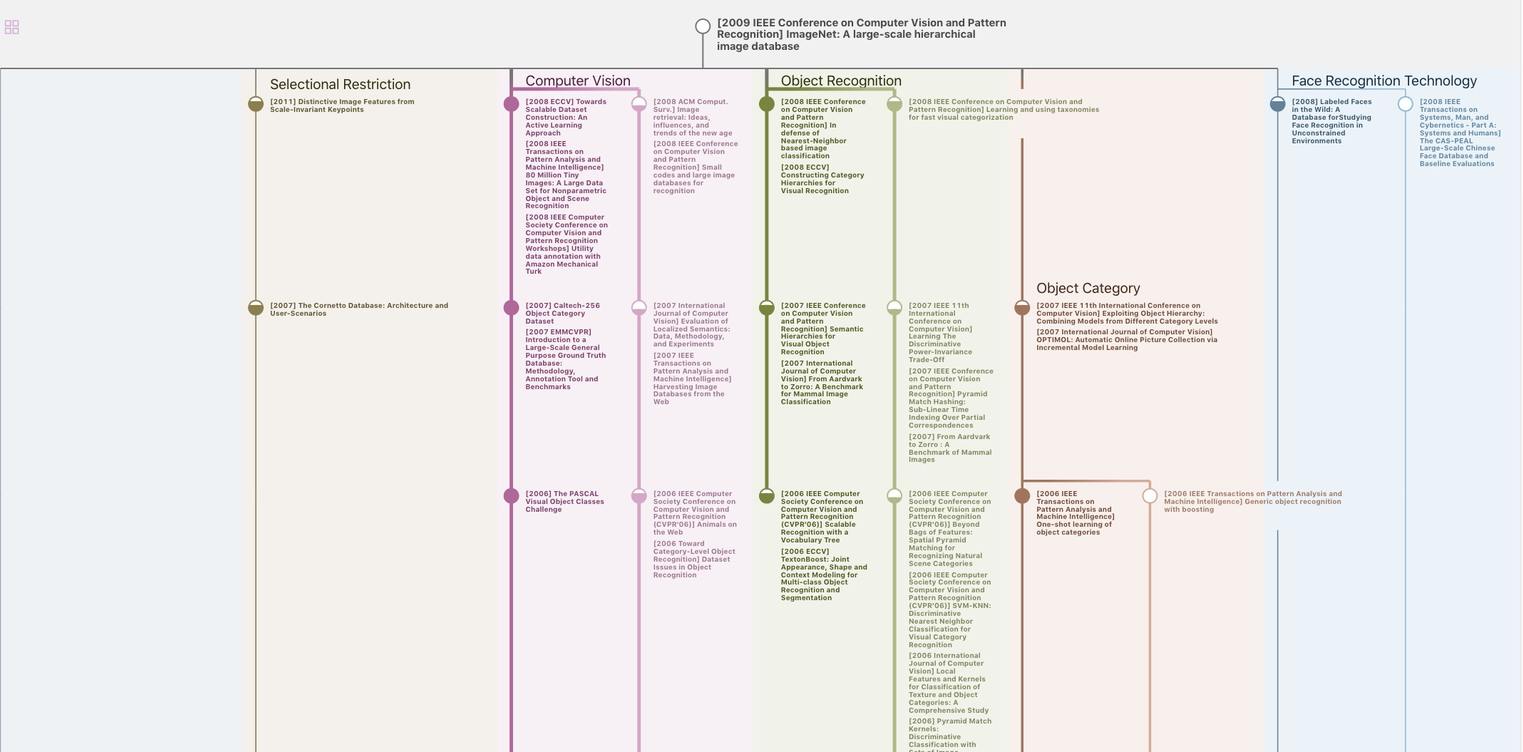
生成溯源树,研究论文发展脉络
Chat Paper
正在生成论文摘要