AI-Driven Counter-Terrorism: Enhancing Global Security Through Advanced Predictive Analytics
IEEE Access(2023)
摘要
Recent terrorist attacks have emerged as a formidable menace to global peace and security, giving rise to an acute humanitarian and economic crisis characterized by the loss of numerous lives and the incurring of substantial financial damages. In response to this pressing concern, the scholarly community has introduced a range of AI-driven predictive analytics methodologies as prospective instruments in the fight against terrorism. Machine Learning (ML) techniques, commonly employed in counter-terrorism efforts, face the formidable challenge of accurately forecasting terrorism activities due to the escalating complexity and voluminous nature of the underlying data. In this context, we present a solution in the form of an optimal weighted voting ensemble classifier, specially tailored for the classification of weapon types in terrorist attacks. Leveraging the computational capabilities of the Particle Swarm Optimization (PSO) algorithm, we determine the optimal weight assignments for the base learners, namely Random Forest and Xtreme Gradient Boosted Machines. Additionally, we developed several machine learning models to predict the likelihood of casualties in the aftermath of a terrorist incident. Rigorous validation of these models is carried out utilizing the publicly available Global Terrorism Database (GTD), necessitating meticulous data preprocessing to rectify anomalies and address class imbalances, a task effectively accomplished through the application of the Synthetic Minority Over-sampling Technique (SMOTE). To underscore the effectiveness of our proposed model, we conduct an extensive comparative analysis, bench-marking it against state-of-the-art machine learning models. The comprehensive experimental results unequivocally demonstrate the superiority of our models, as they consistently achieve the lowest error rates, thereby highlighting their enhanced generalizability and performance. Our detailed experiments show that the PSO-based classifier achieves 95% accuracy in weapon classification, while in case of casualty prediction, The XGB model showcases the most consistent and reliable performance across all datasets from Pakistan, India, and Afghanistan, with the lowest Mean Squared Error. The findings derived from our study furnish invaluable insights to empower counter-terrorism agencies, facilitating data-driven decision-making and the proactive implementation of measures to mitigate and counteract the scourge of terrorist acts.
更多查看译文
关键词
Predictive analytics,machine learning,spatial patterns,artificial intelligence
AI 理解论文
溯源树
样例
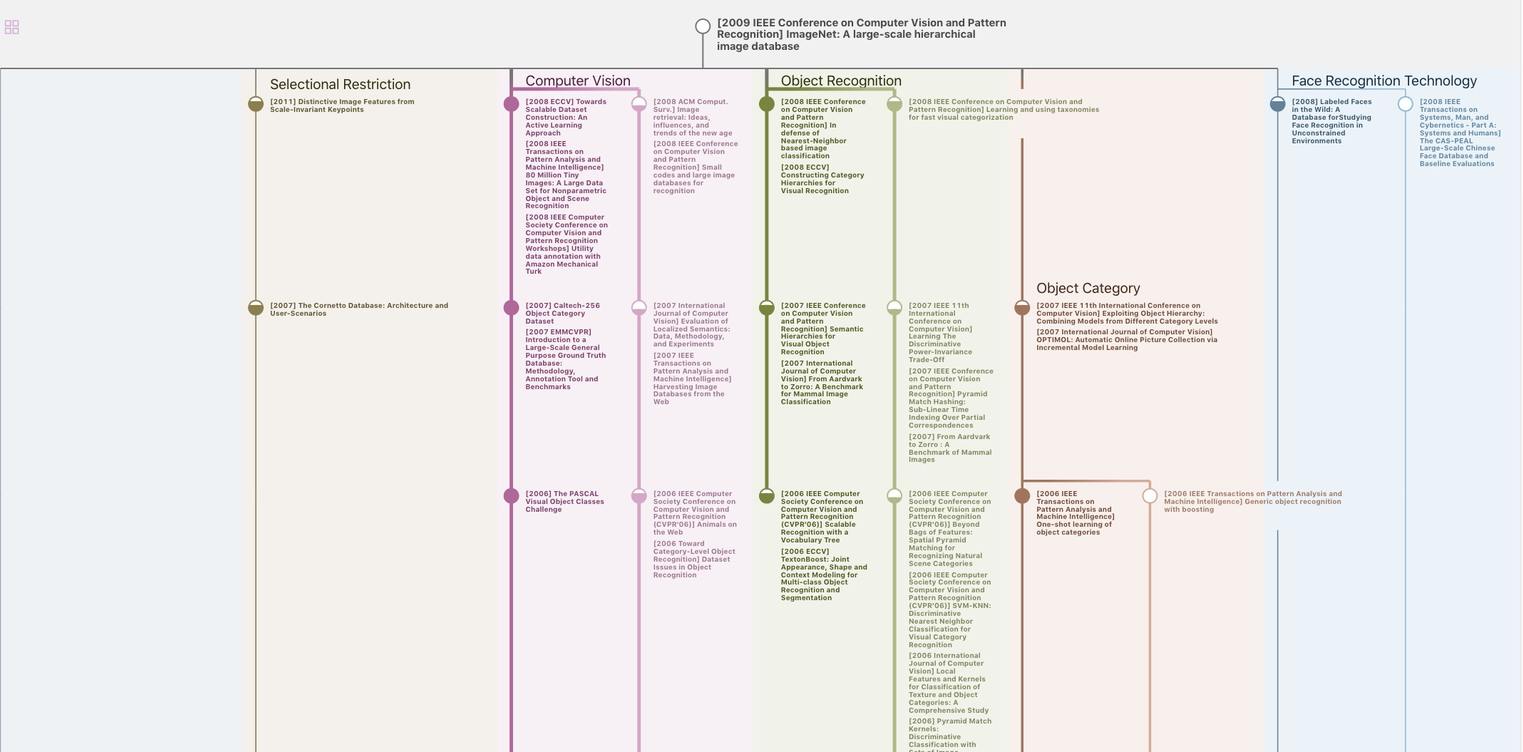
生成溯源树,研究论文发展脉络
Chat Paper
正在生成论文摘要