Study on Photofluorescent Uranium Ore Sorting Based on Deep Learning
MINERALS ENGINEERING(2024)
摘要
Uranium ore stands as a vital source of energy, and the process of ore sorting serves to segregate valuable uranium ore from its less valuable counterparts. This not only enhances the intrinsic ore grade but also mitigates the challenges associated with subsequent refining processes, thus leading to substantial resource cost savings. Consequently, the necessity of pre-sorting uranium ore becomes evident. While prevailing X-ray-based ore sorting technology remains at the forefront, the quest for a more efficacious method to sort uranium ore remains ongoing. This study harnesses the inherent photofluorescence properties of uranium ore by subjecting it to ultraviolet light, inducing the emission of a vibrant green fluorescence. Consequently, we propose a novel sorting technique founded on photofluorescence, augmented by deep learning to enhance the precision and categorization of uranium ore images. Two distinct models, the Convolutional Neural Network (CNN) model and the Transformer model, are developed in tandem. Empirical findings underscore the superiority of the Swin Transformer model, with training accuracy, validation accuracy, and classification rate registering at 0.9780, 0.9719, and 96.4 ms/it, respectively, surpassing the performance of alternative models like Alex Net and Res Net 34. Furthermore, the study employs visual aids, including channel visualization diagrams, confusion matrices, and Grad-CAM diagrams, to elucidate the operational intricacies of the model in the sorting of photofluorescent uranium ore images. This allows for an insightful analysis of the characteristics that influence classification weights. The potential applications of this method are indeed substantial, opening new avenues for uranium ore exploration and utilization.
更多查看译文
关键词
Uranium ore sorting,Photofluorescence,Deep learning,Swin Transformer
AI 理解论文
溯源树
样例
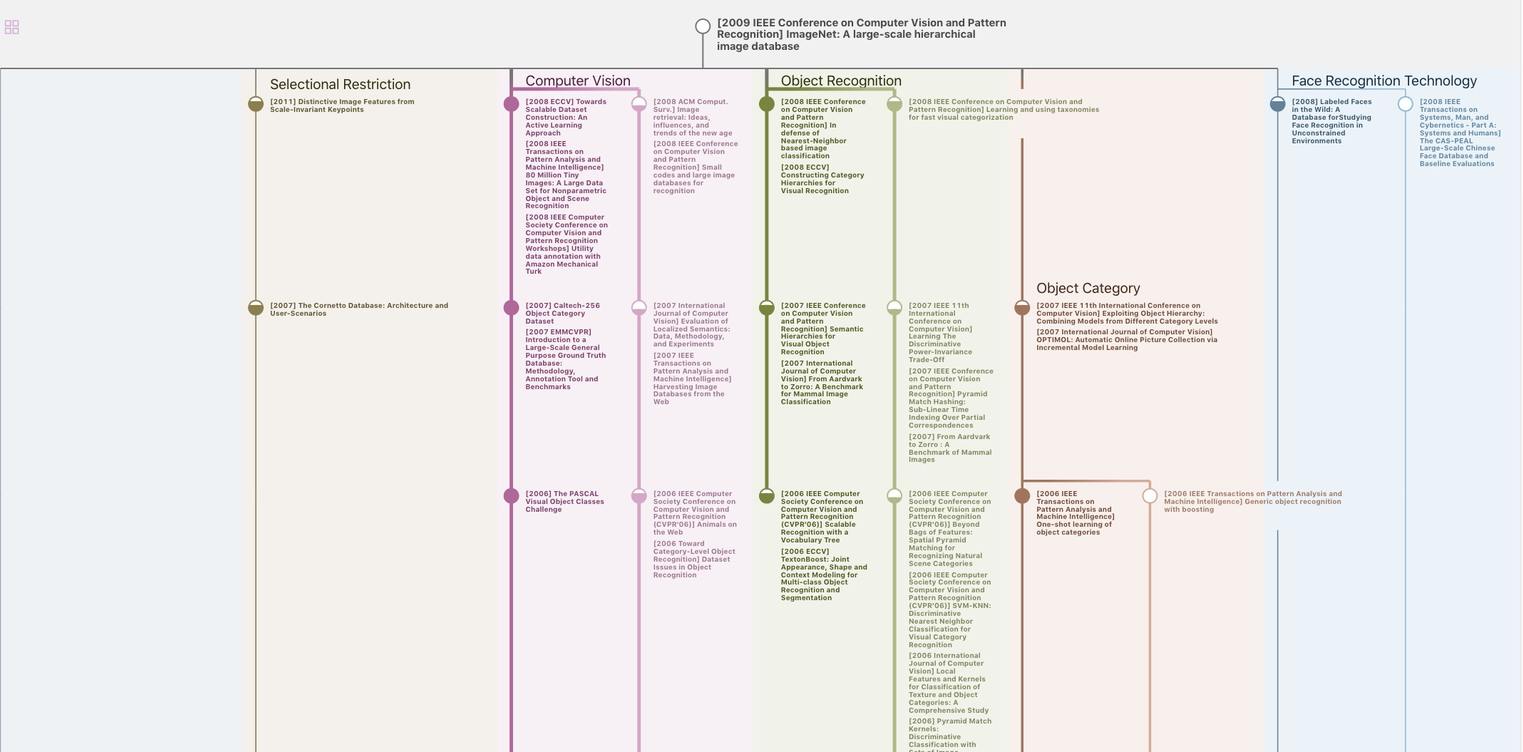
生成溯源树,研究论文发展脉络
Chat Paper
正在生成论文摘要