Unscented Kalman Filter for Distribution Networks with Quantization Effects and False Data Injection Attacks
2023 International Conference on Power System Technology (PowerCon)(2023)
摘要
An improvement of the unscented Kalman filter algorithm is designed for the problem of distribution network state estimation under the influence of quantization effects and false data injection attacks. Firstly, the measured data are quantified using a probabilistic uniform quantizer. Secondly, the random variables obeying Bernoulli distribution are used to express whether the attack occurs or not, and the measurement equations of the system are reconstructed. Then, the upper bound of the estimated error covariance is derived by using the quantization error and the norm boundedness of the attack vector and minimising the upper bound of the estimated error covariance at each moment to obtain the optimal filtering gain. Finally, simulations based on the IEEE-69 distribution network test system were carried out to verify that the improved unscented Kalman filter algorithm can effectively ensure the estimation performance of the system against the quantization effect and false data injection attacks.
更多查看译文
关键词
quantization effects,false data injection attacks,distribution network,unscented Kalman filtering
AI 理解论文
溯源树
样例
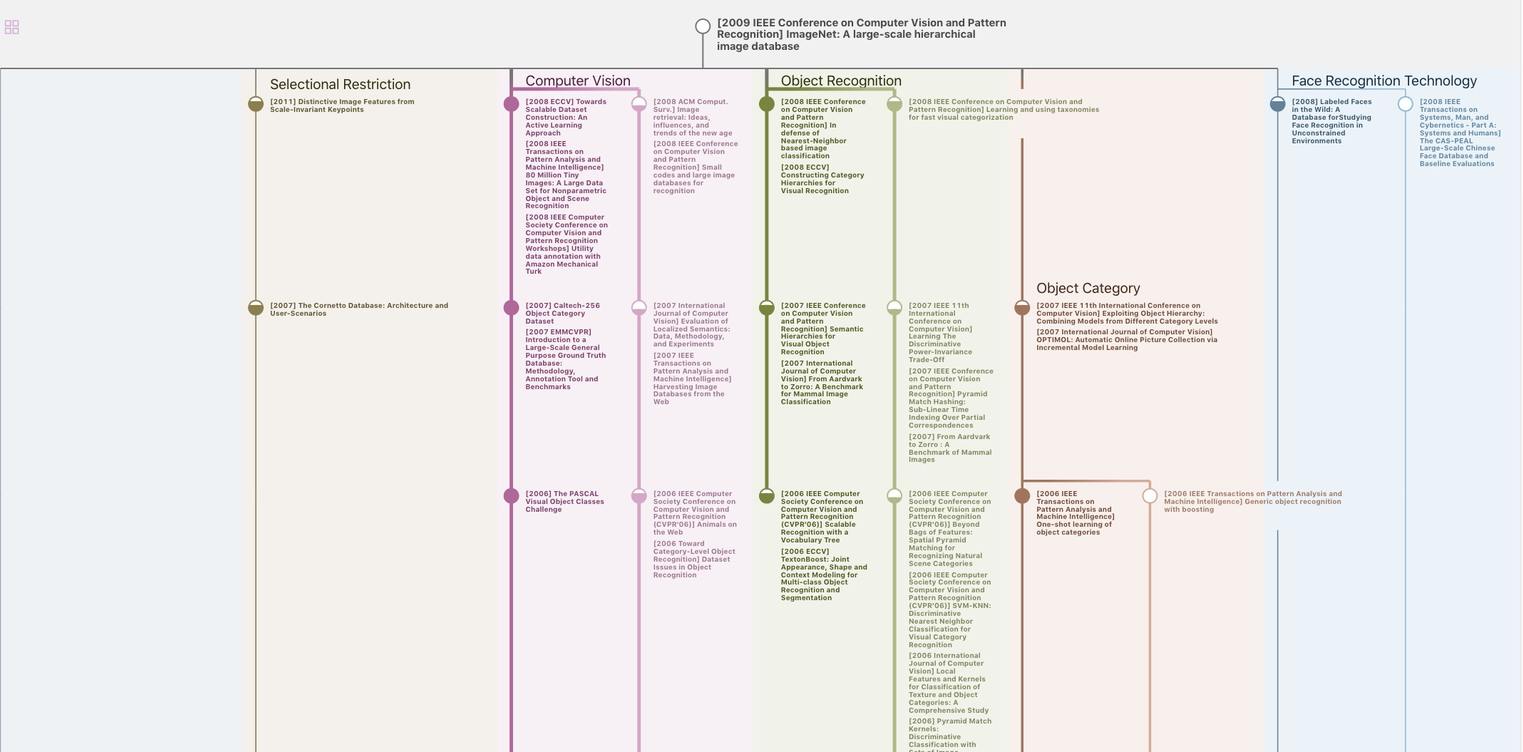
生成溯源树,研究论文发展脉络
Chat Paper
正在生成论文摘要