Synthesizing Traffic Datasets using Graph Neural Networks
CoRR(2023)
摘要
Traffic congestion in urban areas presents significant challenges, and
Intelligent Transportation Systems (ITS) have sought to address these via
automated and adaptive controls. However, these systems often struggle to
transfer simulated experiences to real-world scenarios. This paper introduces a
novel methodology for bridging this `sim-real' gap by creating photorealistic
images from 2D traffic simulations and recorded junction footage. We propose a
novel image generation approach, integrating a Conditional Generative
Adversarial Network with a Graph Neural Network (GNN) to facilitate the
creation of realistic urban traffic images. We harness GNNs' ability to process
information at different levels of abstraction alongside segmented images for
preserving locality data. The presented architecture leverages the power of
SPADE and Graph ATtention (GAT) network models to create images based on
simulated traffic scenarios. These images are conditioned by factors such as
entity positions, colors, and time of day. The uniqueness of our approach lies
in its ability to effectively translate structured and human-readable
conditions, encoded as graphs, into realistic images. This advancement
contributes to applications requiring rich traffic image datasets, from data
augmentation to urban traffic solutions. We further provide an application to
test the model's capabilities, including generating images with manually
defined positions for various entities.
更多查看译文
关键词
Graph Neural Networks,Time Of Day,Image Segmentation,Data Augmentation,Generative Adversarial Networks,Adaptive Control,Image Generation,Traffic Congestion,Realistic Images,Intelligent Transportation Systems,Graph Attention,Different Levels Of Abstraction,Traffic Simulation,Graph Attention Network,Convolutional Neural Network,Image Regions,Pedestrian,Graphical User Interface,Bounding Box,Nodes In The Graph,Segmentation Map,Fréchet Inception Distance,OpenStreetMap Data,Traffic Control,Latent Image,Variational Autoencoder,Deep Reinforcement Learning,Pixel Accuracy,Input Graph,Transposed Convolution Layers
AI 理解论文
溯源树
样例
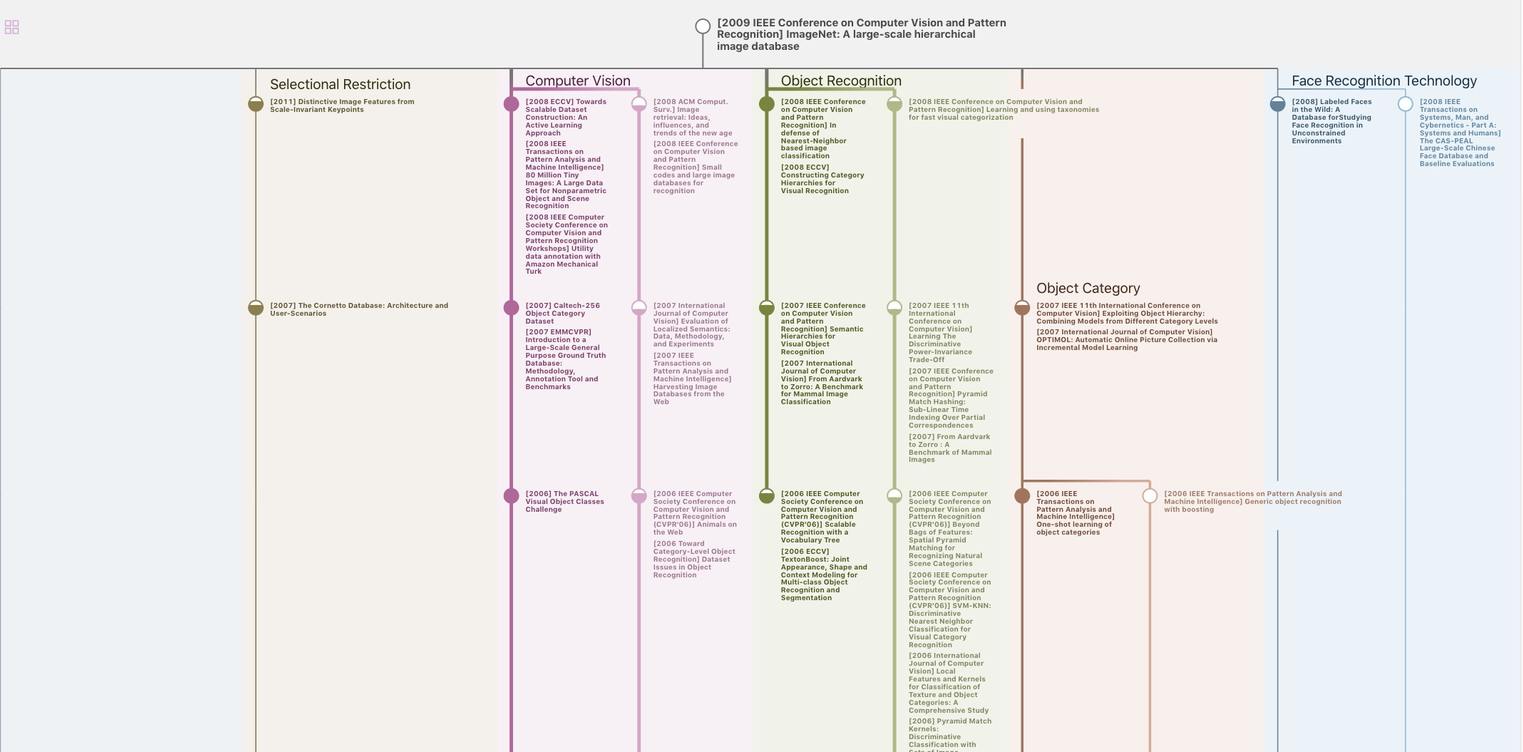
生成溯源树,研究论文发展脉络
Chat Paper
正在生成论文摘要