Discriminative Reward Co-Training
crossref(2023)
摘要
Abstract We propose discriminative reward co-training (DIRECT) as an extension to deep reinforcement learning algorithms. Building upon the concept of self-imitation learning (SIL), we introduce an imitation buffer to store beneficial trajectories generated by the policy, determined by their return. A discriminator network is trained concurrently to the policy to distinguish between trajectories generated by the current policy and beneficial trajectories generated by previous policies. The discriminator’s verdict is used to construct a reward signal for optimizing the policy. By interpolating prior experience, DIRECT is able to act as a reward surrogate, steering policy optimization towards more valuable regions of the reward landscape, thus, towards learning an optimal policy. In this article we formally introduce the additional components, their intended purpose and parameterization, and define a unified training procedure. To reveal insights into the mechanics of the proposed architecture, we provide evaluations of the introduced hyperparameters. Further benchmark evaluations in various discrete and continuous control environments provide evidence that DIRECT is especially beneficial in environments possessing sparse rewards, hard exploration tasks, and shifting circumstances. Our results show that DIRECT outperforms state-of-the-art algorithms in those challenging scenarios by providing a surrogate reward to the policy and directing the optimization towards valuable areas.
更多查看译文
AI 理解论文
溯源树
样例
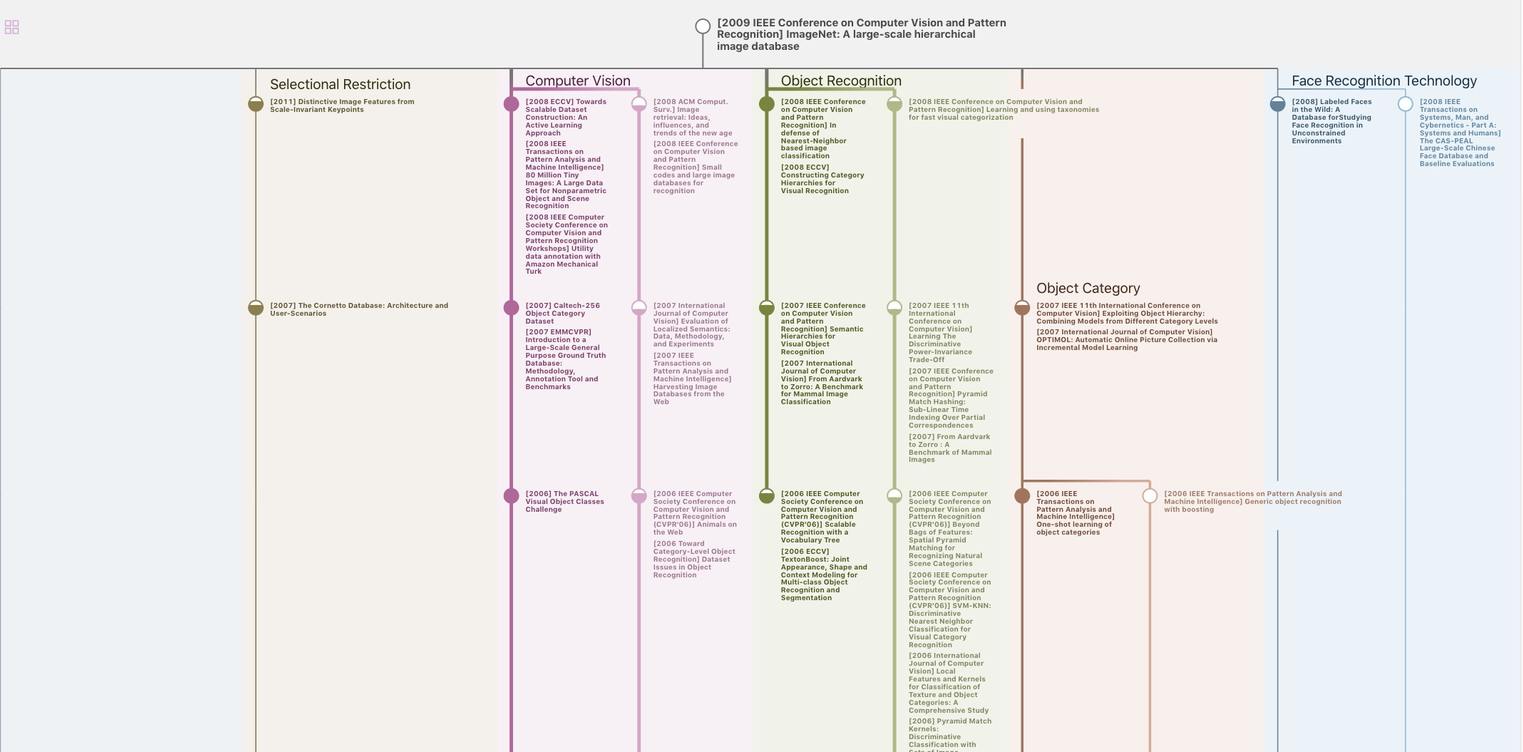
生成溯源树,研究论文发展脉络
Chat Paper
正在生成论文摘要